Analysis Of Personality And Eeg Features In Emotion Recognition Using Machine Learning Techniques To Classify Arousal And Valence Labels
MACHINE LEARNING AND KNOWLEDGE EXTRACTION(2020)
摘要
We analyzed the contribution of electroencephalogram (EEG) data, age, sex, and personality traits to emotion recognition processes-through the classification of arousal, valence, and discrete emotions labels-using feature selection techniques and machine learning classifiers. EEG traits and age, sex, and personality traits were retrieved from a well-known dataset-AMIGOS-and two sets of traits were built to analyze the classification performance. We found that age, sex, and personality traits were not significantly associated with the classification of arousal, valence and discrete emotions using machine learning. The added EEG features increased the classification accuracies (compared with the original report), for arousal and valence labels. Classification of arousal and valence labels achieved higher than chance levels; however, they did not exceed 70% accuracy in the different tested scenarios. For discrete emotions, the mean accuracies and the mean area under the curve scores were higher than chance; however, F1 scores were low, implying that several false positives and false negatives were present. This study highlights the performance of EEG traits, age, sex, and personality traits using emotion classifiers. These findings could help to understand the traits relationship in a technological and data level for personalized human-computer interactions systems.
更多查看译文
关键词
EEG, emotion recognition, human computer interactions, feature selection, machine learning, personality
AI 理解论文
溯源树
样例
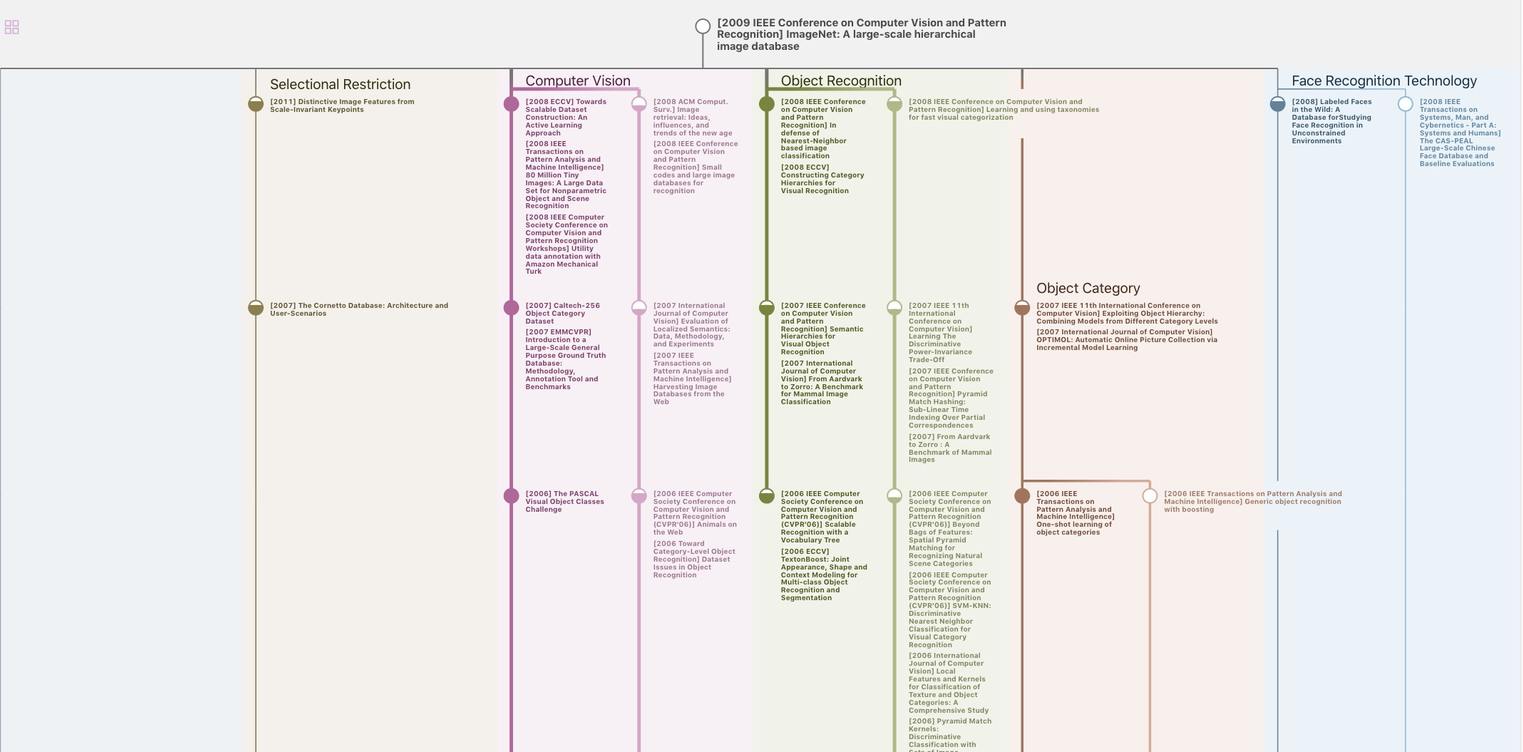
生成溯源树,研究论文发展脉络
Chat Paper
正在生成论文摘要