Understanding Physicians' Online-Offline Behavior Dynamics: An Empirical Study
INFORMATION SYSTEMS RESEARCH(2020)
摘要
Physicians' participation in online healthcare platforms serves to integrate online healthcare resources with the offline medical system. This integration brings opportunities for reshaping healthcare delivery systems. In the field of telemedicine, there has been an extensive discussion about physician participation, but little is known about how physicians actually participate in online healthcare platforms and offline medical systems. Understanding physicians' participation dynamics between online and offline channels is of great importance to academic researchers, practitioners, and policy makers. Such an understanding can reveal insights into how healthcare is actually delivered to patients through both channels, how to contribute to quantifying the social impacts of online healthcare services (Health 2.0), and how to improve healthcare delivery systems. Thus, in this study, we investigate physicians' online-offline behavior dynamics using data from both online and offline channels to conduct our analysis. As physicians' online and offline activities are highly endogenous, we deploy a time-series technique and develop a structural vector autoregression model to examine the behavior dynamics. We find that physicians' online activities can lead to a higher service quantity in offline channels, whereas offline activities may reduce physicians' online services because of resource constraints. Our results also show that the more offline patients physicians serve, the more articles the physicians will likely share online. These findings are robust to various econometric specifications and estimation methods. Our research advocates for the benefits Health 2.0 produces and provides evidence of the value of online healthcare communities and the policies that support them.
更多查看译文
关键词
physicians' online participation, online-offline behavior dynamics, endogenous system, structural vector autoregression (SVAR) models
AI 理解论文
溯源树
样例
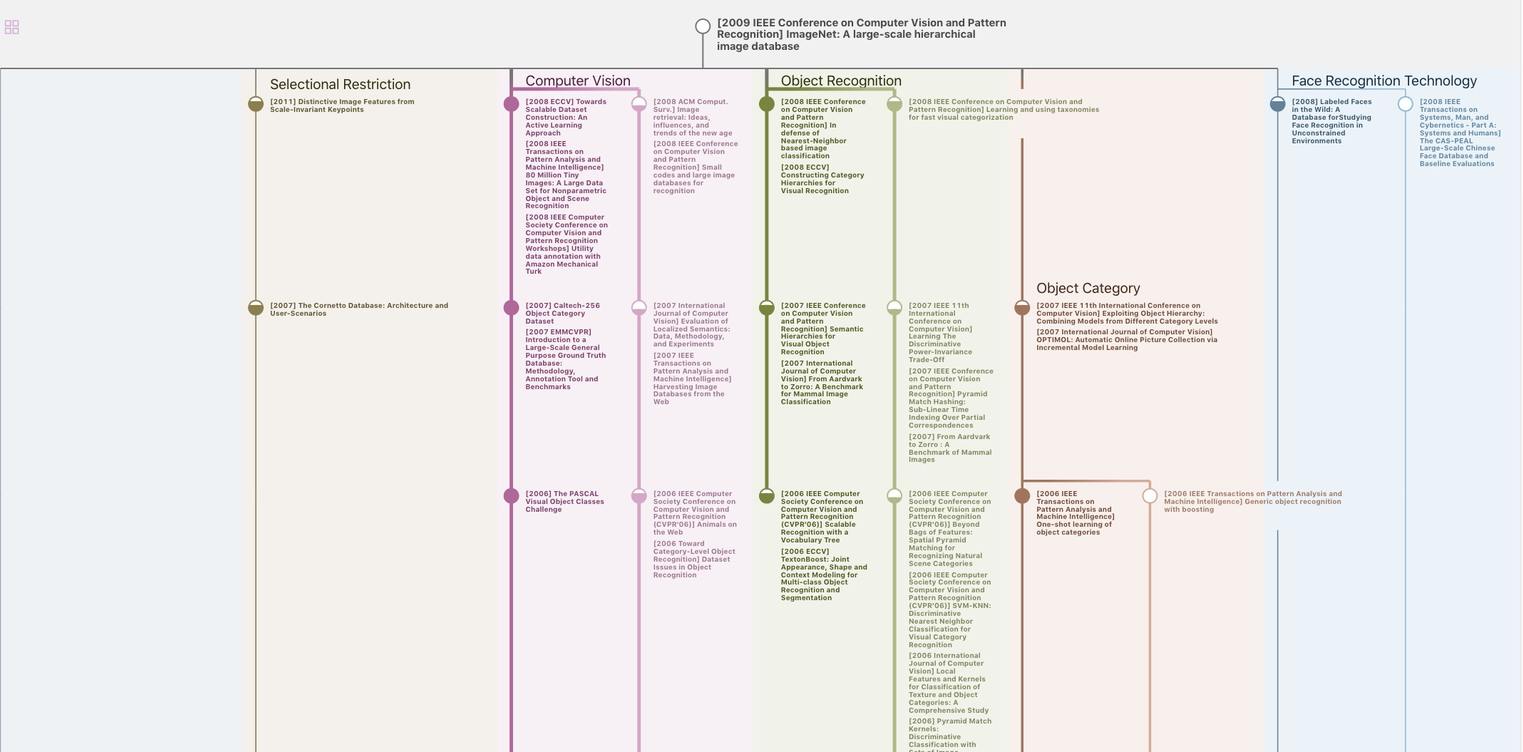
生成溯源树,研究论文发展脉络
Chat Paper
正在生成论文摘要