Comparison of Preprocessors for Machine Learning in the Predictive Maintenance Domain
2020 IEEE 29th International Symposium on Industrial Electronics (ISIE)(2020)
摘要
The optimization of maintenance schedules by predicting machine-health-information is a very obvious application domain for Machine Learning techniques. As part of an ongoing research project, we investigate the ability of Machine Learning to contribute to error prediction in noisy measurements using various preprocessing methods. We focus our efforts on drift-based error patterns and chose several well-established approaches from quality-assurance literature as potential preprocessors for three different Machine Learning methods. We compare the performance of these methods in predicting machine failures, using data based on realistic measurements taken from a supply line in an automotive factory. We further expose the tested methods to different levels of noise and evaluate the performance in detail for drift patterns of varying severity.
更多查看译文
关键词
machine learning,predictive maintenance,preprocessing,drift detection,accuracy,neural network,long-short-term-memory
AI 理解论文
溯源树
样例
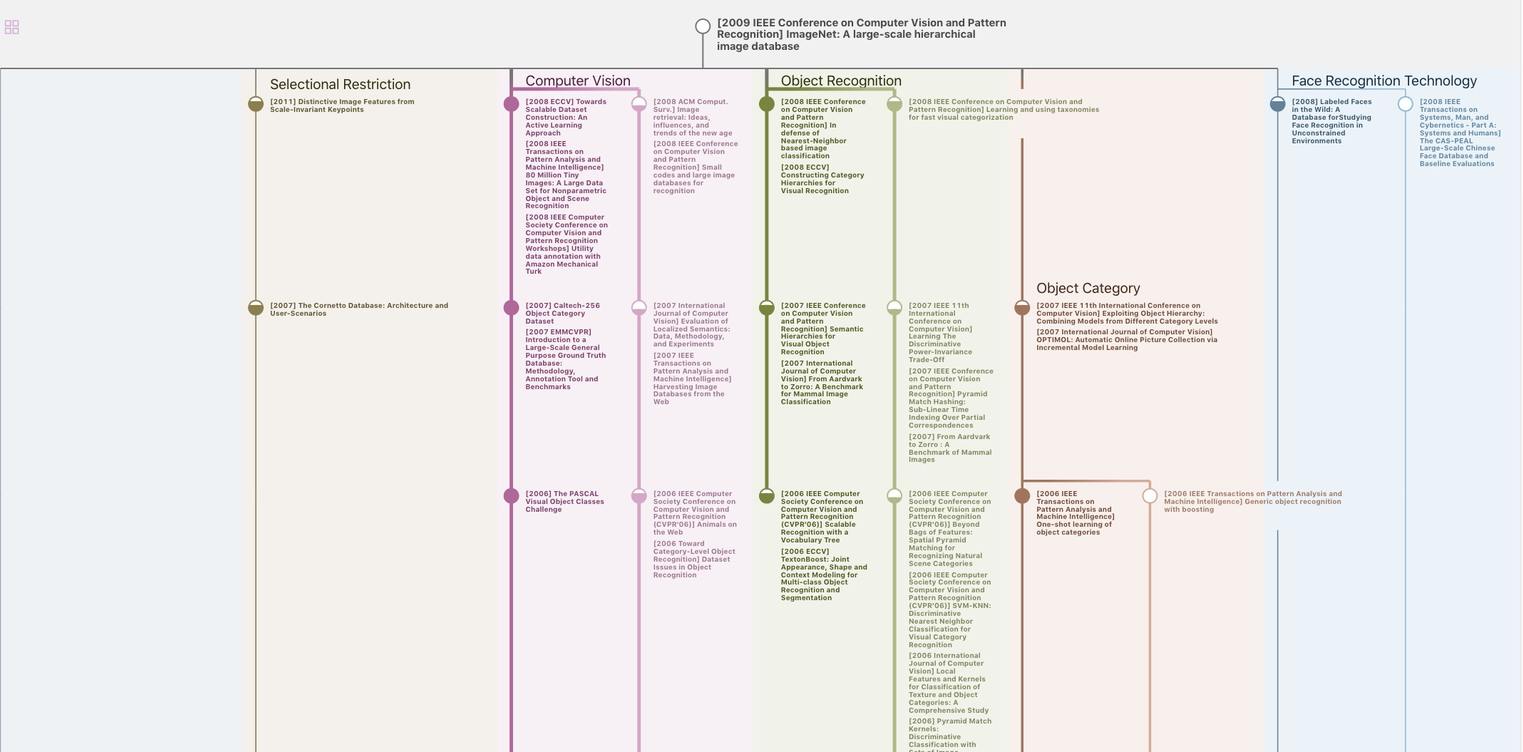
生成溯源树,研究论文发展脉络
Chat Paper
正在生成论文摘要