On Learning Universal Representations Across Languages.
international conference on learning representations(2021)
摘要
Recent studies have demonstrated the overwhelming advantage of cross-lingual pre-trained models (PTMs), such as multilingual BERT and XLM, on cross-lingual NLP tasks. However, existing approaches essentially capture the co-occurrence among tokens through involving the masked language model (MLM) objective with token-level cross entropy. In this work, we extend these approaches to learn sentence-level representations, and show the effectiveness on cross-lingual understanding and generation. We propose Hierarchical Contrastive Learning (HiCTL) to (1) learn universal representations for parallel sentences distributed in one or multiple languages and (2) distinguish the semantically-related words from a shared cross-lingual vocabulary for each sentence. We conduct evaluations on two challenging cross-lingual tasks, XTREME and machine translation. Experimental results show that the HiCTL outperforms the state of the art XLM-R by an absolute gain of 1.3% accuracy on XTREME as well as achieves substantial improvements of +1.7~+3.6 BLEU on both the high-resource and low-resource English-X translation tasks over strong baselines.
更多查看译文
关键词
learning universal representations,languages
AI 理解论文
溯源树
样例
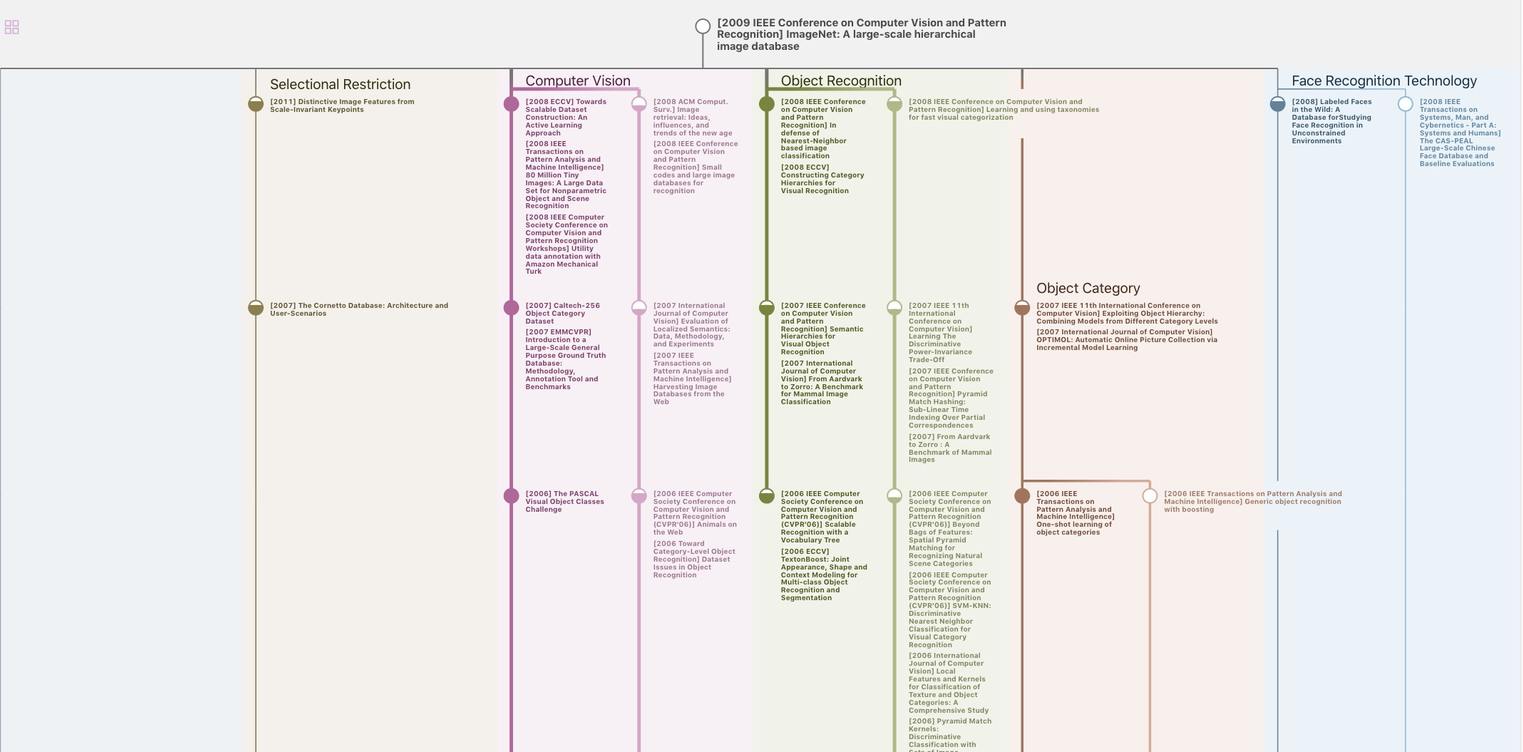
生成溯源树,研究论文发展脉络
Chat Paper
正在生成论文摘要