Extracting and Leveraging Nodule Features with Lung Inpainting for Local Feature Augmentation
MLMI@MICCAI(2020)
摘要
Chest X-ray (CXR) is the most common examination for fast detection of pulmonary abnormalities. Recently, automated algorithms have been developed to classify multiple diseases and abnormalities in CXR scans. However, because of the limited availability of scans containing nodules and the subtle properties of nodules in CXRs, state-of-the-art methods do not perform well on nodule classification. To create additional data for the training process, standard augmentation techniques are applied. However, the variance introduced by these methods are limited as the images are typically modified globally. In this paper, we propose a method for local feature augmentation by extracting local nodule features using a generative inpainting network. The network is applied to generate realistic, healthy tissue and structures in patches containing nodules. The nodules are entirely removed in the inpainted representation. The extraction of the nodule features is processed by subtraction of the inpainted patch from the nodule patch. With arbitrary displacement of the extracted nodules in the lung area across different CXR scans and further local modifications during training, we significantly increase the nodule classification performance and outperform state-of-the-art augmentation methods.
更多查看译文
关键词
Nodule classification, Local feature augmentation, Context encoder, Chest X-ray
AI 理解论文
溯源树
样例
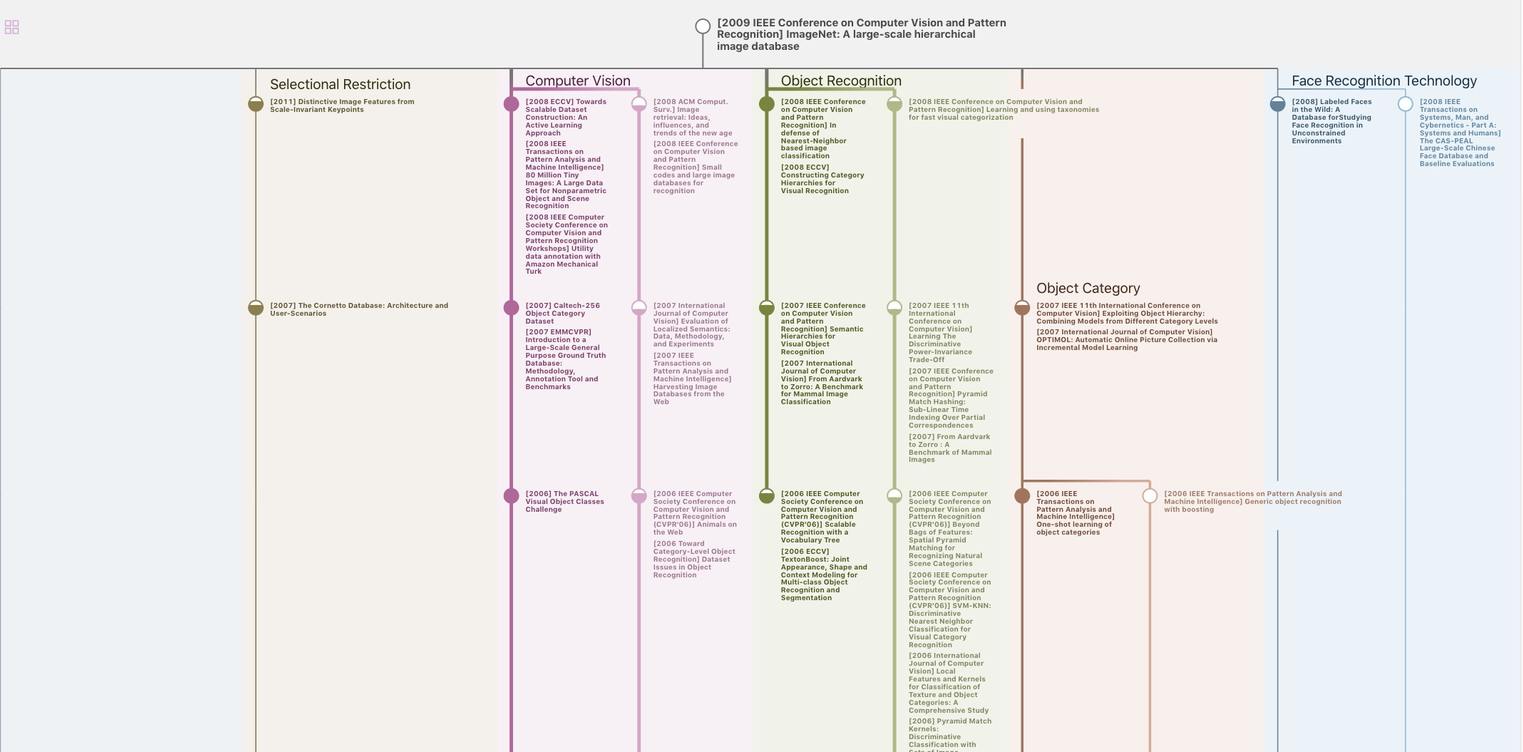
生成溯源树,研究论文发展脉络
Chat Paper
正在生成论文摘要