Learning Pose Estimation for UAV Autonomous Navigation and Landing Using Visual-Inertial Sensor Data
2020 AMERICAN CONTROL CONFERENCE (ACC)(2020)
摘要
In this work, we propose a robust network-in-the-loop control system for autonomous navigation and landing of an Unmanned-Aerial-Vehicle (UAV). To estimate the UAV's absolute pose, we develop a deep neural network (DNN) architecture for visual-inertial odometry, which provides a robust alternative to traditional methods. We first evaluate the accuracy of the estimation by comparing the prediction of our model to traditional visual-inertial approaches on the publicly available EuRoC MAV dataset. The results indicate a clear improvement in the accuracy of the pose estimation up to 25% over the baseline. Finally, we integrate the data-driven estimator in the closed-loop flight control system of Airsim, a simulator available as a plugin for Unreal Engine, and we provide simulation results for autonomous navigation and landing.
更多查看译文
关键词
UAV autonomous navigation,visual-inertial sensor data,robust network-in-the-loop control system,deep neural network architecture,visual-inertial odometry,EuRoC MAV dataset,pose estimation learning,data-driven estimator,UAV autonomous landing,Airsim closed-loop flight control system
AI 理解论文
溯源树
样例
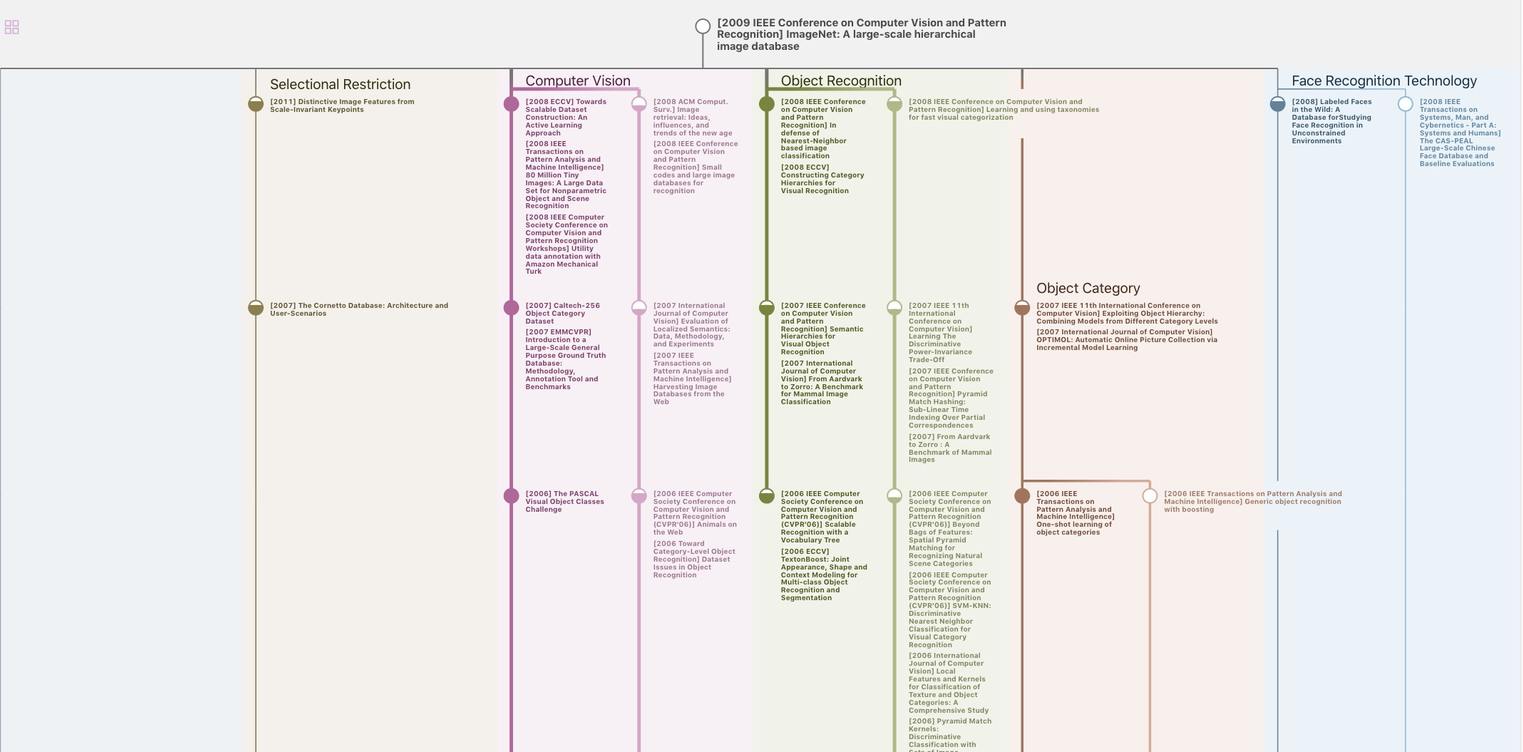
生成溯源树,研究论文发展脉络
Chat Paper
正在生成论文摘要