A feature-supervised generative adversarial network for environmental monitoring during hazy days
SCIENCE OF THE TOTAL ENVIRONMENT(2020)
摘要
The adverse hazeweather condition has brought considerable difficulties in vision-based environmental applications. While, until now, most of the existing environmental monitoring studies are under ordinary conditions, and the studies of complex haze weather conditions have been ignored. Thence, this paper proposes a feature-supervised learning network based on generative adversarial networks (GAN) for environmental monitoring during hazy days. Its main idea is to train the model under the supervision of feature maps from the ground truth. Four key technical contributions are made in the paper. First, pairs of hazy and clean images are used as inputs to supervise the encoding process and obtain high-quality feature maps. Second, the basic GAN formulation is modified by introducing perception loss, style loss, and feature regularization loss to generate better results. Third, multi-scale images are applied as the input to enhance the performance of discriminator. Finally, a hazy remote sensing dataset is created for testing our dehazing method and environmental detection. Extensive experimental results showthat the proposedmethod has achieved better performance than current state-of-the-art methods on both synthetic datasets and real-world remote sensing images. (c) 2020 Elsevier B.V. All rights reserved.
更多查看译文
关键词
Environmental monitoring,Remote sensing,Adversarial generative networks (GAN),Feature-supervised encoder,Multi-scale discriminator
AI 理解论文
溯源树
样例
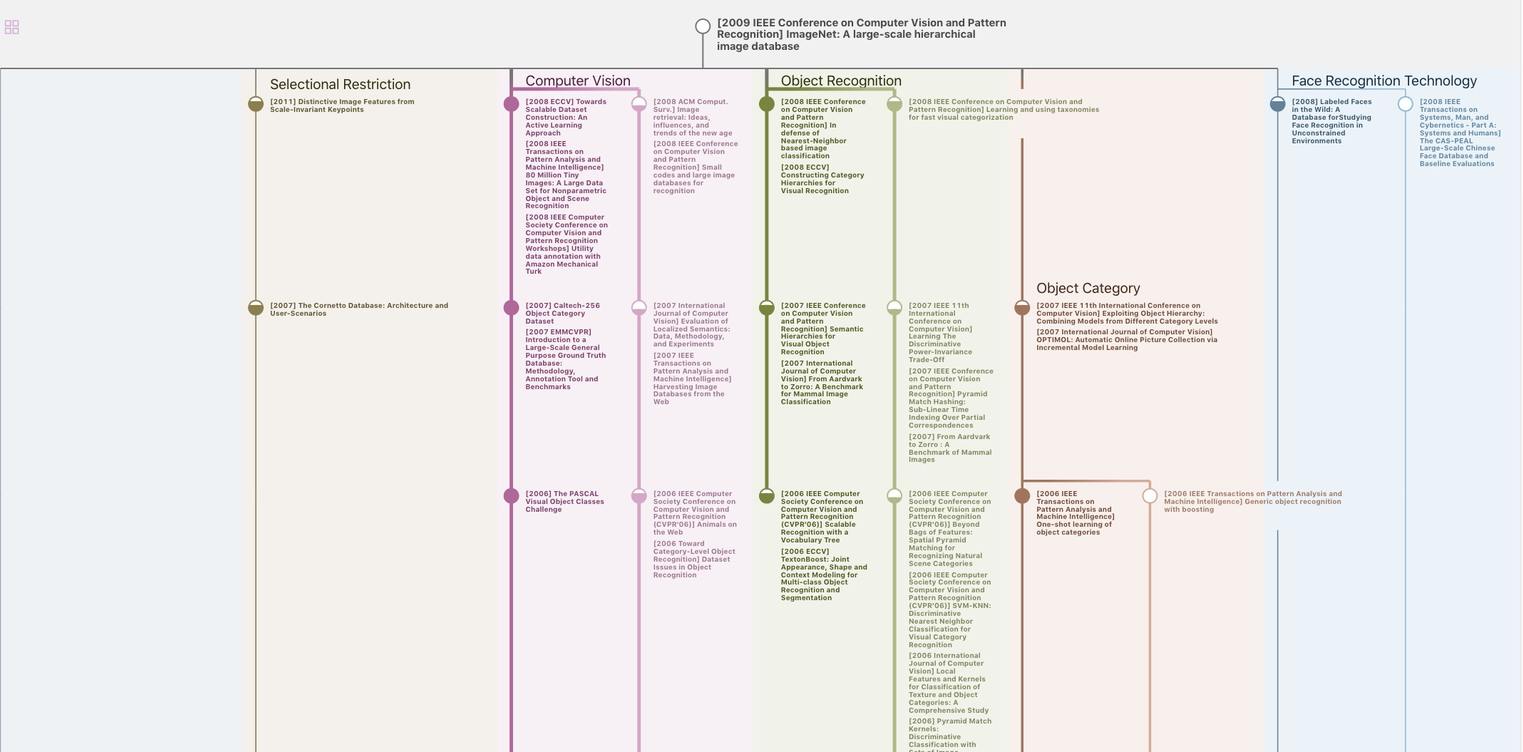
生成溯源树,研究论文发展脉络
Chat Paper
正在生成论文摘要