Distilling Visual Priors from Self-Supervised Learning
ECCV Workshops(2020)
摘要
Convolutional Neural Networks (CNNs) are prone to overfit small training datasets. We present a novel two-phase pipeline that leverages self-supervised learning and knowledge distillation to improve the generalization ability of CNN models for image classification under the data-deficient setting. The first phase is to learn a teacher model which possesses rich and generalizable visual representations via self-supervised learning, and the second phase is to distill the representations into a student model in a self-distillation manner, and meanwhile fine-tune the student model for the image classification task. We also propose a novel margin loss for the self-supervised contrastive learning proxy task to better learn the representation under the data-deficient scenario. Together with other tricks, we achieve competitive performance in the VIPriors image classification challenge.
更多查看译文
关键词
Self-supervised learning,Knowledge-distillation
AI 理解论文
溯源树
样例
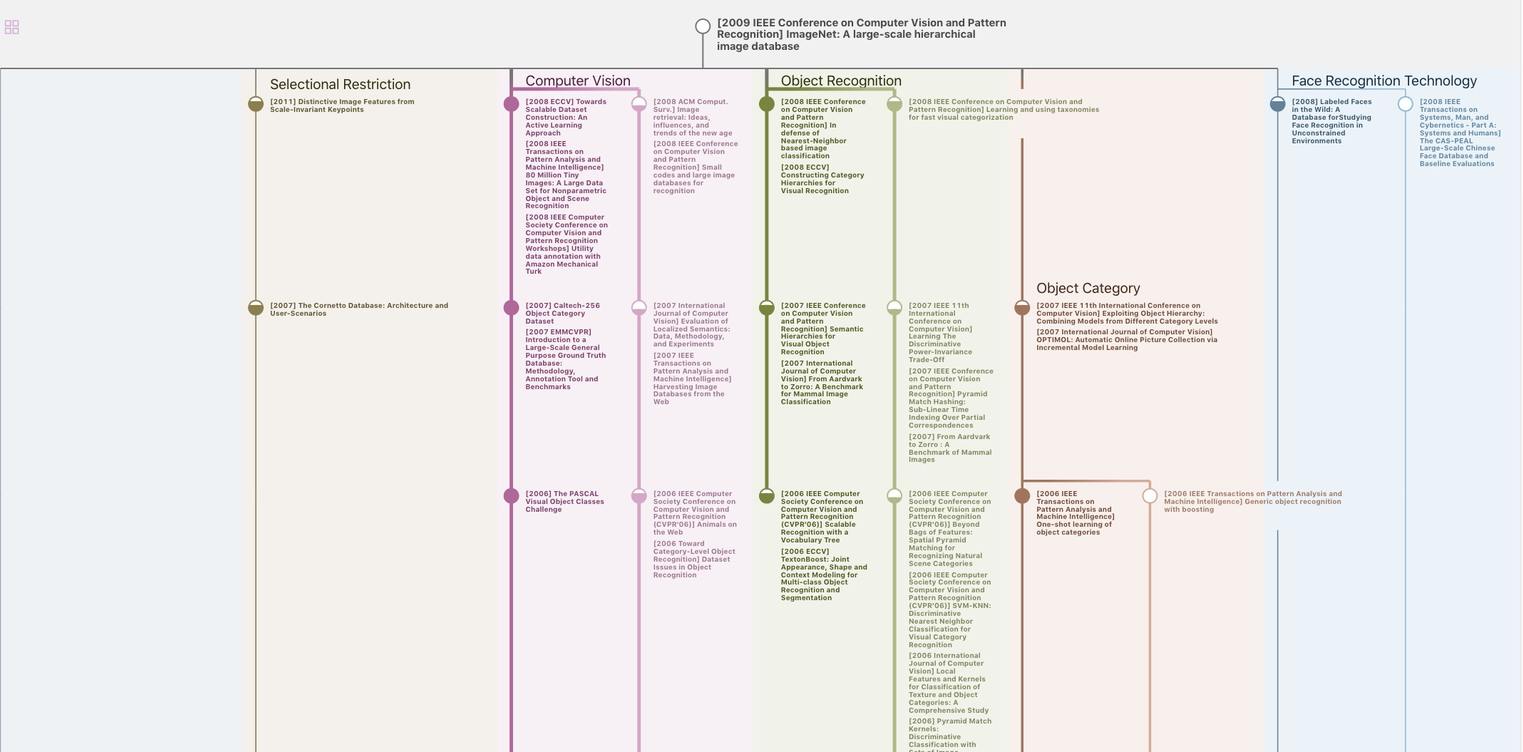
生成溯源树,研究论文发展脉络
Chat Paper
正在生成论文摘要