Information Extraction from Document Images via FCA based Template Detection and Knowledge Graph Rule Induction.
CVPR Workshops(2020)
摘要
We view information extraction from document images as a complex problem that requires a combination of 1) state of the art deep learning vision models for detection of entities and primitive relations, 2) symbolic background knowledge that expresses prior information of spatial and semantic relationships, using the entities and primitive relations from the neural detectors, and 3) learning of symbolic extraction rules using one, or few examples of annotated document images. Several challenges arise in ensuring that this neuro-symbolic software stack works together seamlessly. These include vision-based challenges to ensure that the documents are "seen" at the appropriate level of detail to detect entities; symbolic representation challenges in identifying primitive relations between the entities identified by the vision system; learning-based challenges of identifying the appropriate level of symbolic abstraction for the retrieval rules, the need to identify background knowledge that is relevant to the documents being analyzed, and learning general symbolic rules in data-deficient domains. In this paper, we describe how we meet some of these challenges in the design of our document-reading platform. In particular, we focus on use cases with multiple templates which additionally involves finding structurally similar images in large heterogeneous document image collections. An adaptive lattice based template allocation module was utilized for evaluating document similarity based on both textual content and document structure. A knowledge graph is used for capturing document structure and a relational rule learning system is employed on the knowledge graph for generating extraction rules. Experiments on a publicly shared data-set 1 of 1400 trade finance documents demonstrates the viability of the proposed system.
更多查看译文
关键词
information extraction,FCA based template detection,knowledge graph rule induction,complex problem,vision models,primitive relations,spatial relationships,semantic relationships,symbolic extraction rules,annotated document images,neuro-symbolic software stack works,vision-based challenges,symbolic representation challenges,learning-based challenges,symbolic abstraction,retrieval rules,background knowledge,general symbolic rules,document-reading platform,multiple templates,structurally similar images,heterogeneous document image collections,template allocation module,document similarity,document structure,relational rule learning system,trade finance documents
AI 理解论文
溯源树
样例
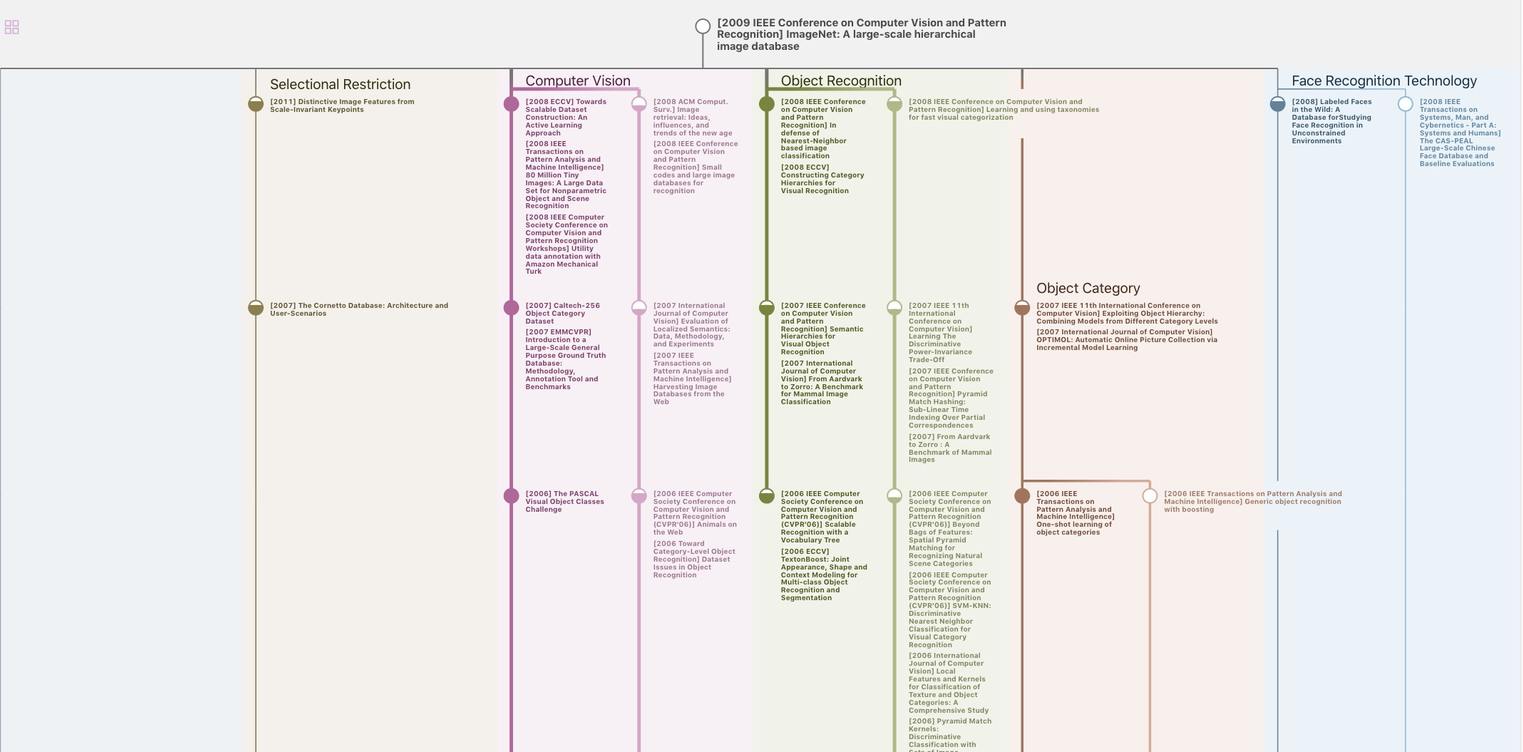
生成溯源树,研究论文发展脉络
Chat Paper
正在生成论文摘要