Distributionally Robust Surrogate Optimal Control For Large-Scale Dynamical Systems
2020 AMERICAN CONTROL CONFERENCE (ACC)(2020)
摘要
This paper explores tractable robust optimal control of nonlinear systems with large state spaces. Conventional applications of surrogate modeling for control replace the underlying dynamical model with a data-driven surrogate function. For large-scale systems, this approach possesses a host of shortcomings. We address these challenges by presenting a novel robust surrogate optimization framework for finite-time and receding horizon optimal control. Rather than modeling the entire state transition function, we define a surrogate model which maps the initial state and time series of control inputs to an approximate objective function value. We also define surrogate models which predict time series of relevant constraint functions. Since the bulk of the relevant information is encoded in the initial state, we apply a principal component analysis to project the state onto a reduced basis, allowing surrogate models with tractable parameterizations. To guarantee constraint satisfaction, we use phi-divergence to formulate distributionally robust chance constraints which are satisfied for worst-case realizations of the test data modeling error distribution. We validate our approach using a case study of optimal lithium-ion battery fast charging using a large-scale electrochemical battery model.
更多查看译文
关键词
principal component analysis,surrogate optimization framework,lithium-ion battery,electrochemical battery model,test data modeling error distribution,distributionally robust chance constraints,relevant constraint functions,approximate objective function value,state transition function,horizon optimal control,data-driven surrogate function,large-scale dynamical systems,distributionally robust surrogate optimal control
AI 理解论文
溯源树
样例
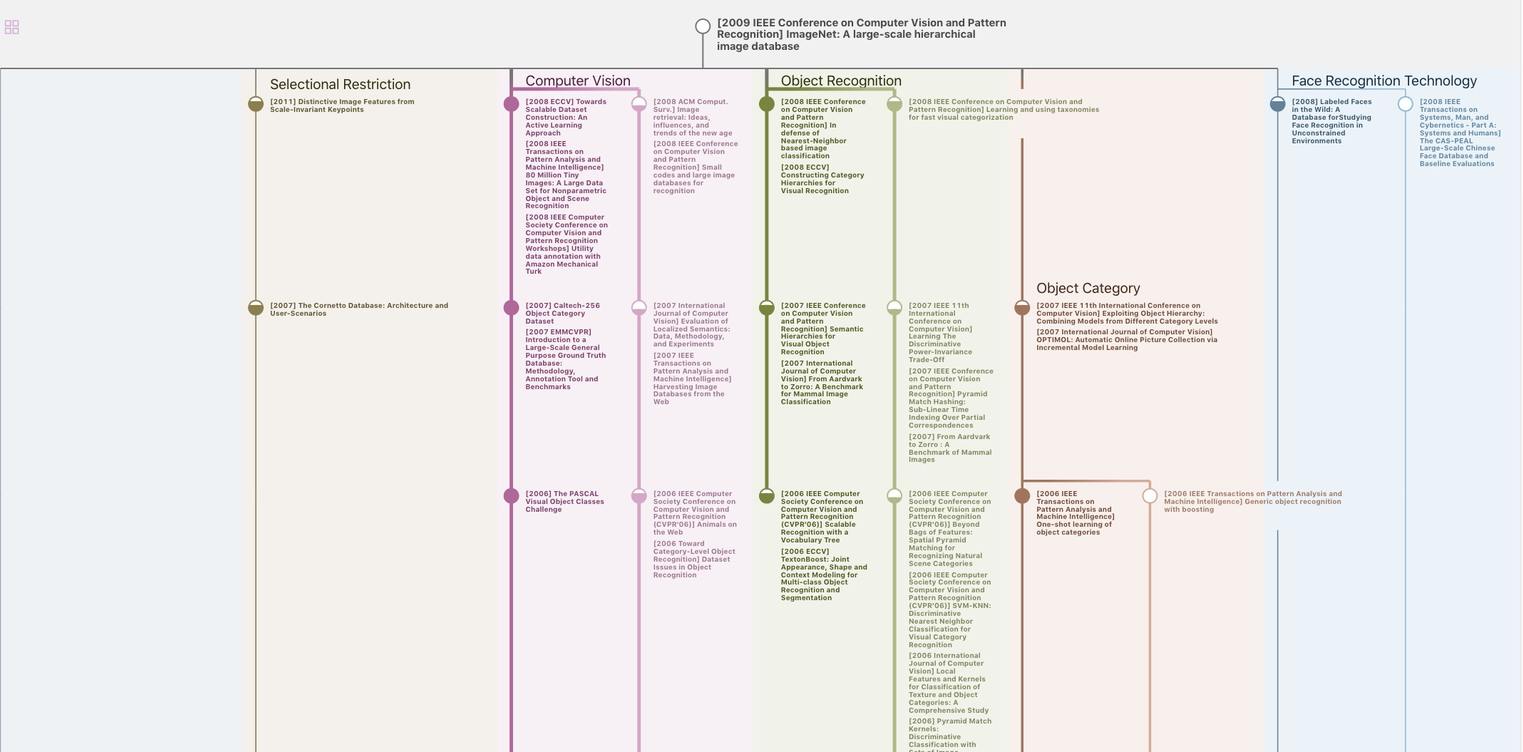
生成溯源树,研究论文发展脉络
Chat Paper
正在生成论文摘要