Stochastic Bundle Adjustment for Efficient and Scalable 3D Reconstruction
European Conference on Computer Vision(2020)
摘要
Current bundle adjustment solvers such as the Levenberg-Marquardt (LM) algorithm are limited by the bottleneck in solving the Reduced Camera System (RCS) whose dimension is proportional to the camera number. When the problem is scaled up, this step is neither efficient in computation nor manageable for a single compute node. In this work, we propose a stochastic bundle adjustment algorithm which seeks to decompose the RCS approximately inside the LM iterations to improve the efficiency and scalability. It first reformulates the quadratic programming problem of an LM iteration based on the clustering of the visibility graph by introducing the equality constraints across clusters. Then, we propose to relax it into a chance constrained problem and solve it through sampled convex program. The relaxation is intended to eliminate the interdependence between clusters embodied by the constraints, so that a large RCS can be decomposed into independent linear sub-problems. Numerical experiments on unordered Internet image sets and sequential SLAM image sets, as well as distributed experiments on large-scale datasets, have demonstrated the high efficiency and scalability of the proposed approach. Codes are released at https://github.com/zlthinker/STBA.
更多查看译文
关键词
Stochastic bundle adjustment,Clustering,3D reconstruction
AI 理解论文
溯源树
样例
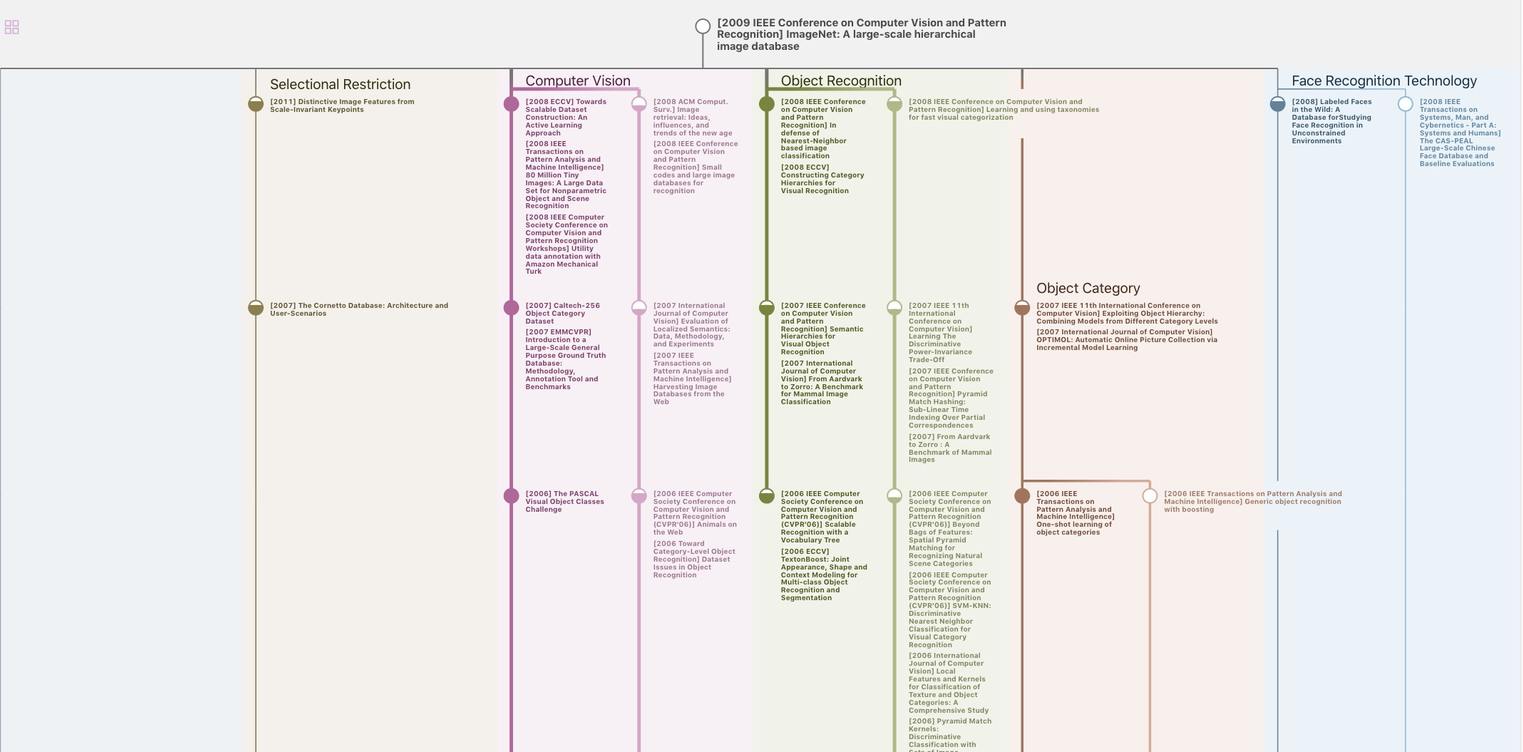
生成溯源树,研究论文发展脉络
Chat Paper
正在生成论文摘要