CRSSC: Salvage Reusable Samples from Noisy Data for Robust Learning
arxiv(2020)
摘要
Due to the existence of label noise in web images and the high memorization capacity of deep neural networks, training deep fine-grained (FG) models directly through web images tends to have an inferior recognition ability. In the literature, to alleviate this issue, loss correction methods try to estimate the noise transition matrix, but the inevitable false correction would cause severe accumulated errors. Sample selection methods identify clean ("easy") samples based on the fact that small losses can alleviate the accumulated errors. However, "hard" and mislabeled examples that can both boost the robustness of FG models are also dropped. To this end, we propose a certainty-based reusable sample selection and correction approach, termed as CRSSC, for coping with label noise in training deep FG models with web images. Our key idea is to additionally identify and correct reusable samples, and then leverage them together with clean examples to update the networks. We demonstrate the superiority of the proposed approach from both theoretical and experimental perspectives. The source code, models, and data have been made available at https://github.com/NUSTMachine-Intelligence-Laboratory/CRSSC.
更多查看译文
关键词
label noise, noisy data, sample selection, robust learning
AI 理解论文
溯源树
样例
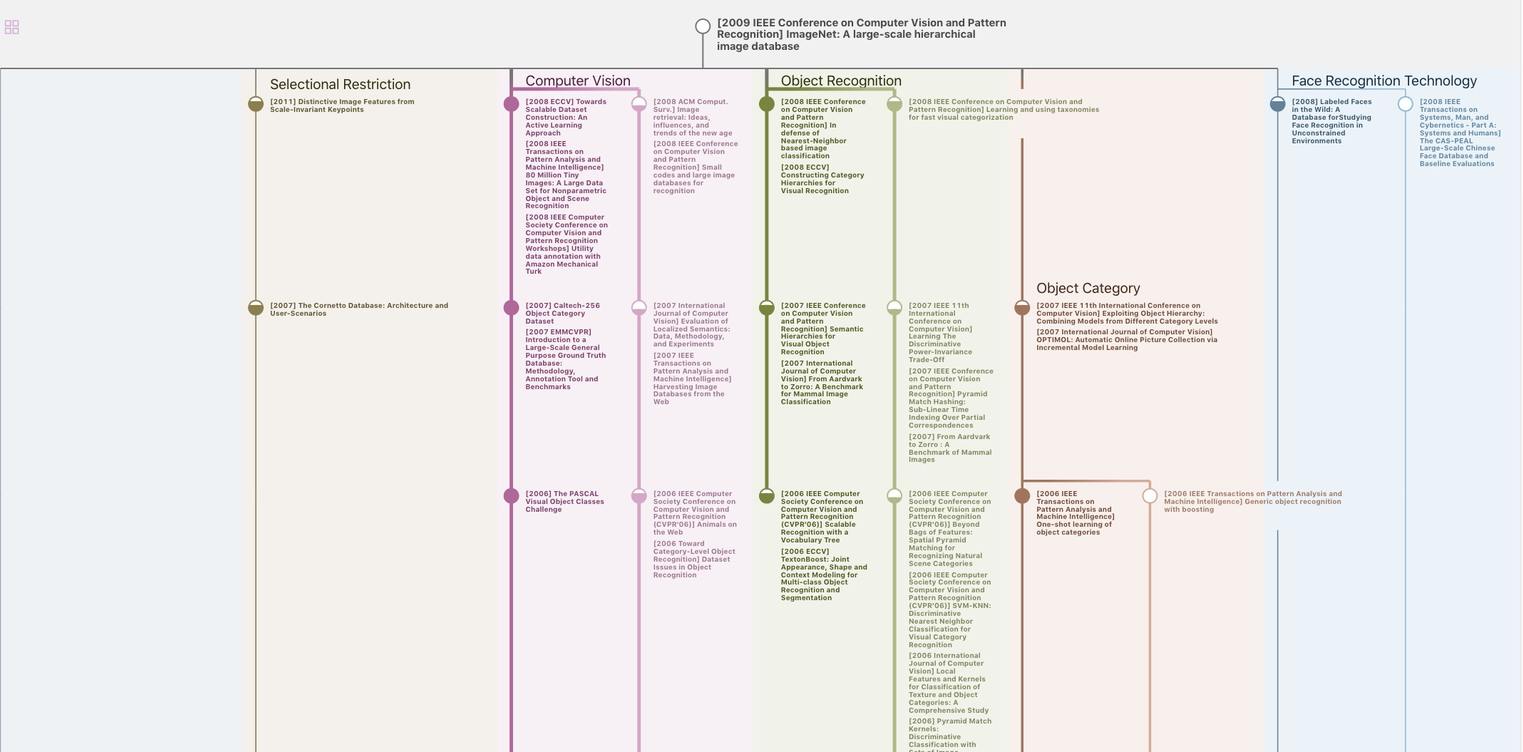
生成溯源树,研究论文发展脉络
Chat Paper
正在生成论文摘要