Dense Scene Multiple Object Tracking with Box-Plane Matching
MM '20: The 28th ACM International Conference on Multimedia Seattle WA USA October, 2020(2020)
摘要
Multiple Object Tracking (MOT) is an important task in computer vision. MOT is still challenging due to the occlusion problem, especially in dense scenes. Following the tracking-by-detection framework, we propose the Box-Plane Matching (BPM) method to improve the MOT performacne in dense scenes. First, we design the Layer-wise Aggregation Discriminative Model (LADM) to filter the noisy detections. Then, to associate remaining detections correctly, we introduce the Global Attention Feature Model (GAFM) to extract appearance feature and use it to calculate the appearance similarity between history tracklets and current detections. Finally, we propose the Box-Plane Matching strategy to achieve data association according to the motion similarity and appearance similarity between tracklets and detections. With the effectiveness of the three modules, our team achieves the 1st place on the Track-1 leaderboard in the ACM MM Grand Challenge HiEve 2020.
更多查看译文
关键词
Multiple object tracking, Box-plane, Detection, Feature extraction
AI 理解论文
溯源树
样例
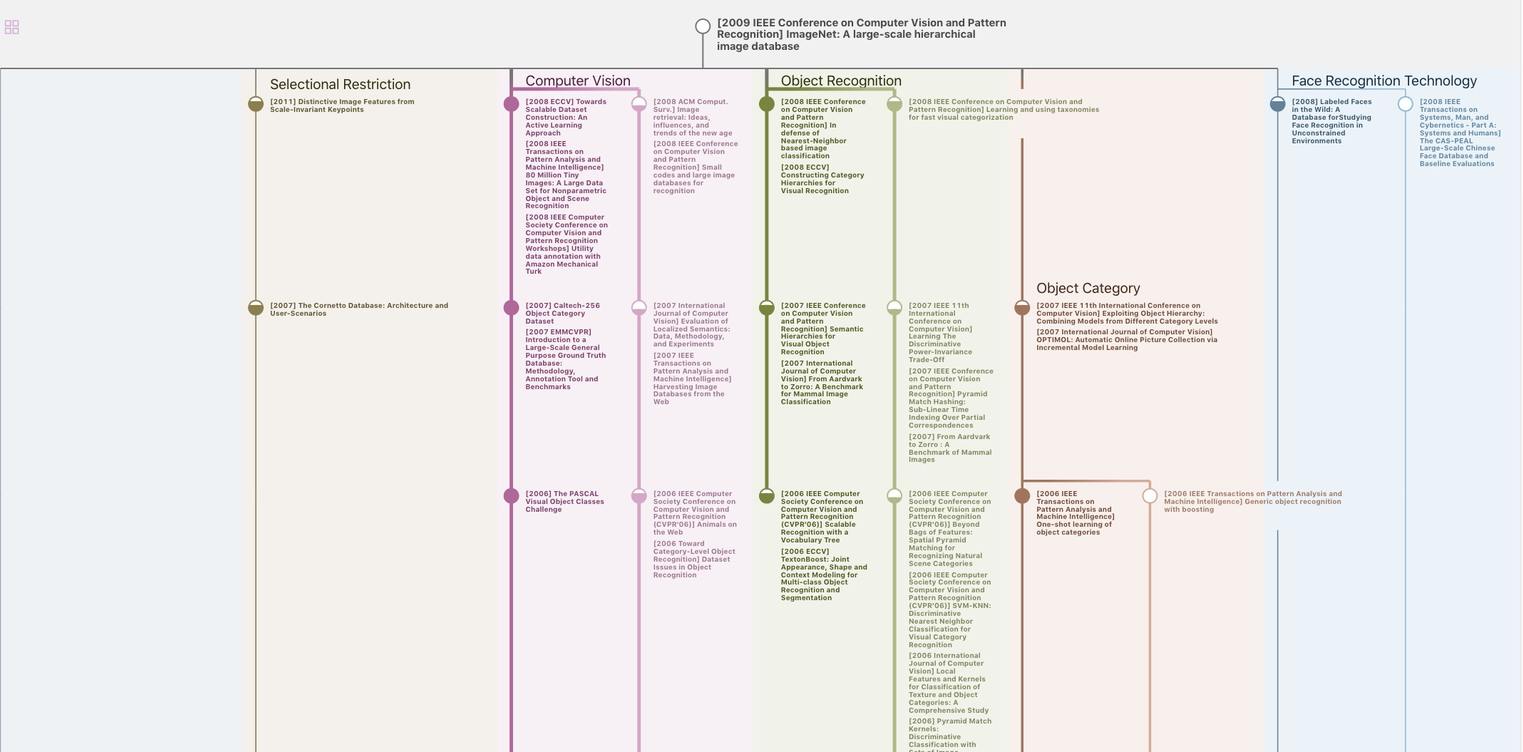
生成溯源树,研究论文发展脉络
Chat Paper
正在生成论文摘要