Tutornet: Towards Flexible Knowledge Distillation For End-To-End Speech Recognition
IEEE-ACM TRANSACTIONS ON AUDIO SPEECH AND LANGUAGE PROCESSING(2021)
摘要
In recent years, there has been a great deal of research in developing end-to-end speech recognition models, which enable simplifying the traditional pipeline and achieving promising results. Despite their remarkable performance improvements, end-to-end models typically require expensive computational cost to show successful performance. To reduce this computational burden, knowledge distillation (KD), which is a popular model compression method, has been used to transfer knowledge from a deep and complex model (teacher) to a shallower and simpler model (student). Previous KD approaches have commonly designed the architecture of the student by reducing the width per layer or the number of layers of the teacher. This structural reduction scheme might limit the flexibility of model selection since the student model structure should be similar to that of the given teacher. To cope with this limitation, we propose a KD method for end-to-end speech recognition, namely TutorNet, that applies KD techniques across different types of neural networks at the hidden representation-level as well as the output-level. For concrete realizations, we firstly apply representation-level knowledge distillation (RKD) during the initialization step, and then apply the softmax-level knowledge distillation (SKD) combined with the original task learning. When the student is trained with RKD, we make use of frame weighting that points out the frames to which the teacher pays more attention. Through a number of experiments, it is verified that TutorNet not only distills the knowledge between networks with different topologies but also significantly contributes to improving the performance of the distilled student.
更多查看译文
关键词
Computational modeling, Hidden Markov models, Speech recognition, Training, Knowledge engineering, Speech processing, Task analysis, Speech recognition, connectionist temporal classification, knowledge distillation, teacher-student learning, transfer learning
AI 理解论文
溯源树
样例
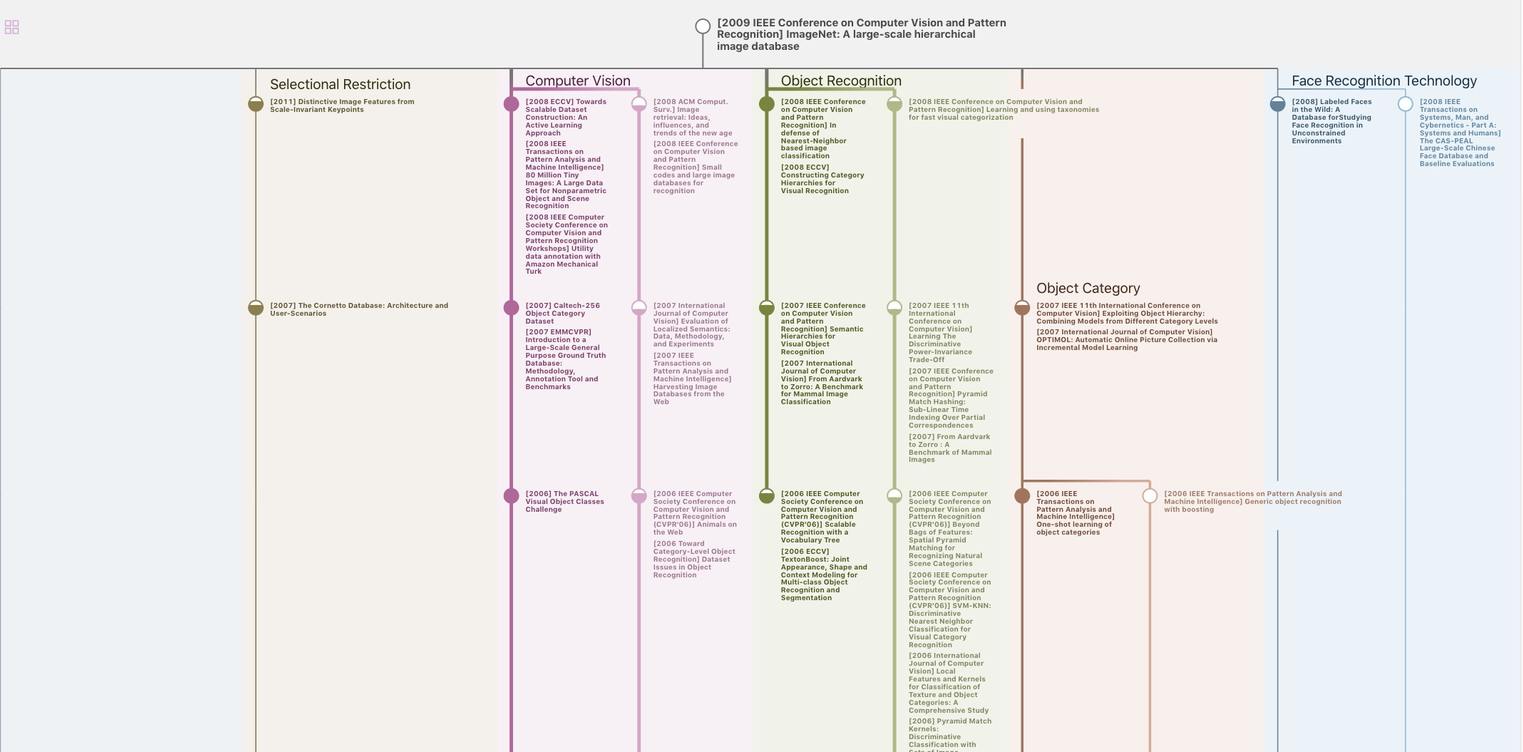
生成溯源树,研究论文发展脉络
Chat Paper
正在生成论文摘要