Cross-View Semantic Segmentation For Sensing Surroundings
IEEE ROBOTICS AND AUTOMATION LETTERS(2020)
摘要
Sensing surroundings plays a crucial role in human spatial perception, as it extracts the spatial configuration of objects as well as the free space from the observations. To facilitate the robot perception with such a surrounding sensing capability, we introduce a novel visual task called Cross-view Semantic Segmentation as well as a framework named View Parsing Network (VPN) to address it. In the cross-view semantic segmentation task, the agent is trained to parse the first-view observations into a top-down-view semantic map indicating the spatial location of all the objects at pixel-level. The main issue of this task is that we lack the real-world annotations of top-down-view data. To mitigate this, we train the VPN in 3D graphics environment and utilize the domain adaptation technique to transfer it to handle real-world data. We evaluate our VPN on both synthetic and real-world agents. The experimental results show that our model can effectively make use of the information from different views and multi-modalities to understanding spatial information. Our further experiment on a LoCoBot robot shows that our model enables the surrounding sensing capability from 2D image input. Code and demo videos can be found at https://view-parsing-network.github.io.
更多查看译文
关键词
Semantic scene understanding, deep learning for visual perception, visual learning, visual-based navigation, computer vision for other robotic applications
AI 理解论文
溯源树
样例
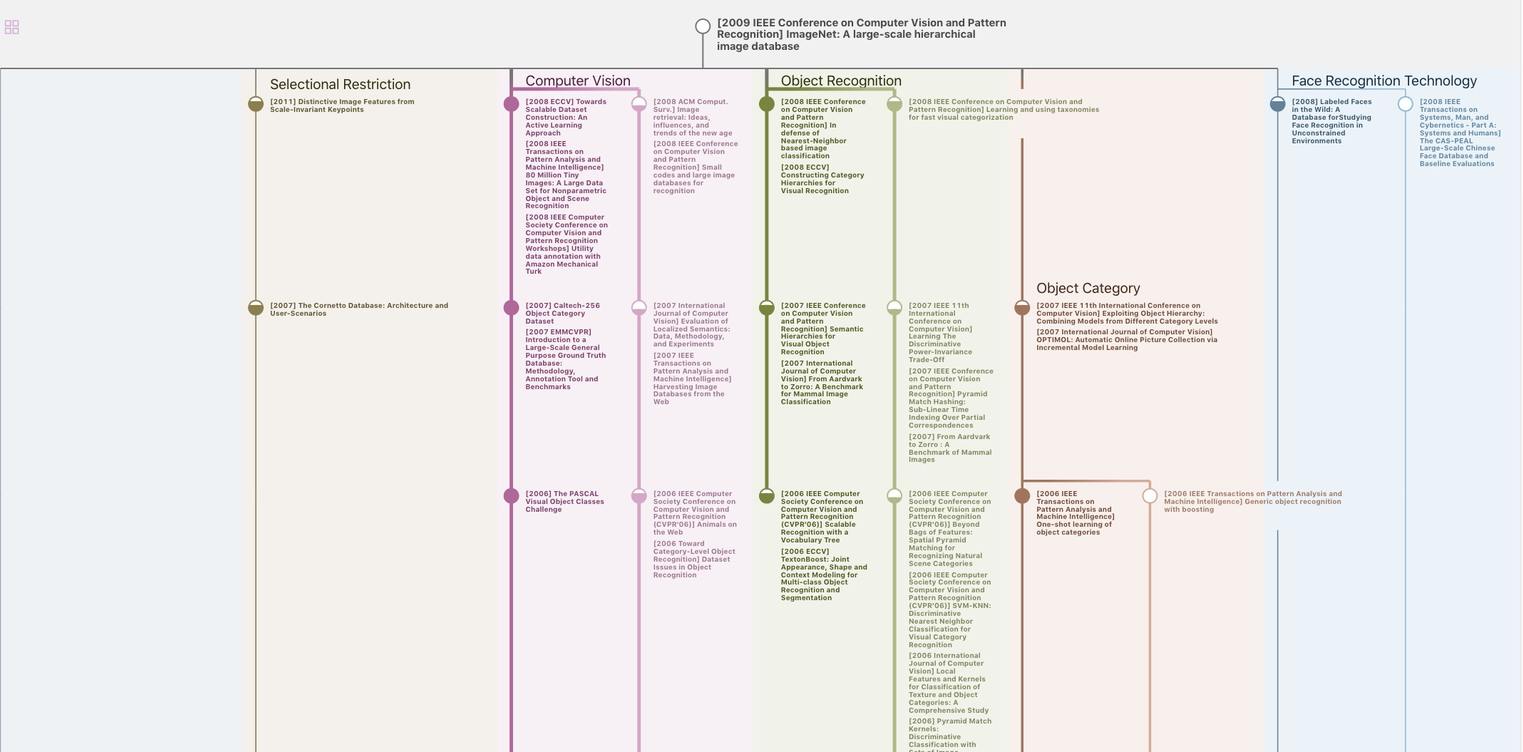
生成溯源树,研究论文发展脉络
Chat Paper
正在生成论文摘要