Learning-Assisted Eavesdropping and Symbol-Level Precoding Countermeasures for Downlink MU-MISO Systems
IEEE Open Journal of the Communications Society(2020)
摘要
In this work, we introduce a machine-learning (ML) based detection attack, where an eavesdropper (Eve) is able to learn the symbol detection function based on precoded pilots. With this ability, an Eve can correctly detect symbols with a high probability. To counteract this attack, we propose a novel symbol-level precoding (SLP) scheme that enhances physical-layer security (PLS) while guaranteeing a constructive interference effect at the intended users. Contrary to conventional SLP schemes, the proposed scheme is robust to the ML-based attack. In particular, the proposed scheme enhances security by designing Eve's received signal to lie at the boundaries of the detection regions. This distinct design causes Eve's detection decisions to be based almost purely on noise. The proposed countermeasure is then extended to account for multi-antennas at the Eve and also for multi-level modulation schemes. In the numerical results, we validate both the detection attack and the countermeasures and show that this gain in security can be achieved at the expense of only a small additional power consumption at the transmitter, and more importantly, these benefits are obtained without affecting the performance at the intended user.
更多查看译文
关键词
Multi-user interference,constructive interference,symbol-level precoding,physical-layer security,machine learning,convex optimization,MISO,bit-error rate
AI 理解论文
溯源树
样例
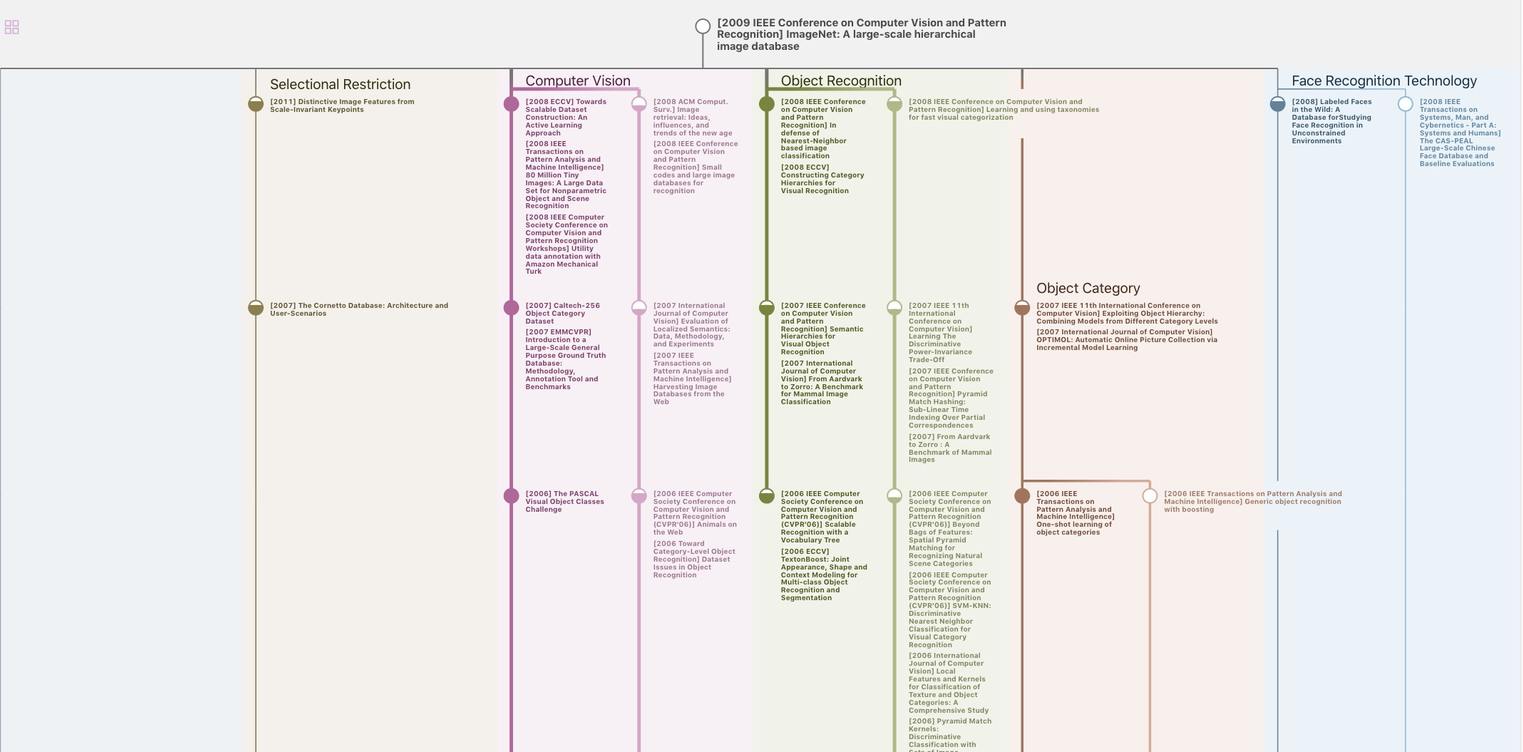
生成溯源树,研究论文发展脉络
Chat Paper
正在生成论文摘要