Prime-Aware Adaptive Distillation
European Conference on Computer Vision(2020)
摘要
Knowledge distillation (KD) aims to improve the performance of a student network by mimicing the knowledge from a powerful teacher network. Existing methods focus on studying what knowledge should be transferred and treat all samples equally during training. This paper introduces the adaptive sample weighting to KD. We discover that previous effective hard mining methods are not appropriate for distillation. Furthermore, we propose Prime-Aware Adaptive Distillation (PAD) by the incorporation of uncertainty learning. PAD perceives the prime samples in distillation and then emphasizes their effect adaptively. PAD is fundamentally different from and would refine existing methods with the innovative view of unequal training. For this reason, PAD is versatile and has been applied in various tasks including classification, metric learning, and object detection. With ten teacher-student combinations on six datasets, PAD promotes the performance of existing distillation methods and outperforms recent state-of-the-art methods.
更多查看译文
关键词
Knowledge distillation,Adaptive weighting,Uncertainty learning
AI 理解论文
溯源树
样例
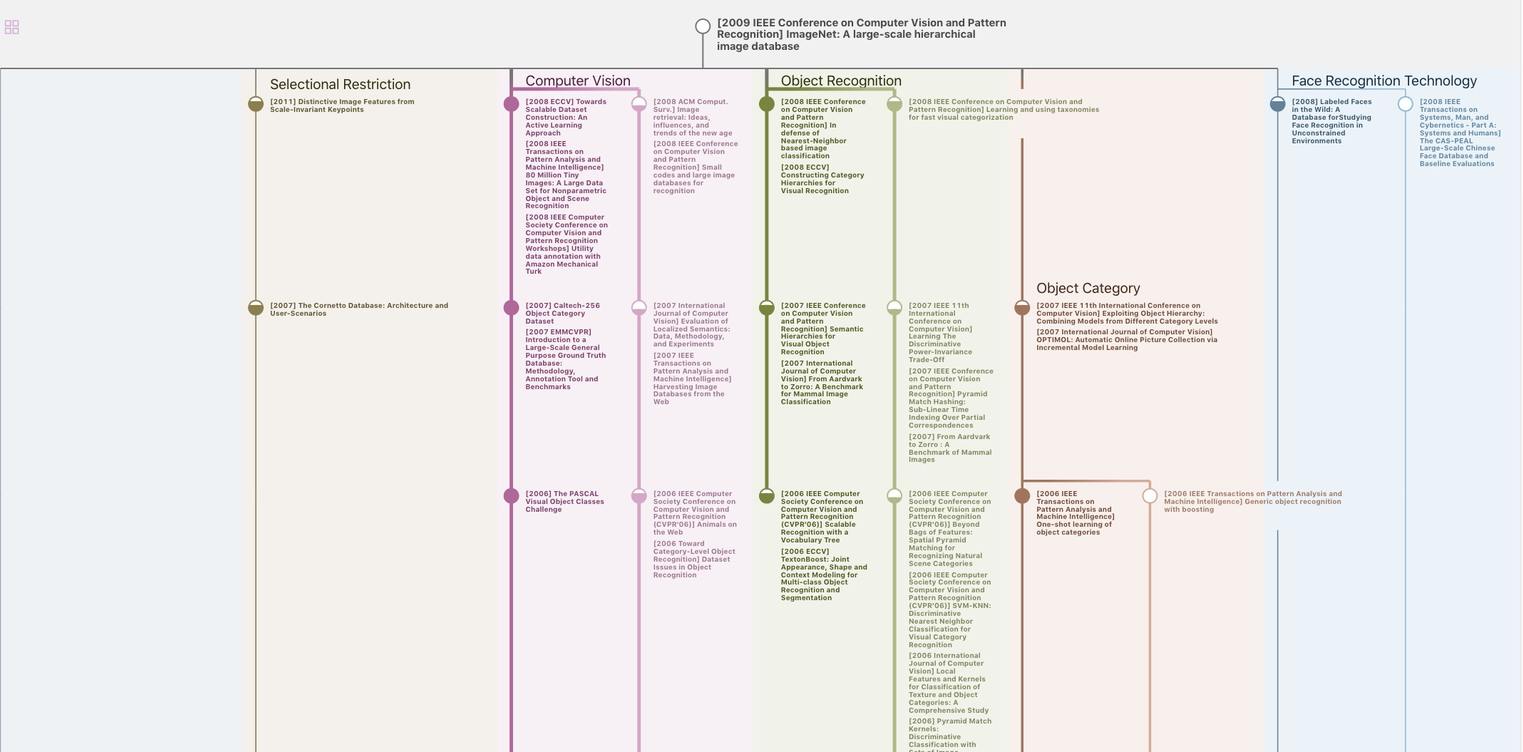
生成溯源树,研究论文发展脉络
Chat Paper
正在生成论文摘要