MusiCoder: A Universal Music-Acoustic Encoder Based on Transformer
MMM (1)(2020)
摘要
Music annotation has always been one of the critical topics in the field of Music Information Retrieval (MIR). Traditional models use supervised learning for music annotation tasks. However, as supervised machine learning approaches increase in complexity, the increasing need for more annotated training data can often not be matched with available data. In this paper, a new self-supervised music acoustic representation learning approach named MusiCoder is proposed. Inspired by the success of BERT, MusiCoder builds upon the architecture of self-attention bidirectional transformers. Two pre-training objectives, including Contiguous Frames Masking (CFM) and Contiguous Channels Masking (CCM), are designed to adapt BERT-like masked reconstruction pre-training to continuous acoustic frame domain. The performance of MusiCoder is evaluated in two downstream music annotation tasks. The results show that MusiCoder outperforms the state-of-the-art models in both music genre classification and auto-tagging tasks. The effectiveness of MusiCoder indicates a great potential of a new self-supervised learning approach to understand music: first apply masked reconstruction tasks to pre-train a transformer-based model with massive unlabeled music acoustic data, and then finetune the model on specific downstream tasks with labeled data.
更多查看译文
关键词
Music information retrieval,Self-supervised representation learning,Masked reconstruction,Transformer
AI 理解论文
溯源树
样例
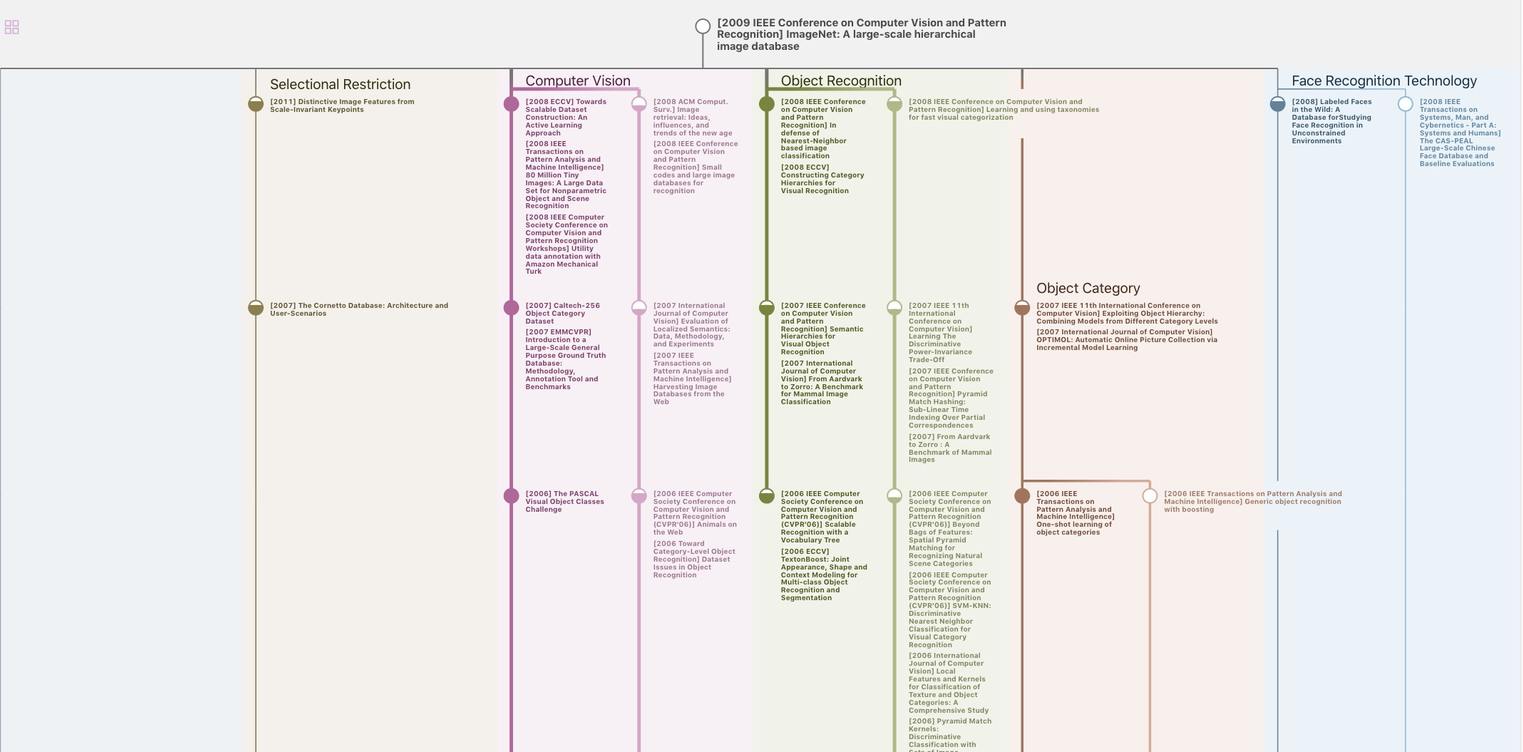
生成溯源树,研究论文发展脉络
Chat Paper
正在生成论文摘要