Scalable Coherence In Large Scale Second-Order Networks Using High-Gain Observer
2020 AMERICAN CONTROL CONFERENCE (ACC)(2020)
摘要
We propose a scalable second-order consensus algorithm where by tuning the controller parameter, the convergence rate of the consensus protocol is almost invariant with respect to the size of the network. This is beneficial when the algebraic connectivity of the graph Laplacian decreases towards zero, with an increase in the network size, which leads to degraded closed-loop performance. We realize the controller using a high-gain observer and it is shown that for sufficiently small observer parameter, the convergence rate under output feedback approaches the one under state feedback. We also study the controller performance under stochastic disturbances by first defining a performance output and then calculating the H-2 norm from the disturbance input to the performance output. We show that the H-2 norm for the state feedback controller is scalable as the network size increases. Moreover, we also show that for sufficiently small observer parameter, the H-2 norm under output feedback approaches the one under state feedback.
更多查看译文
关键词
state feedback controller,network size,observer parameter,output feedback,scalable coherence,high-gain observer,second-order consensus algorithm,controller parameter tuning,convergence rate,consensus protocol,algebraic connectivity,closed-loop performance,controller performance,graph Laplacian,large scale second-order networks,stochastic disturbances,ℋ2 norm,multiagent systems
AI 理解论文
溯源树
样例
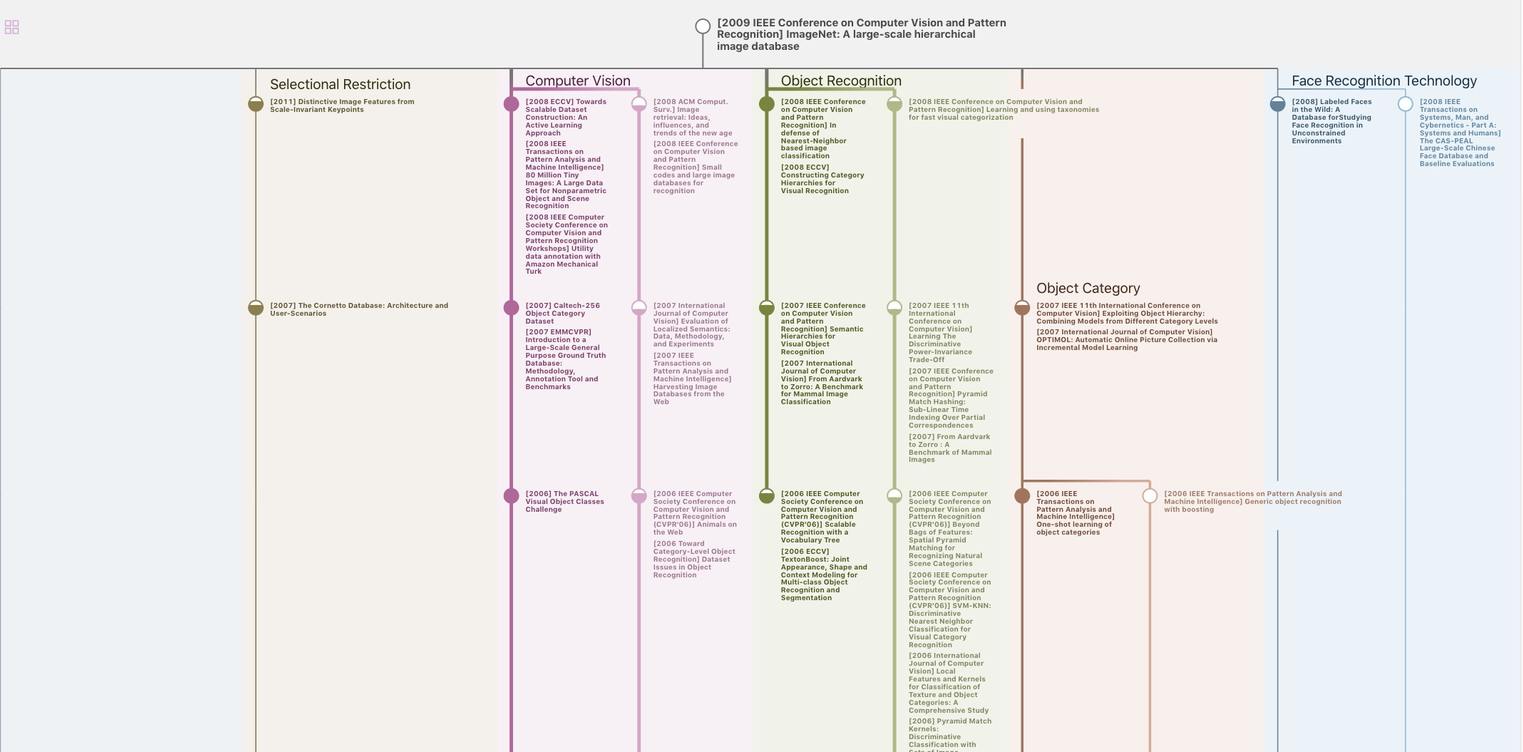
生成溯源树,研究论文发展脉络
Chat Paper
正在生成论文摘要