Tensor-Train-Based Algorithms For Aggregate State Estimation Of Swarms With Interacting Agents
2020 AMERICAN CONTROL CONFERENCE (ACC)(2020)
摘要
In this paper, we develop an efficient implementation of the gas-kinetic (GK) Probability Hypothesis Density (PHD) filter for aggregate swarm state estimation with interacting agents. We borrow a kinetic/mesoscopic partial differential equation (PDE) model of a swarm of interacting agents from biology moving in a plane with a heading state, which requires the computation of integrals up to five dimensions. In the context of the GK-PHD, we propagate this model by computing in a compressed format called the Tensor Train (TT) format, yielding better memory and runtime properties than a grid-based approach. Under certain assumptions, we prove that TT-GK-PHD has a time complexity of an order of magnitude better than the grid-based approach. Finally, we showcase the usefulness of our algorithm on a scenario which cannot be solved via the grid-based approach due to hardware memory constraints. Then in a computational experiment we demonstrate the better runtime and memory of TT-GK-PHD over the grid-based approach.
更多查看译文
关键词
grid-based approach,TT-GK-PHD,tensor-train-based algorithms,interacting agents,aggregate swarm state estimation,heading state,tensor train format,runtime properties,gas-kinetic probability hypothesis density filter,partial differential equation model,compressed format,time complexity,hardware memory constraints
AI 理解论文
溯源树
样例
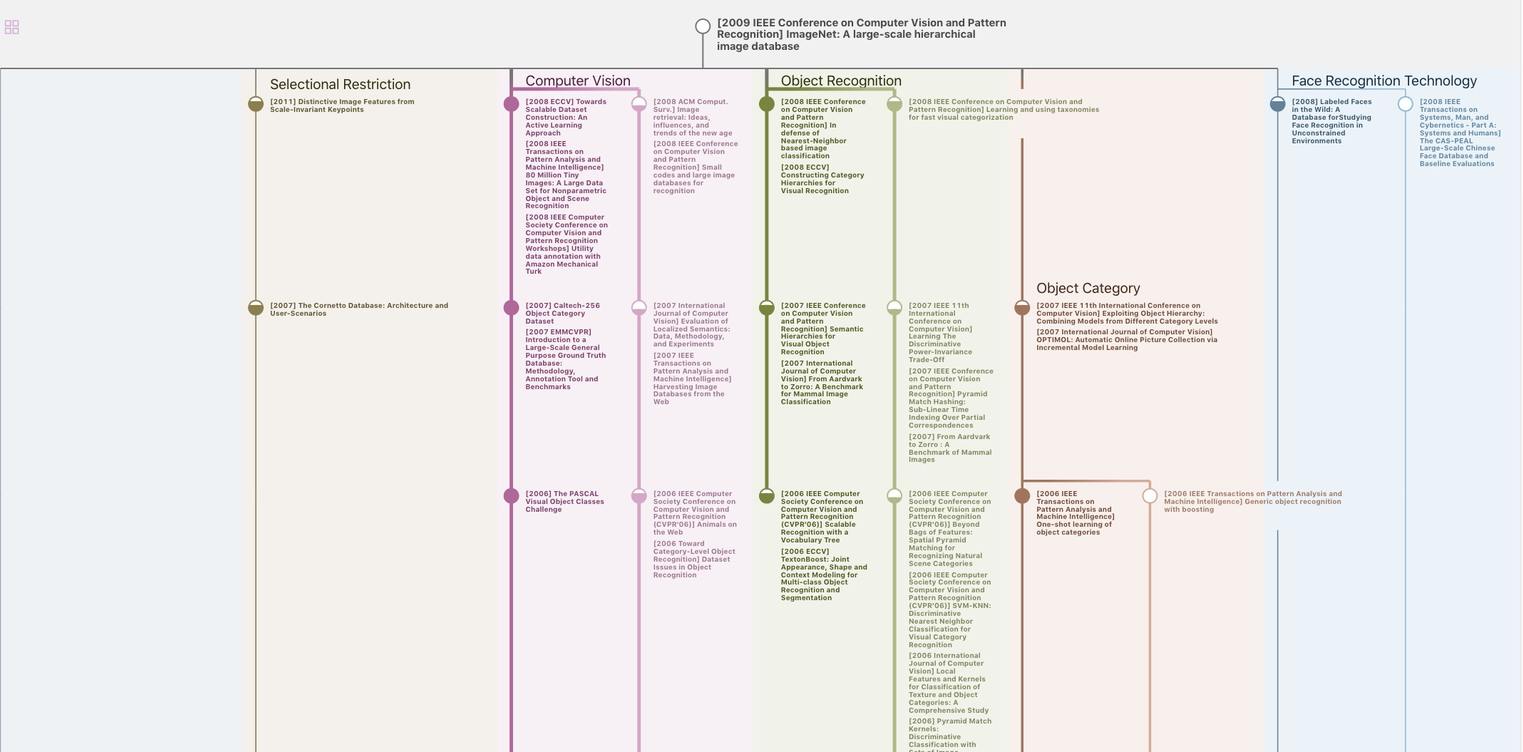
生成溯源树,研究论文发展脉络
Chat Paper
正在生成论文摘要