Towards Fine-grained Sampling for Active Learning in Object Detection.
CVPR Workshops(2020)
摘要
We study the problem of using active learning to reduce annotation effort in training object detectors. Existing efforts in this space ignore the fact that image annotation costs are variable, depending on the number of objects present in a single image. In this regard, we examine a fine-grained sampling based approach for active learning in object detection. Over an unlabeled pool of images, our method aims to selectively pick the most informative subset of bounding boxes (as opposed to full images) to query an annotator. We measure annotation efforts in terms of the number of ground truth bounding boxes obtained. We study the effects of our method on the Feature Pyramid Network and RetinaNet models, and show promising savings in labeling effort to obtain good detection performance.
更多查看译文
关键词
single image,fine-grained sampling based approach,active learning,object detection,labeling effort,object detectors,image annotation costs,feature pyramid network,RetinaNet models,ground truth bounding boxes
AI 理解论文
溯源树
样例
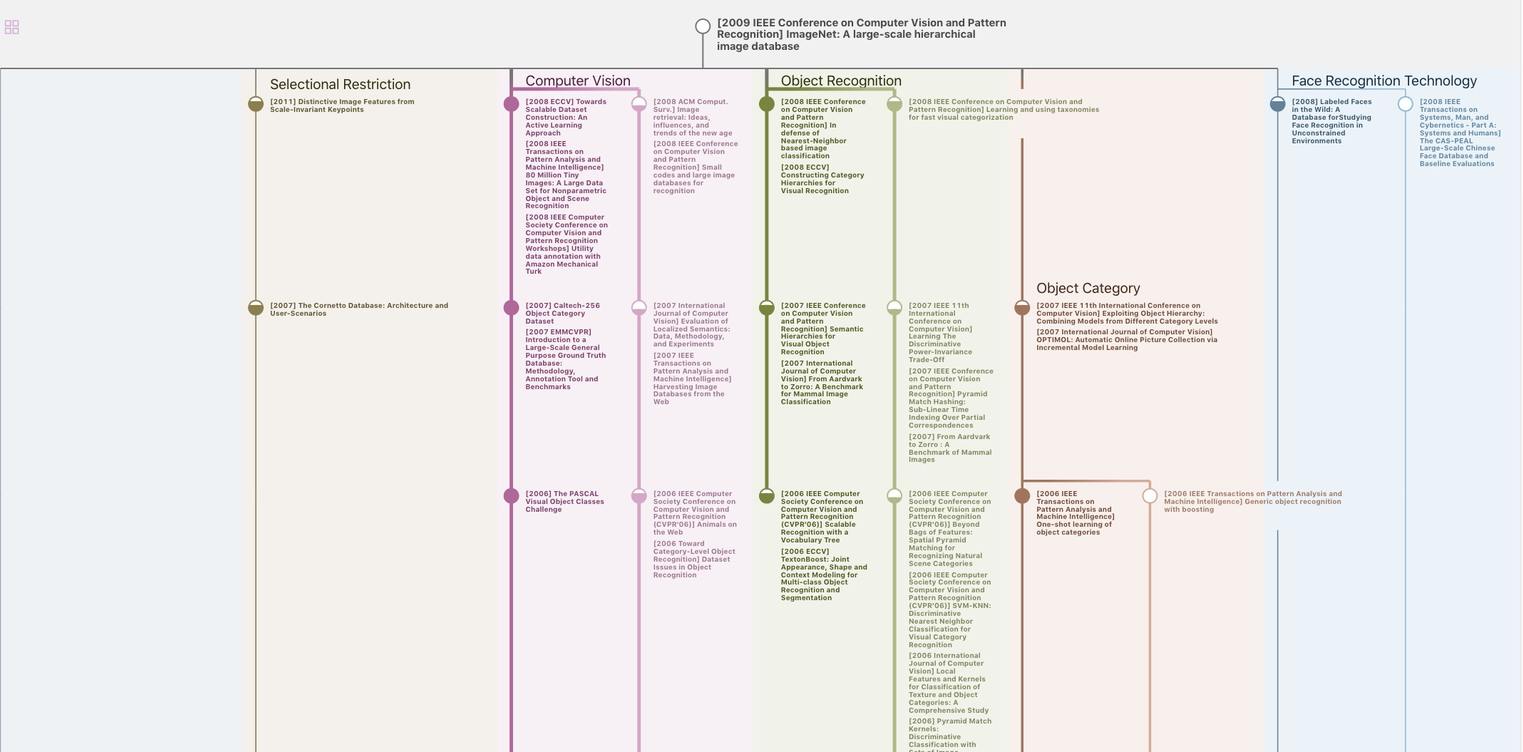
生成溯源树,研究论文发展脉络
Chat Paper
正在生成论文摘要