Graph Signal Processing for Geometric Data and Beyond: Theory and Applications.
IEEE Trans. Multim.(2022)
摘要
Geometric data acquired from real-world scenes, e.g., 2D depth images, 3D point clouds, and 4D dynamic point clouds, have found a wide range of applications including immersive telepresence, autonomous driving, surveillance, etc. Due to irregular sampling patterns of most geometric data, traditional image/video processing methodologies are limited, while Graph Signal Processing (GSP)---a fast-developing field in the signal processing community---enables processing signals that reside on irregular domains and plays a critical role in numerous applications of geometric data from low-level processing to high-level analysis. To further advance the research in this field, we provide the first timely and comprehensive overview of GSP methodologies for geometric data in a unified manner by bridging the connections between geometric data and graphs, among the various geometric data modalities, and with spectral/nodal graph filtering techniques. We also discuss the recently developed Graph Neural Networks (GNNs) and interpret the operation of these networks from the perspective of GSP. We conclude with a brief discussion of open problems and challenges.
更多查看译文
关键词
Three-dimensional displays,Geometry,Symmetric matrices,Manifolds,Laplace equations,Tools,Spectral analysis,Graph Signal Processing (GSP),geometric data,riemannian manifold,graph neural networks (GNNs),interpretability
AI 理解论文
溯源树
样例
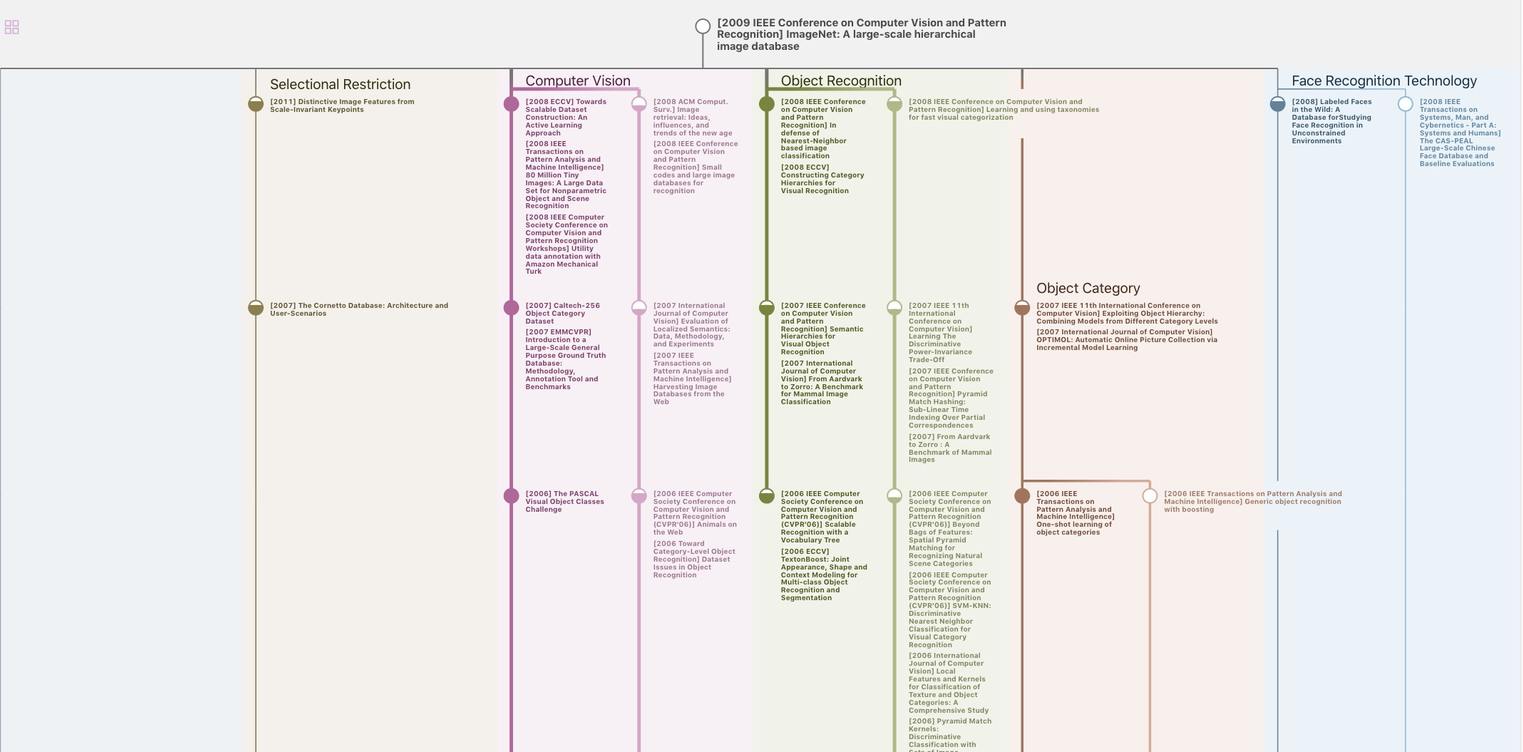
生成溯源树,研究论文发展脉络
Chat Paper
正在生成论文摘要