A Novel Adaptive K-Nn Classifier For Handling Imbalance: Application To Brain Mri
INTELLIGENT DATA ANALYSIS(2020)
摘要
The problem of efficiently classifying imbalanced data has become one of the most challenging tasks in machine learning. Some real world examples include medical image analysis, fraud detection, fault diagnosis, and anomaly detection. Although several data-level algorithms have been developed to address imbalance, they are typically subject to some restrictions. We propose a novel variant of the k-NN family of classifiers, and name this as Density-based Adaptive-distance kNN (DAkNN). It can effectively handle data with skewed distributions and varying class-densities using the concept of adaptive distance. Comparative superiority is experimentally established over related data-level algorithms (SMOTE, ADASYN), using ten sets of two-class data, in terms of geometric mean (of the true positive and negative rates) and accuracy. Additionally, five sets of multi-class data are considered and compared with different variants of k-NN, which are currently very popular. Finally, DAkNN is successfully applied on the highly imbalanced Lower Grade Glioma (LGG) MR images, with an Average-Dice score of 0.9082 for delineating the tumor regions. The results demonstrate clear superiority over state-of-the-art algorithms.
更多查看译文
关键词
k-NN classifier, adaptive distance, skewed data, brain tumors, medical imagery
AI 理解论文
溯源树
样例
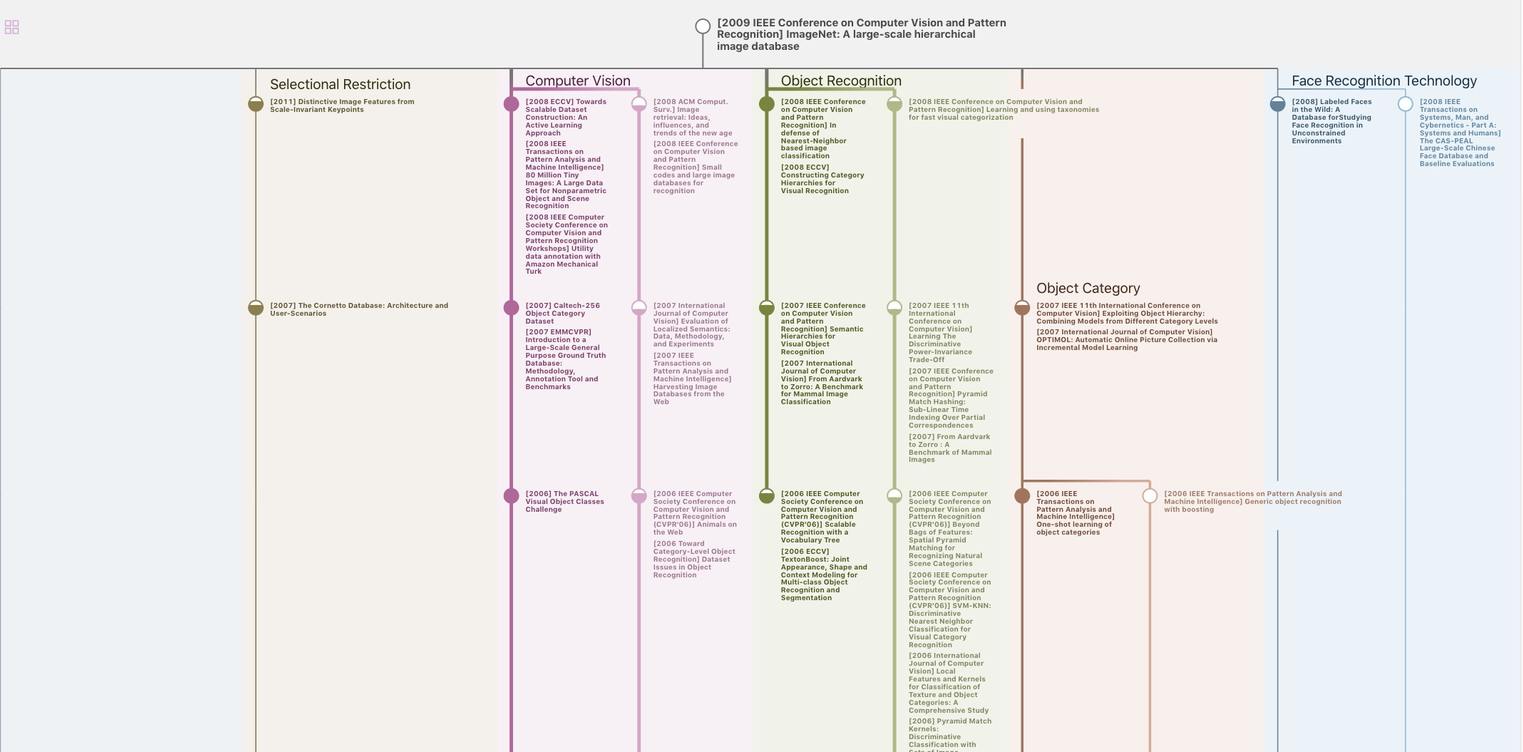
生成溯源树,研究论文发展脉络
Chat Paper
正在生成论文摘要