Regret Analysis For Learning In A Multi-Agent Linear-Quadratic Control Problem
2020 AMERICAN CONTROL CONFERENCE (ACC)(2020)
摘要
We consider a multi-agent Linear-Quadratic (LQ) reinforcement learning problem consisting of three systems, an unknown system and two known systems. In this problem, there are three agents - the actions of agent 1 can affect the unknown system as well as the two known systems while the actions of agents 2 and 3 can only affect their respective co-located known systems. Further, the unknown system's state can affect the known systems' state evolution. In this paper, we are interested in minimizing the infinite-horizon average cost. We propose a Thompson Sampling (TS)-based multi-agent learning algorithm where each agent learns the unknown system's dynamics independently. Our result indicates that the expected regret of our algorithm is upper bounded by (O) over tilde (root T) under certain assumptions, where (O) over tilde (center dot) hides constants and logarithmic factors. Numerical simulations are provided to illustrate the performance of our proposed algorithm.
更多查看译文
关键词
regret analysis,unknown system,Thompson sampling-based multiagent learning algorithm,multiagent linear-quadratic reinforcement learning problem,multiagent linear-quadratic control problem,known systems
AI 理解论文
溯源树
样例
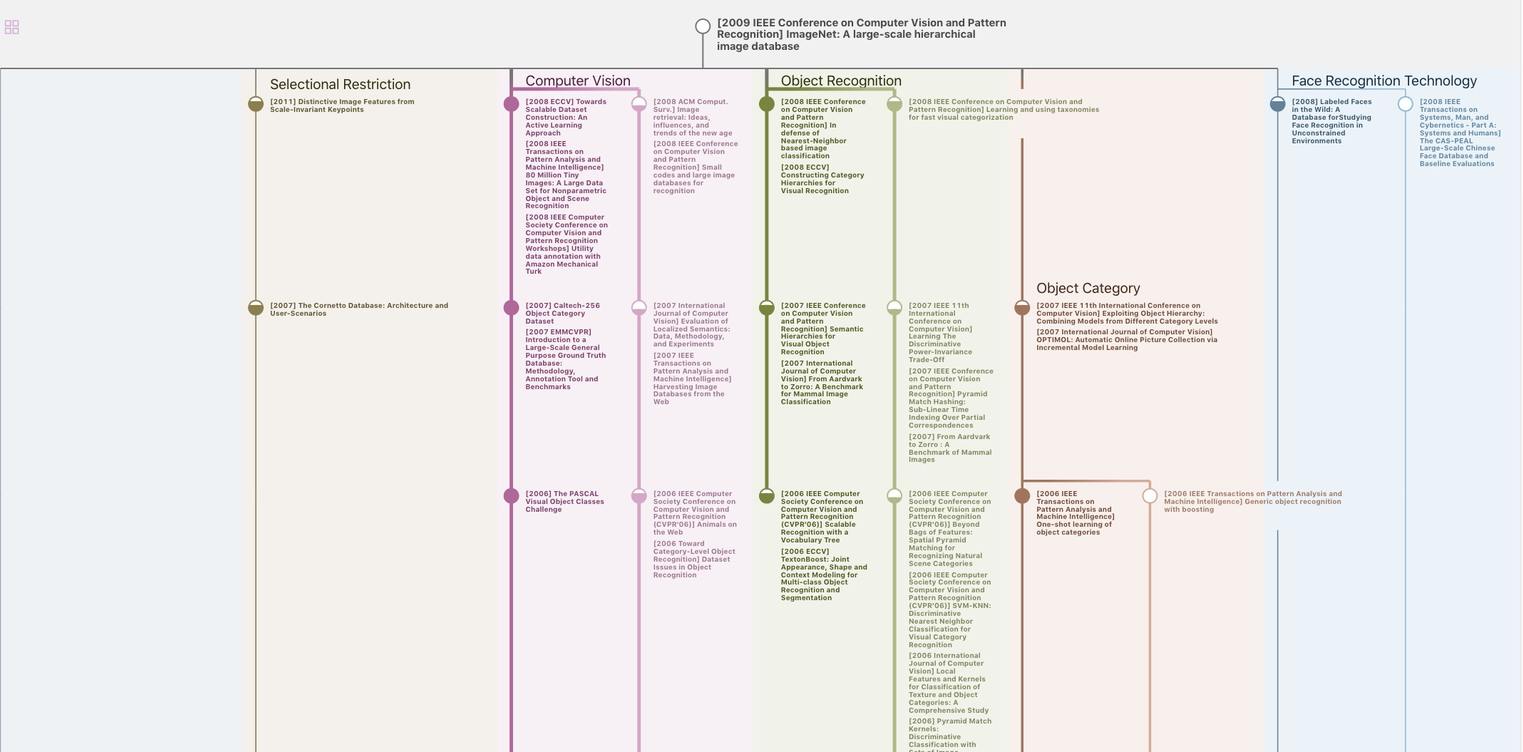
生成溯源树,研究论文发展脉络
Chat Paper
正在生成论文摘要