Verifying Pufferfish Privacy in Hidden Markov Models.
VMCAI(2022)
摘要
Pufferfish is a Bayesian privacy framework for designing and analyzing privacy mechanisms. It refines differential privacy, the current gold standard in data privacy, by allowing explicit prior knowledge in privacy analysis. Through these privacy frameworks, a number of privacy mechanisms have been developed in literature. In practice, privacy mechanisms often need be modified or adjusted to specific applications. Their privacy risks have to be re-evaluated for different circumstances. Moreover, computing devices only approximate continuous noises through floating-point computation, which is discrete in nature. Privacy proofs can thus be complicated and prone to errors. Such tedious tasks can be burdensome to average data curators. In this paper, we propose an automatic verification technique for Pufferfish privacy. We use hidden Markov models to specify and analyze discretized Pufferfish privacy mechanisms. We show that the Pufferfish verification problem in hidden Markov models is NP-hard. Using Satisfiability Modulo Theories solvers, we propose an algorithm to analyze privacy requirements. We implement our algorithm in a prototypical tool called FAIER, and present several case studies. Surprisingly, our case studies show that na\"ive discretization of well-established privacy mechanisms often fail, witnessed by counterexamples generated by FAIER. In discretized \emph{Above Threshold}, we show that it results in absolutely no privacy. Finally, we compare our approach with testing based approach on several case studies, and show that our verification technique can be combined with testing based approach for the purpose of (i) efficiently certifying counterexamples and (ii) obtaining a better lower bound for the privacy budget $\epsilon$.
更多查看译文
关键词
pufferfish privacy,models
AI 理解论文
溯源树
样例
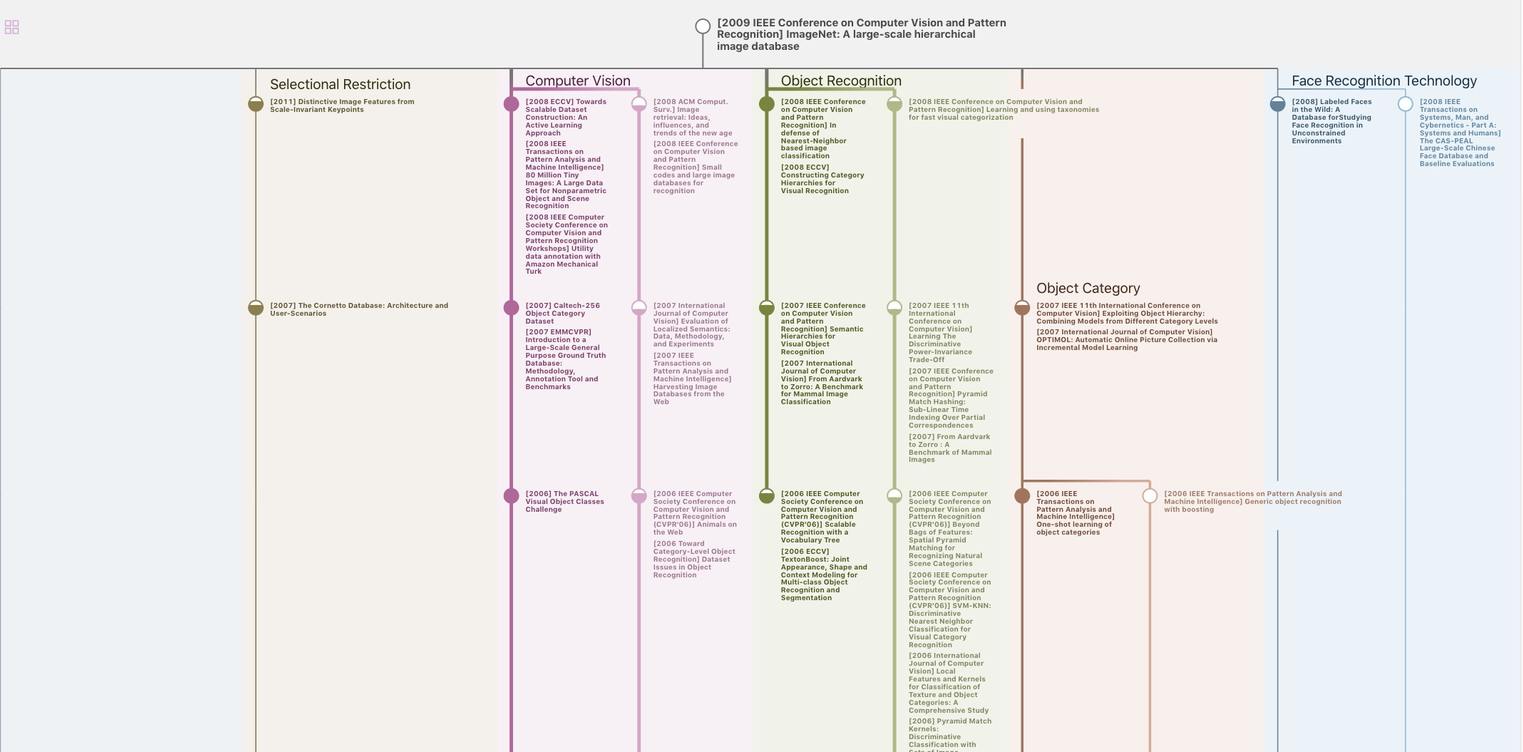
生成溯源树,研究论文发展脉络
Chat Paper
正在生成论文摘要