Deep Mimo Autoprecoder
ICC 2020 - 2020 IEEE INTERNATIONAL CONFERENCE ON COMMUNICATIONS (ICC)(2020)
摘要
In this paper, we propose a novel deep-learning based precoding framework, called deep MIMO autoprecoder, that can be used to learn an optimal precoding policy for multiple-input multiple-output (MIMO) precoding problems, for which optimal solutions are not known. We divide the proposed framework into two steps: action value estimation and optimal action prediction. In the first step, reinforcement learning (RL) is applied to learn and estimate the state-action value function (also called Q-value function) of each discrete precoder in a codebook through interactions with environments of MIMO systems, while for the second step, a supervised learning model is used to predict an optimal precoder in a continuous precoder space that corresponds to the output from the first step.The proposed framework can be implemented by means of two deep neural networks: a deep RL algorithm, called deep Q-network (DQN), and a new neural network, that we call deep Q-value-to-policy network (DQPN). More specifically, an environment-specific DQN is trained for a MIMO scenario in order to estimate the Q-values of each precoder in environmental states. Meanwhile, a single universal DQPN is pretrained offline on the most general MIMO channel model that allows it to achieve generalization over unseen channels. The numerical results verify the effectiveness of the deep MIMO autoprecoder and show that the proposed precoding framework can outperform the conventional approximation algorithm.
更多查看译文
关键词
MIMO, precoding, deep learning, reinforcement, learning, Q-learning, supervised learning
AI 理解论文
溯源树
样例
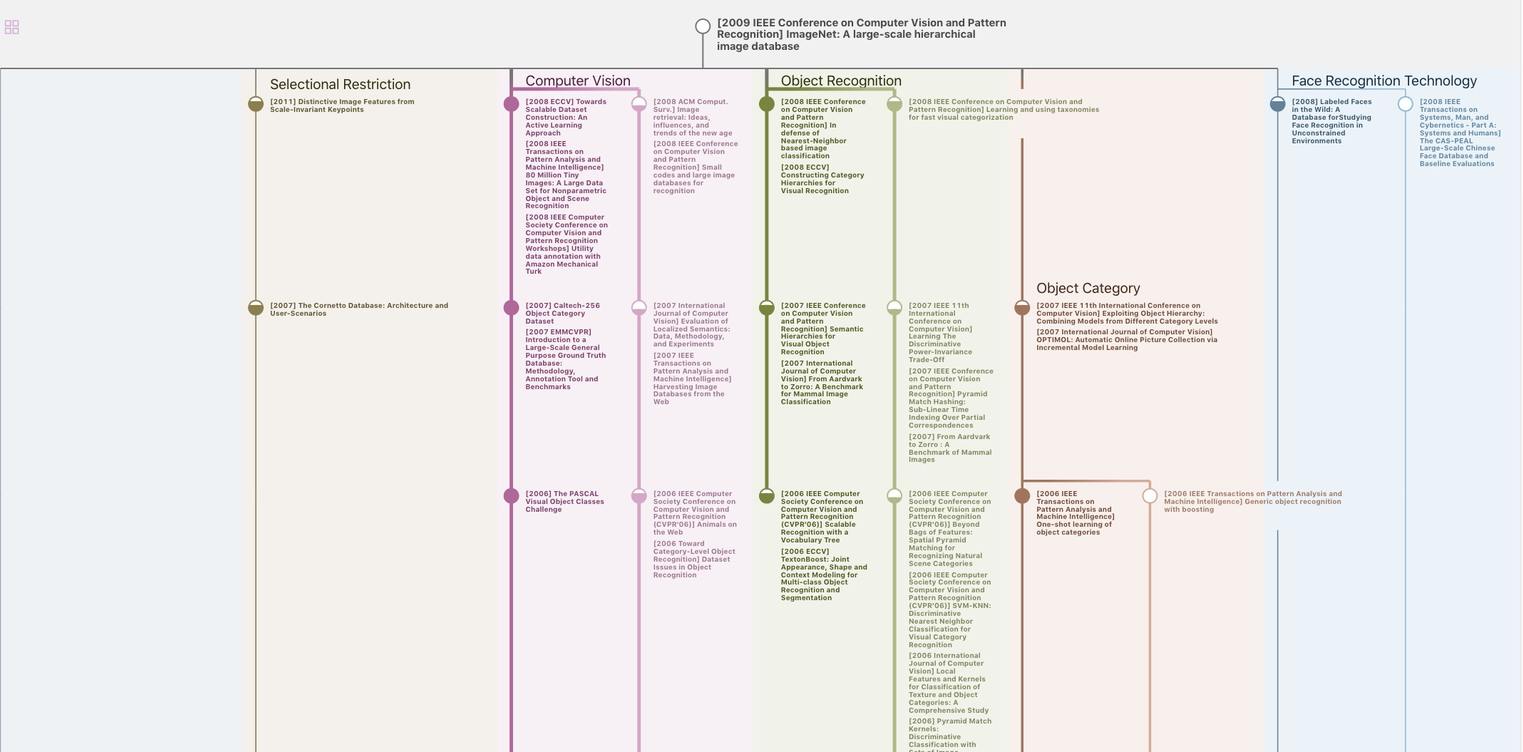
生成溯源树,研究论文发展脉络
Chat Paper
正在生成论文摘要