Deep learning-based classification of posttraumatic stress disorder and depression following trauma utilizing visual and auditory markers of arousal and mood
PSYCHOLOGICAL MEDICINE(2022)
摘要
Background Visual and auditory signs of patient functioning have long been used for clinical diagnosis, treatment selection, and prognosis. Direct measurement and quantification of these signals can aim to improve the consistency, sensitivity, and scalability of clinical assessment. Currently, we investigate if machine learning-based computer vision (CV), semantic, and acoustic analysis can capture clinical features from free speech responses to a brief interview 1 month post-trauma that accurately classify major depressive disorder (MDD) and posttraumatic stress disorder (PTSD). Methods N = 81 patients admitted to an emergency department (ED) of a Level-1 Trauma Unit following a life-threatening traumatic event participated in an open-ended qualitative interview with a para-professional about their experience 1 month following admission. A deep neural network was utilized to extract facial features of emotion and their intensity, movement parameters, speech prosody, and natural language content. These features were utilized as inputs to classify PTSD and MDD cross-sectionally. Results Both video- and audio-based markers contributed to good discriminatory classification accuracy. The algorithm discriminates PTSD status at 1 month after ED admission with an AUC of 0.90 (weighted average precision = 0.83, recall = 0.84, and f1-score = 0.83) as well as depression status at 1 month after ED admission with an AUC of 0.86 (weighted average precision = 0.83, recall = 0.82, and f1-score = 0.82). Conclusions Direct clinical observation during post-trauma free speech using deep learning identifies digital markers that can be utilized to classify MDD and PTSD status.
更多查看译文
关键词
Computer vision, deep learning, depression, digital biomarker, emergency department, landmark feature, posttraumatic stress, resilience, voice analysis
AI 理解论文
溯源树
样例
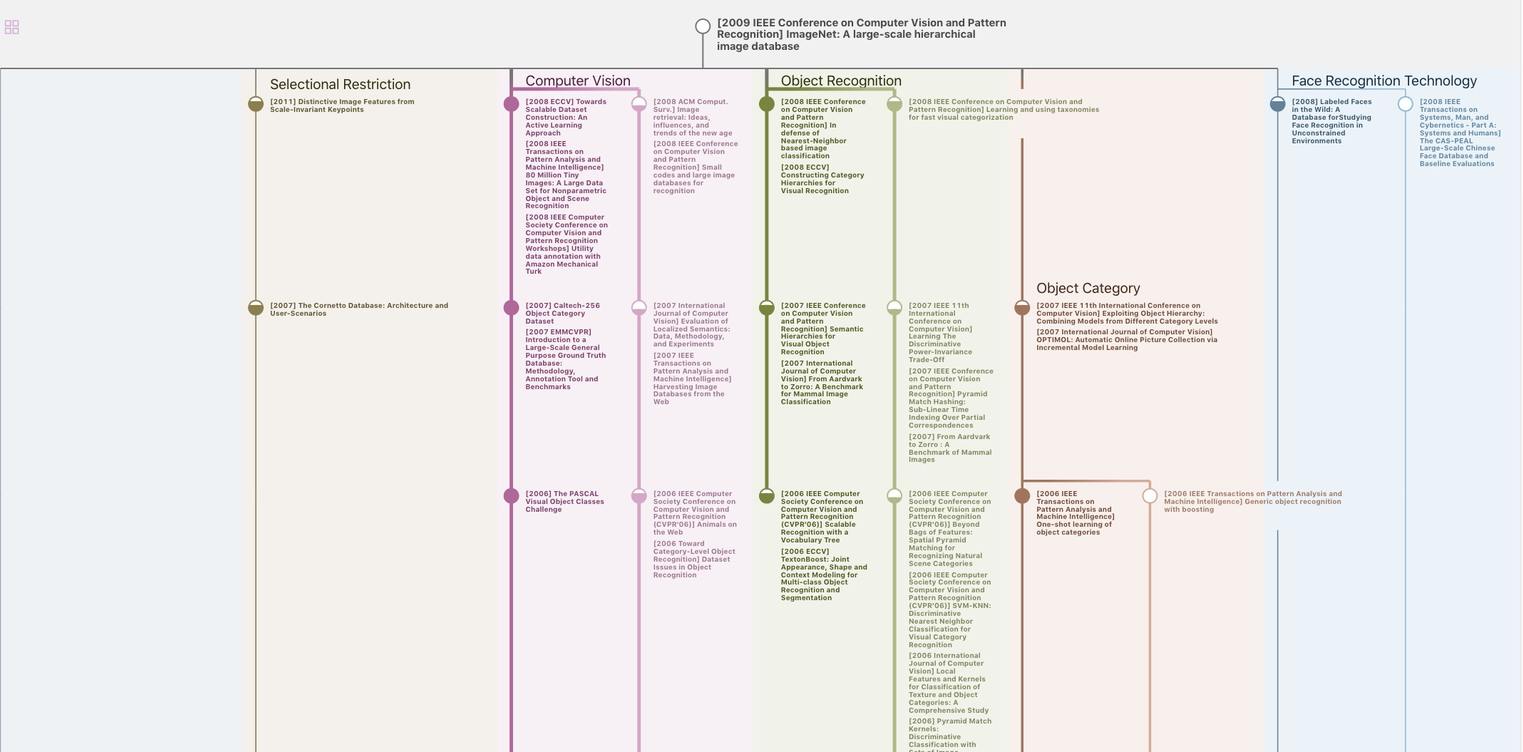
生成溯源树,研究论文发展脉络
Chat Paper
正在生成论文摘要