Squeeze U-Net - A Memory and Energy Efficient Image Segmentation Network.
CVPR Workshops(2020)
摘要
To facilitate implementation of deep neural networks on embedded systems keeping memory and computation requirements low is critical, particularly for real-time mobile use. In this work, we propose a SqueezeNet inspired version of U-Net for image segmentation that achieves a 12X reduction in model size to 32MB, and 3.2X reduction in Multiplication Accumulation operations (MACs) from 287 billion ops to 88 billion ops for inference on the CamVid data set while preserving accuracy. Our proposed Squeeze U-Net is efficient in both low MACs and memory use. Our performance results using Tensorflow 1.14 with Python 3.6 and CUDA 10.1.243 on an NVIDIA K40 GPU shows that Squeeze U-Net is 17% faster for inference and 52% faster for training than U-Net for the same accuracy.
更多查看译文
关键词
low MACs,Squeeze U-Net,deep neural networks,embedded systems,computation requirements,SqueezeNet inspired version,Multiplication Accumulation operations,NVIDIA K40 GPU,energy efficient image segmentation network,memory requirements,CamVid data set,Tensorflow 1.14,Python 3.6,CUDA 10.1.243,memory size 32.0 MByte
AI 理解论文
溯源树
样例
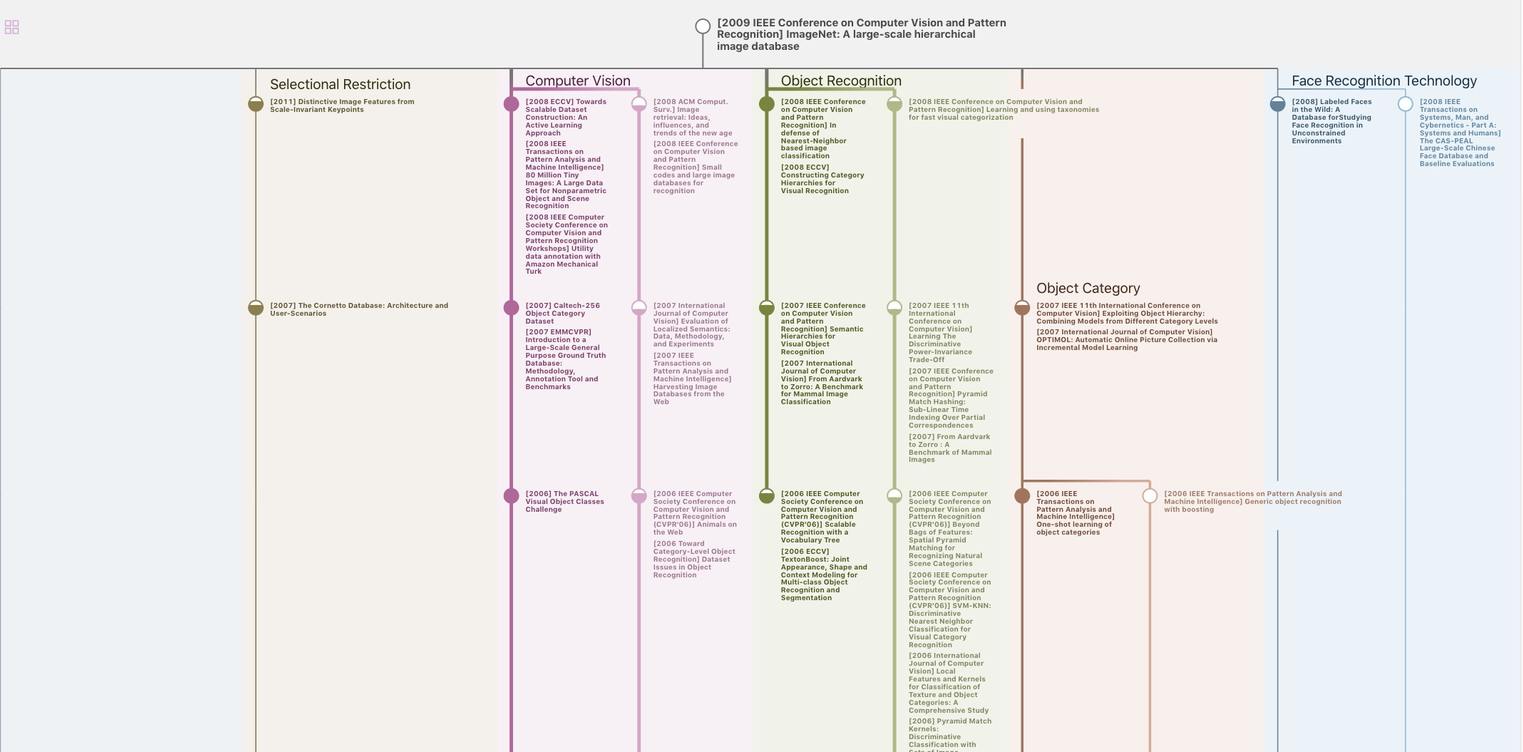
生成溯源树,研究论文发展脉络
Chat Paper
正在生成论文摘要