Convex and Nonconvex Optimization Are Both Minimax-Optimal for Noisy Blind Deconvolution Under Random Designs
arxiv(2023)
摘要
We investigate the effectiveness of convex relaxation and nonconvex optimization in solving bilinear systems of equations under two different designs (i.e., a sort of random Fourier design andGaussian design). Despite the wide applicability, the theoretical understanding about these two paradigms remains largely inadequate in the presence of random noise. The current article makes two contributions by demonstrating that (i) a two-stage nonconvex algorithm attains minimax-optimal accuracy within a logarithmic number of iterations, and (ii) convex relaxation also achieves minimax-optimal statistical accuracy vis-a-vis random noise. Both results significantly improve upon the state-of-the-art theoretical guarantees. Supplementary materials for this article are available online.
更多查看译文
关键词
Blind deconvolution,Bilinear systems of equations,Convex relaxation,Nonconvex optimization,Leave-one-out analysis
AI 理解论文
溯源树
样例
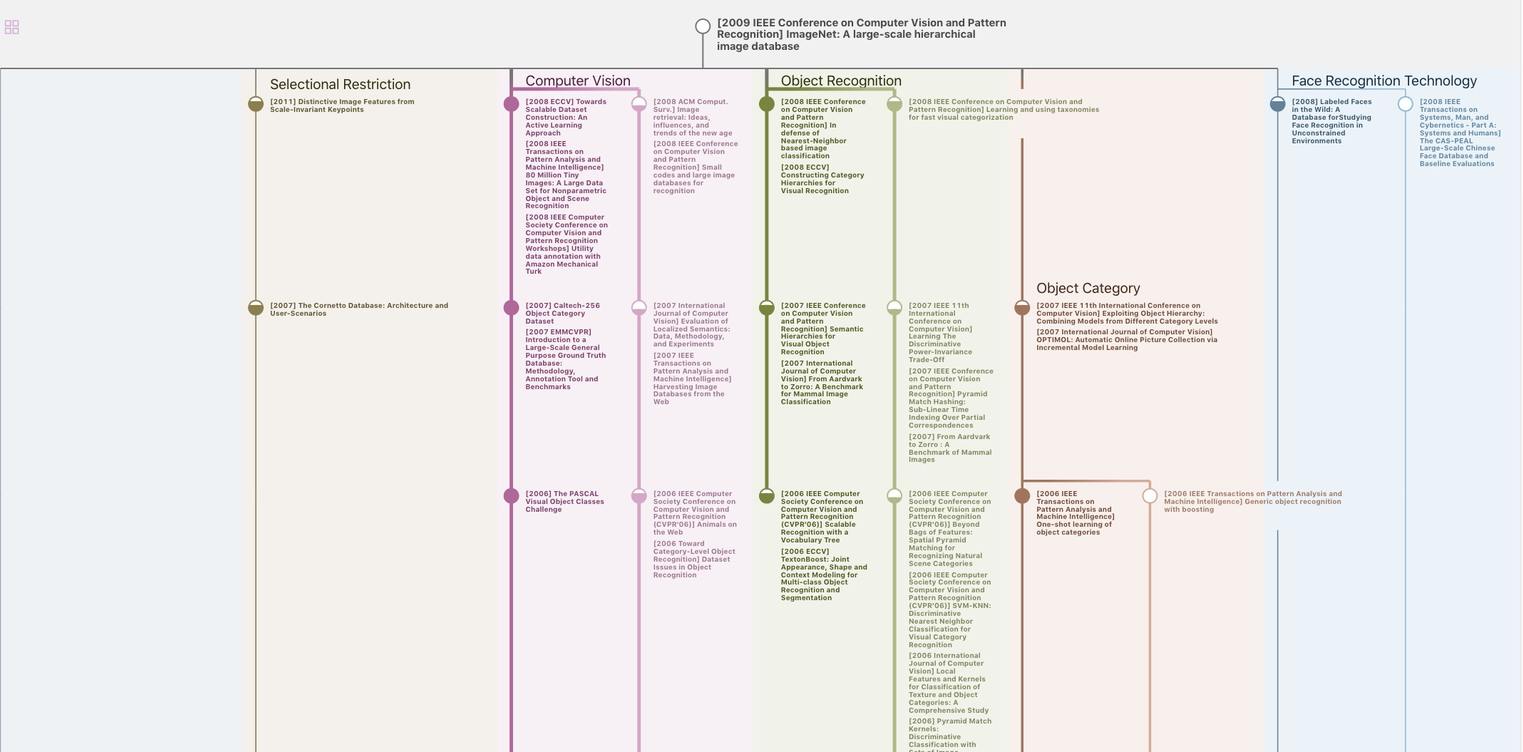
生成溯源树,研究论文发展脉络
Chat Paper
正在生成论文摘要