Coachgan: Fast Adversarial Transfer Learning Between Differently Shaped Entities
ICINCO: PROCEEDINGS OF THE 17TH INTERNATIONAL CONFERENCE ON INFORMATICS IN CONTROL, AUTOMATION AND ROBOTICS(2020)
摘要
In the last decade, robots have been taking an increasingly important place in our societies, and shall the current trend keep the same dynamic,their presence and activities will likely become ubiquitous. As robots will certainly be produced by various industrial actors, it is reasonable to assume that a very diverse robot population will be used by mankind for a broad panel of tasks. As such, it appears probable that robots with a distinct morphology will be required to perform the same task. As an important part of these tasks requires learning-based control and given the millions of interactions steps needed by these approaches to create a single agent, it appears highly desirable to be able to transfer skills from one agent to another despite a potentially different kinematic structure. Correspondingly, this paper introduces a new method, CoachGAN, based on an adversarial framework that allows fast transfer of capacities between a teacher and a student agent. The CoachGAN approach aims at embedding the teacher's way of solving the task within a critic network. Enhanced with the intermediate state variable (ISV) that translates a student state in its teacher equivalent, the critic is then able to guide the student policy in a supervised way in a fraction of the initial training time and without the student having any interaction with the target domain. To demonstrate the flexibility of this approach, CoachGAN is evaluated over a custom tennis task, using various ways to define the intermediate state variables.
更多查看译文
关键词
Transfer Learning, Generative Adversarial Networks, Control, Differentiable Models
AI 理解论文
溯源树
样例
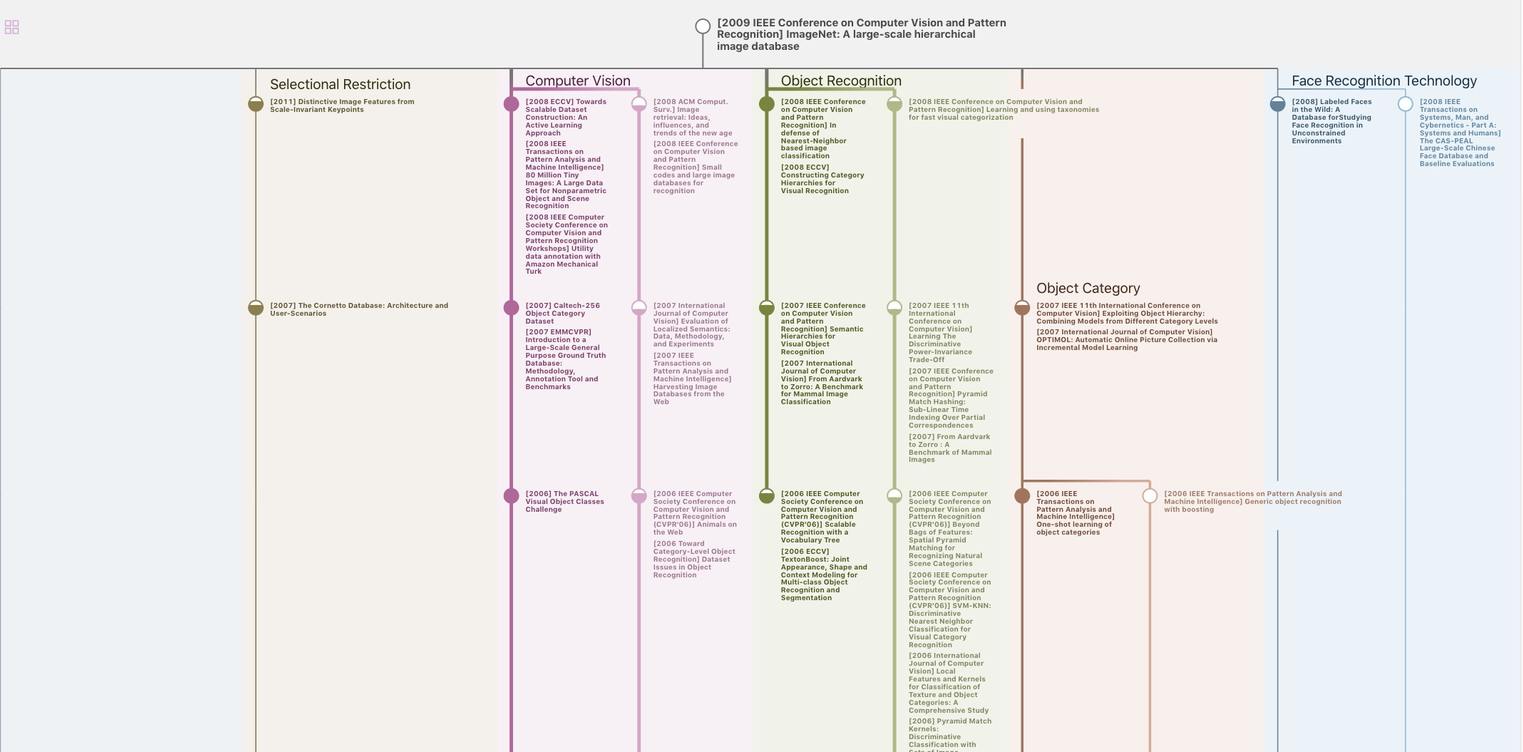
生成溯源树,研究论文发展脉络
Chat Paper
正在生成论文摘要