Capsloc: A Robust Indoor Localization System With Wifi Fingerprinting Using Capsule Networks
ICC 2020 - 2020 IEEE INTERNATIONAL CONFERENCE ON COMMUNICATIONS (ICC)(2020)
摘要
With the unprecedented demand of location-based services in indoor scenarios, wireless indoor localization is emerging as an essential application for mobile users. While the line-of-sight CPS signal is not available at indoor spares. Will fingerprinting using received signal strength (RSS) has become popular with its ubiquitous accessibility. Although the fingerprinting data can be easily collected by portable mobile devices. to achieve robust and efficient indoor localization remains challenging with two constraints. First, the localization accuracy will be degraded by the random fluctuation of signals that caused by multipath effects from RSS signals. Second, indoor localization algorithms are time-consuming due to the handcrafting features and complex filtering on raw dataset. To achieve high localization accuracy with WiFi fingerprinting, in this paper, we propose CapsLoc, a robust indoor localization system by using capsule networks. Specifically, the capsule network model can efficiently extract hierarchical structures from WiFi fingerprint with three main components, including a convolutional layer, a primary capsule layer and a feature capsule layer. We conduct a real-world experimental field test with over 33600 data points. The experimental results show that CapsLoc can achieve accurate indoor localization with an averaged error of 0.68m, which outperforms conventional machine learning methods (KNN and SVM) and existing deep learning methods (CNN and SAE-CNN).
更多查看译文
关键词
Indoor Localization, Capsule Network, RSS Finger-printing, Deep Learning
AI 理解论文
溯源树
样例
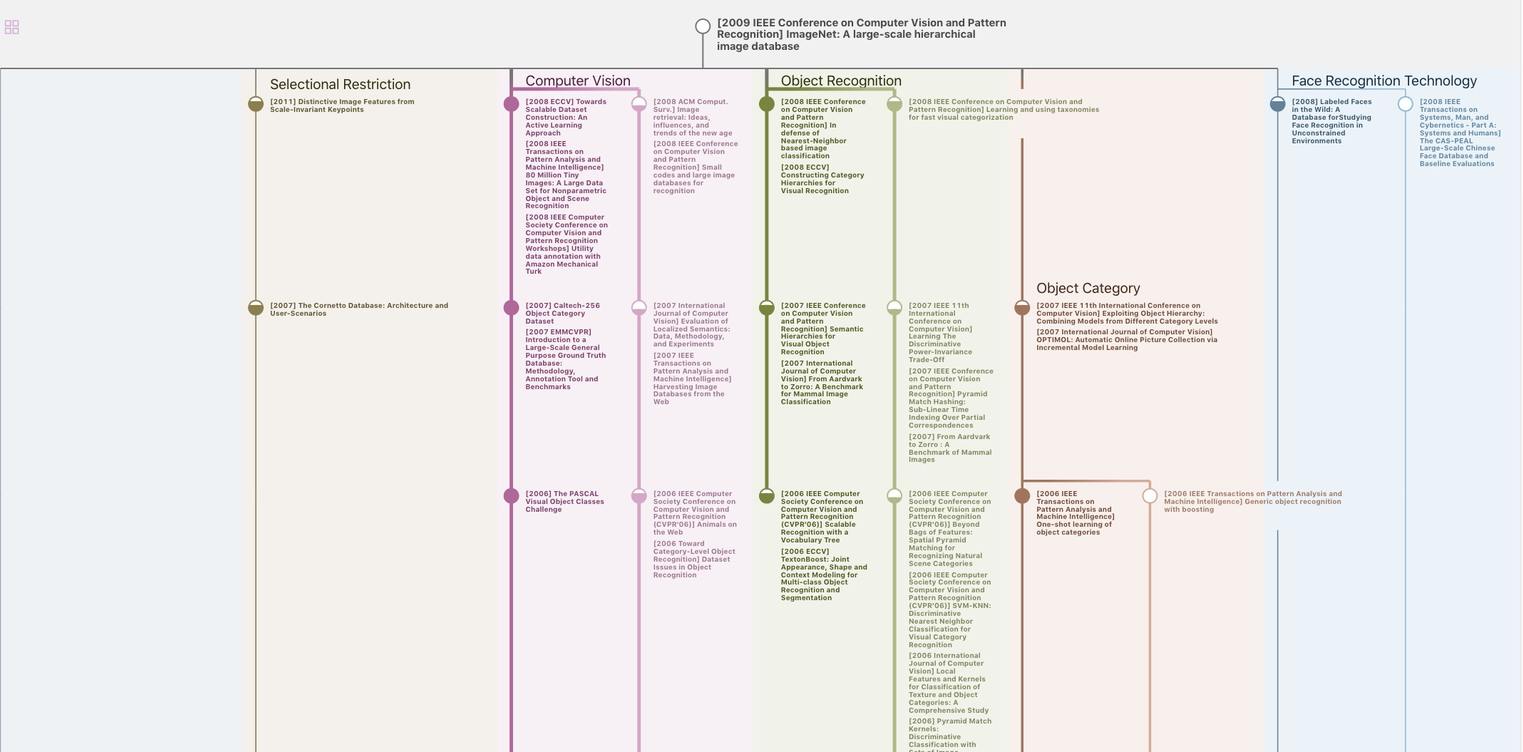
生成溯源树,研究论文发展脉络
Chat Paper
正在生成论文摘要