HIDeGan - A Hyperspectral-guided Image Dehazing GAN.
CVPR Workshops(2020)
摘要
Haze removal in images captured from a diverse set of scenarios is a very challenging problem. The existing dehazing methods either reconstruct the transmission map or directly estimate the dehazed image in RGB color space. In this paper, we make a first attempt to propose a Hyperspectral-guided Image Dehazing Generative Adversarial Network (HIDEGAN). The HIDEGAN architecture is formulated by designing an enhanced version of CYCLEGAN named R2HCYCLE and an enhanced conditional GAN named H2RGAN. The R2HCYCLE makes use of the hyperspectral-image (HSI) in combination with cycle-consistency and skeleton losses in order to improve the quality of information recovery by analyzing the entire spectrum. The H2RGAN estimates the clean RGB image from the hazy hyperspectral image generated by the R2HCYCLE. The models designed for spatial-spectral-spatial mapping generate visually better haze-free images. To facilitate HSI generation, datasets from spectral reconstruction challenge at NTIRE 2018 and NTIRE 2020 are used. A comprehensive set of experiments were conducted on the D-Hazy,and the recent RESIDE-Standard (SOTS), RESIDE-beta (OTS) and RESIDE-Standard (HSTS) datasets. The proposed HIDEGAN outperforms the existing state-of-the-art in all these datasets.
更多查看译文
关键词
hyperspectral-guided image dehazing generative adversarial network,hyperspectral-guided image dehazing GAN,spectral reconstruction challenge,HSI generation,haze-free images,spatial-spectral-spatial mapping,hazy hyperspectral image,clean RGB image,hyperspectral-image,H2RGAN,enhanced conditional GAN,R2HCYCLE,HIDEGAN architecture,RGB color space,dehazed image,transmission map,dehazing methods,haze removal
AI 理解论文
溯源树
样例
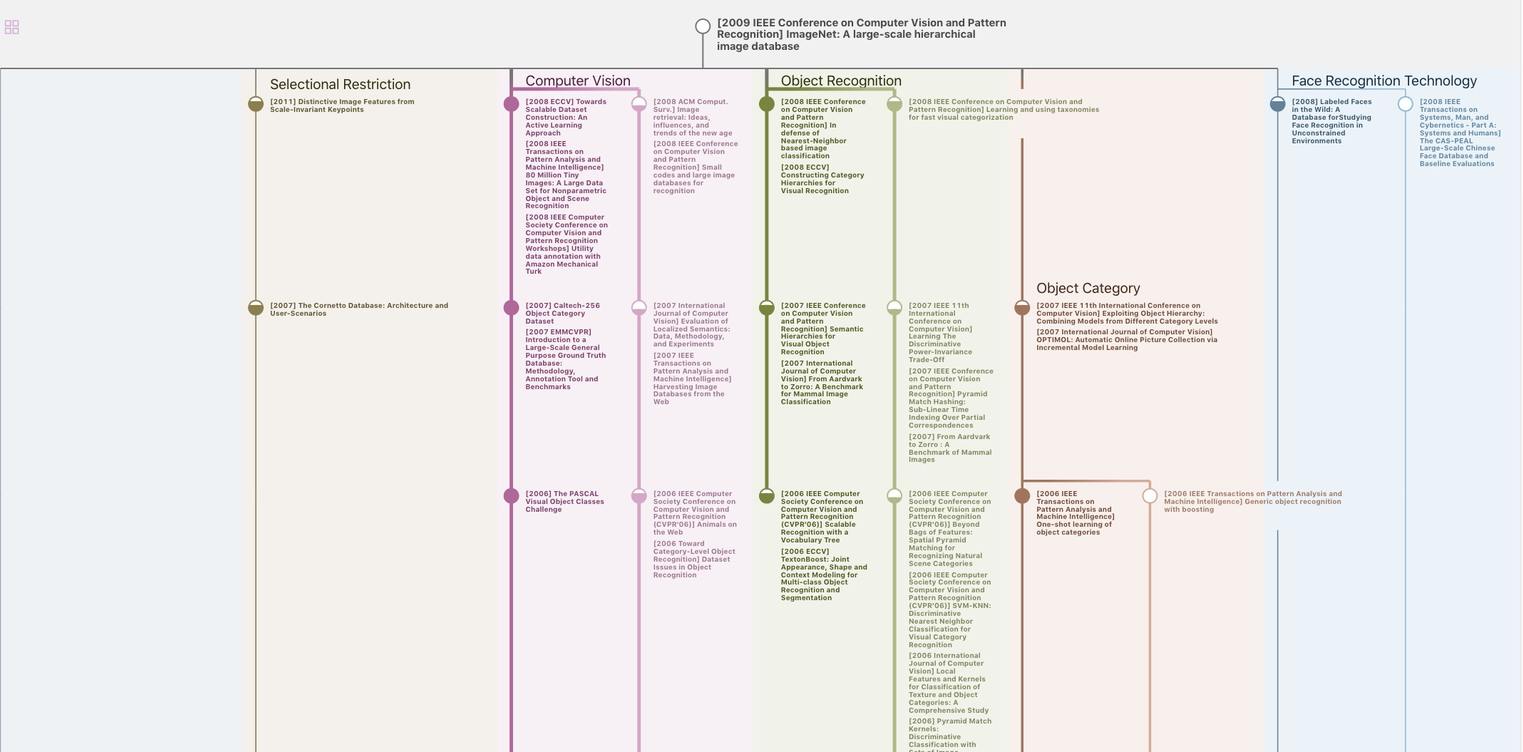
生成溯源树,研究论文发展脉络
Chat Paper
正在生成论文摘要