Training Neural Nets using only an Approximate Tableless LNS ALU
2020 IEEE 31st International Conference on Application-specific Systems, Architectures and Processors (ASAP)(2020)
摘要
The Logarithmic Number System (LNS) is useful in applications that tolerate approximate computation, such as classification using multi-layer neural networks that compute nonlinear functions of weighted sums of inputs from previous layers. Supervised learning has two phases: training (find appropriate weights for the desired classification), and inference (use the weights with approximate sum of products). Several researchers have observed that LNS ALUs in inference may minimize area and power by being both low-precision and approximate (allowing low-cost, tableless implementations). However, the few works that have also trained with LNS report at least part of the system needs accurate LNS. This paper describes a novel approximate LNS ALU implemented simply as logic (without tables) that enables the entire back-propagation training to occur in LNS, at one-third the cost of fixed-point implementation.
更多查看译文
关键词
approximate computation,logarithmic arithmetic,deep learning,neural networks,back-propagation
AI 理解论文
溯源树
样例
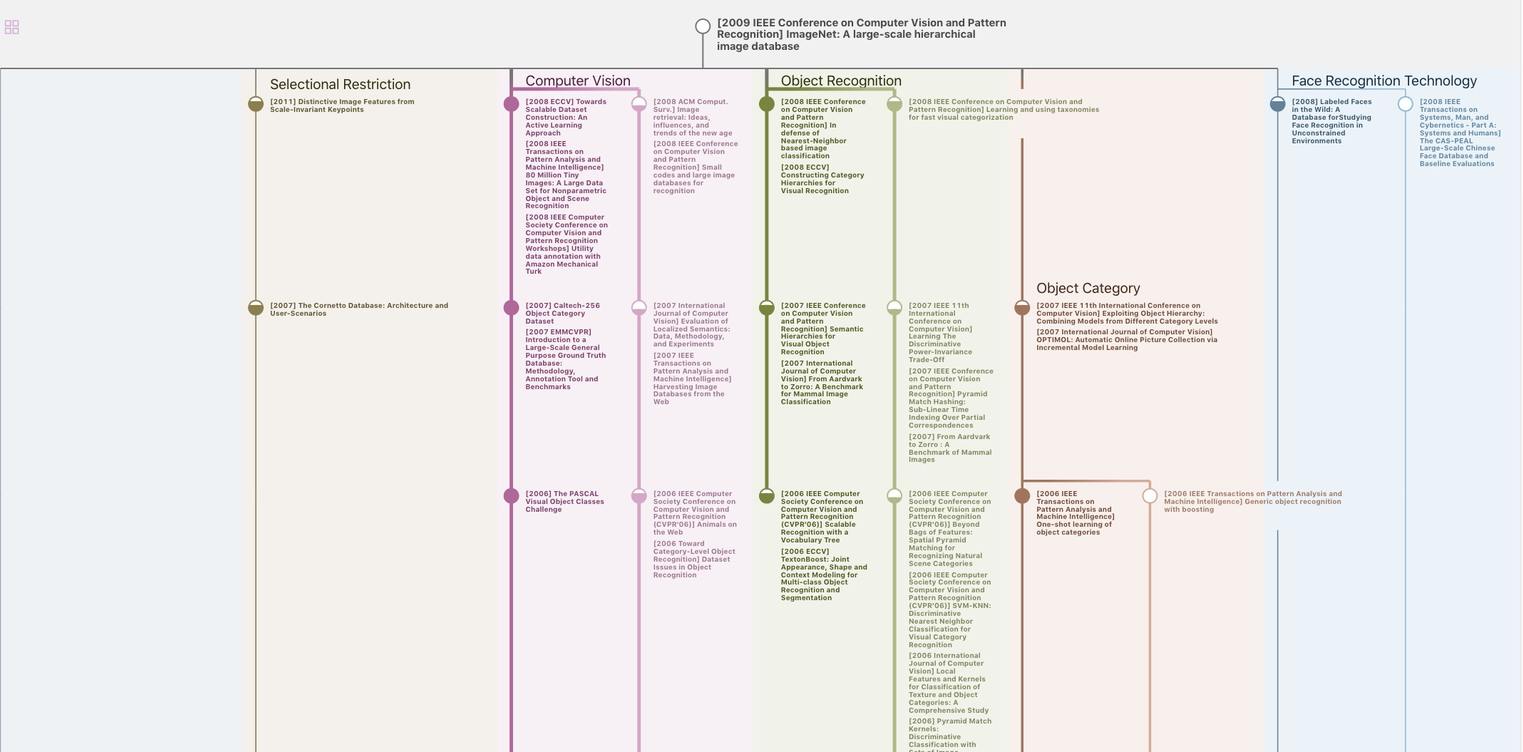
生成溯源树,研究论文发展脉络
Chat Paper
正在生成论文摘要