Csrgan: Medical Image Super-Resolution Using A Generative Adversarial Network
2020 IEEE 17th International Symposium on Biomedical Imaging Workshops (ISBI Workshops)(2020)
摘要
Super-resolution medical image is vital for doctor’s diagnosis and quantitative analysis. In this work we propose a novel super-resolution generative adversarial network which combine conditional GAN (CGAN) and SRGAN, refer to it as CSRGAN to generate super-resolution (SR) images. We use differential geometric information including Jacobian determinant (JD) and curl vector (CV) as conditional inputs of both the discriminator and generator of SRGAN, which make full use of the idea of using GANs to learn a mapping from one manifold to another. In addition, we proposed a content loss motivated by CV feature information instead of VGG loss in SRGAN. We trained our model on a large-scale dataset CelebFaces Attributes, tested it on medical ultrasound image dataset. The experimental results show the method can achieve better performance in SR image generation with higher average peak signal-tonoise ratio (PSNR), Structural Similarity (SSIM) and Mean Opinion Score (MOS) compared with SRGAN.
更多查看译文
关键词
Super-resolution,Differential geometric information,CSRGAN,Manifold
AI 理解论文
溯源树
样例
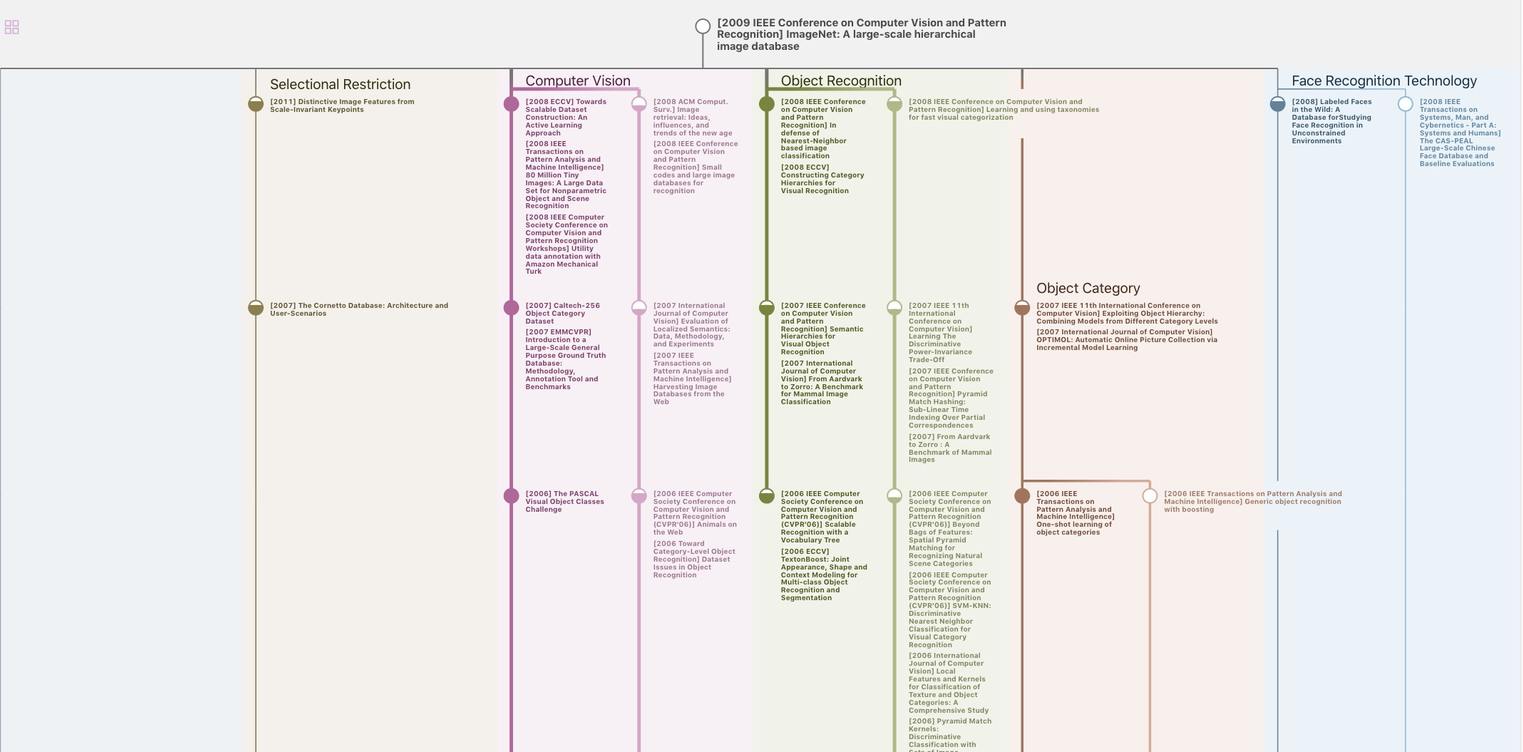
生成溯源树,研究论文发展脉络
Chat Paper
正在生成论文摘要