An Efficient Multi-scale Method for Single Image Super Resolution.
IWCMC(2020)
摘要
Convolutional neural network has shown its superior performance in single image super resolution(SR) as in other computer vision applications. One of its disadvantage is that the computation needs will be significantly higher than that of other applications for the resolution of input is often high definition and it is growing rapidly. In this paper, the low-level vision attributed of super resolution is emphasized by using a multi-scale neural network. The proposed method do not take a very deep framework which is not suitable in ultra-high resolution or mobile terminals. To obtain the geometry structure, a deformable convolution layer is applied in shallow feature extraction which reduces the complexity of the network structure. The deformable convolution scheme constraints the explosion of parameter space and provides shallow features at different scales. The backbone structure is pyramid which offers more scale information for reconstruction. These ideas ensure the efficiency of the model which is also demonstrated on extensive experiments.
更多查看译文
关键词
multi-scale network,deformable convolution,super resolution,pyramid network
AI 理解论文
溯源树
样例
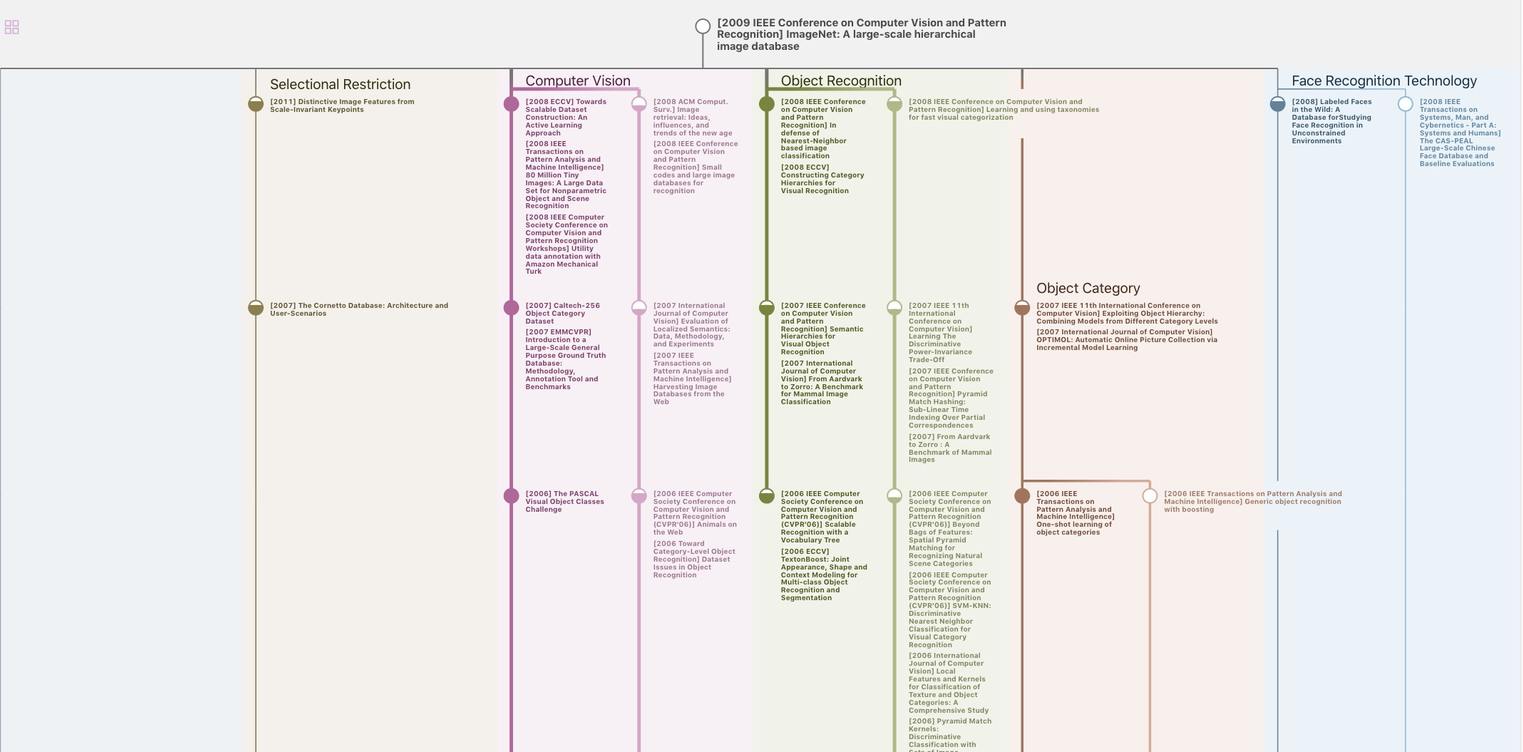
生成溯源树,研究论文发展脉络
Chat Paper
正在生成论文摘要