Conditional as-if analyses in randomized experiments
JOURNAL OF CAUSAL INFERENCE(2021)
摘要
The injunction to "analyze the way you randomize" is well known to statisticians since Fisher advocated for randomization as the basis of inference. Yet even those convinced by the merits of randomization-based inference seldom follow this injunction to the letter. Bernoulli randomized experiments are often analyzed as completely randomized experiments, and completely randomized experiments are analyzed as if they had been stratified; more generally, it is not uncommon to analyze an experiment as if it had been randomized differently. This article examines the theoretical foundation behind this practice within a randomization-based framework. Specifically, we ask when is it legitimate to analyze an experiment randomized according to one design as if it had been randomized according to some other design. We show that a sufficient condition for this type of analysis to be valid is that the design used for analysis should be derived from the original design by an appropriate form of conditioning. We use our theory to justify certain existing methods, question others, and finally suggest new methodological insights such as conditioning on approximate covariate balance.
更多查看译文
关键词
ancillary statistics, causal inference, conditional inference, randomization inference, relevance
AI 理解论文
溯源树
样例
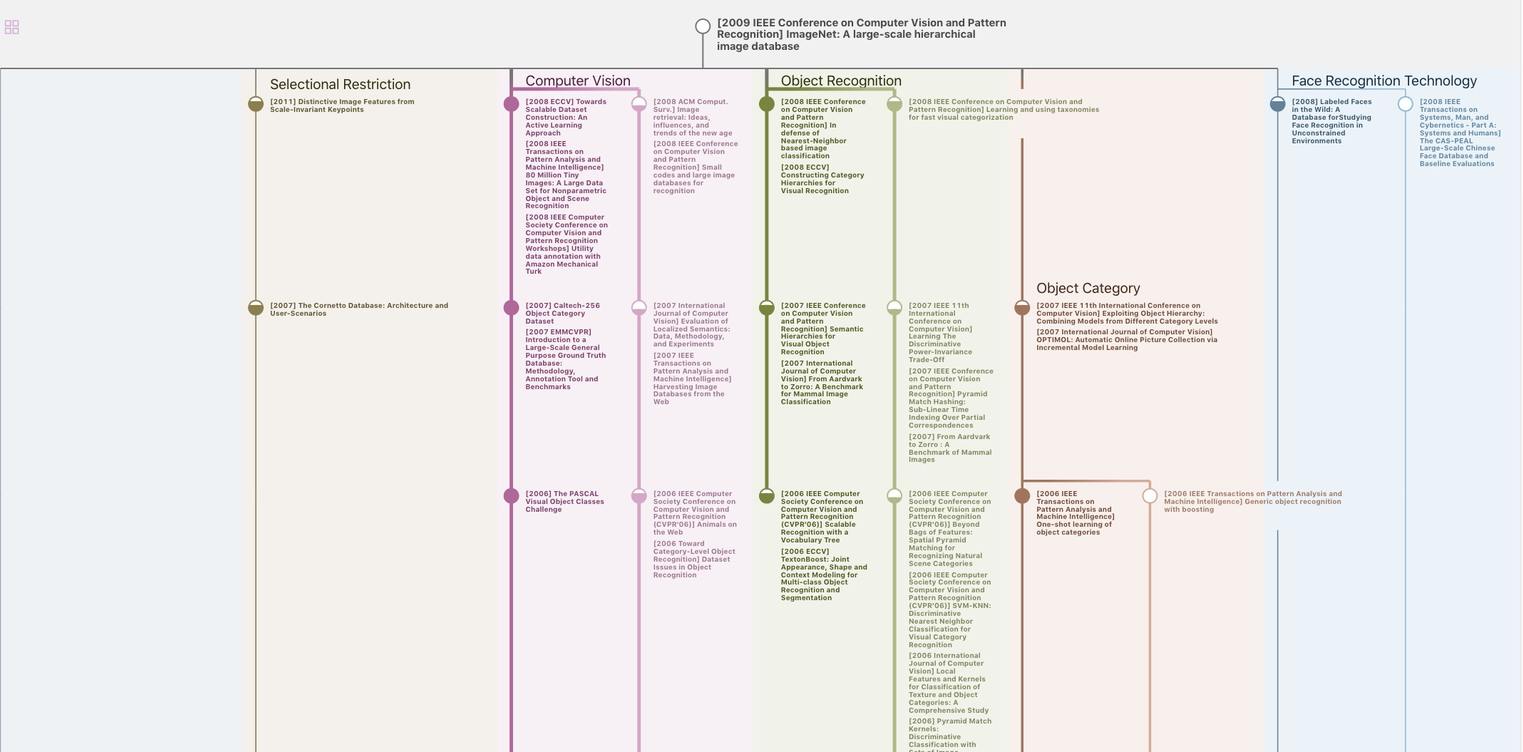
生成溯源树,研究论文发展脉络
Chat Paper
正在生成论文摘要