Development of an Accurate Bedside Swallowing Evaluation Decision Tree Algorithm for Detecting Aspiration in Acute Respiratory Failure Survivors.
Chest(2020)
摘要
BACKGROUND:The bedside swallowing evaluation (BSE) is an assessment of swallowing function and airway safety during swallowing. After extubation, the BSE often is used to identify the risk of aspiration in acute respiratory failure (ARF) survivors.
RESEARCH QUESTION:We conducted a multicenter prospective study of ARF survivors to determine the accuracy of the BSE and to develop a decision tree algorithm to identify aspiration risk.
STUDY DESIGN AND METHODS:Patients extubated after ≥ 48 hours of mechanical ventilation were eligible. Study procedures included the BSE followed by a gold standard evaluation, the flexible endoscopic evaluation of swallowing (FEES).
RESULTS:Overall, 213 patients were included in the final analysis. Median time from extubation to BSE was 25 hours (interquartile range, 21-45 hours). The FEES was completed 1 hour after the BSE (interquartile range, 0.5-2 hours). A total of 33% (70/213; 95% CI, 26.6%-39.2%) of patients aspirated on at least one FEES bolus consistency test. Thin liquids were the most commonly aspirated consistency: 27% (54/197; 95% CI, 21%-34%). The BSE detected any aspiration with an accuracy of 52% (95% CI, 45%-58%), a sensitivity of 83% (95% CI, 74%-92%), and negative predictive value (NPV) of 81% (95% CI, 72%-91%). Using recursive partitioning analyses, a five-variable BSE-based decision tree algorithm was developed that improved the detection of aspiration with an accuracy of 81% (95% CI, 75%-87%), sensitivity of 95% (95% CI, 90%-98%), and NPV of 97% (95% CI, 95%-99%).
INTERPRETATION:The BSE demonstrates variable accuracy to identify patients at high risk for aspiration. Our decision tree algorithm may enhance the BSE and may be used to identify patients at high risk for aspiration, yet requires further validation.
TRIAL REGISTRY:ClinicalTrials.gov; No.: NCT02363686; URL: www.clinicaltrials.gov.
更多查看译文
AI 理解论文
溯源树
样例
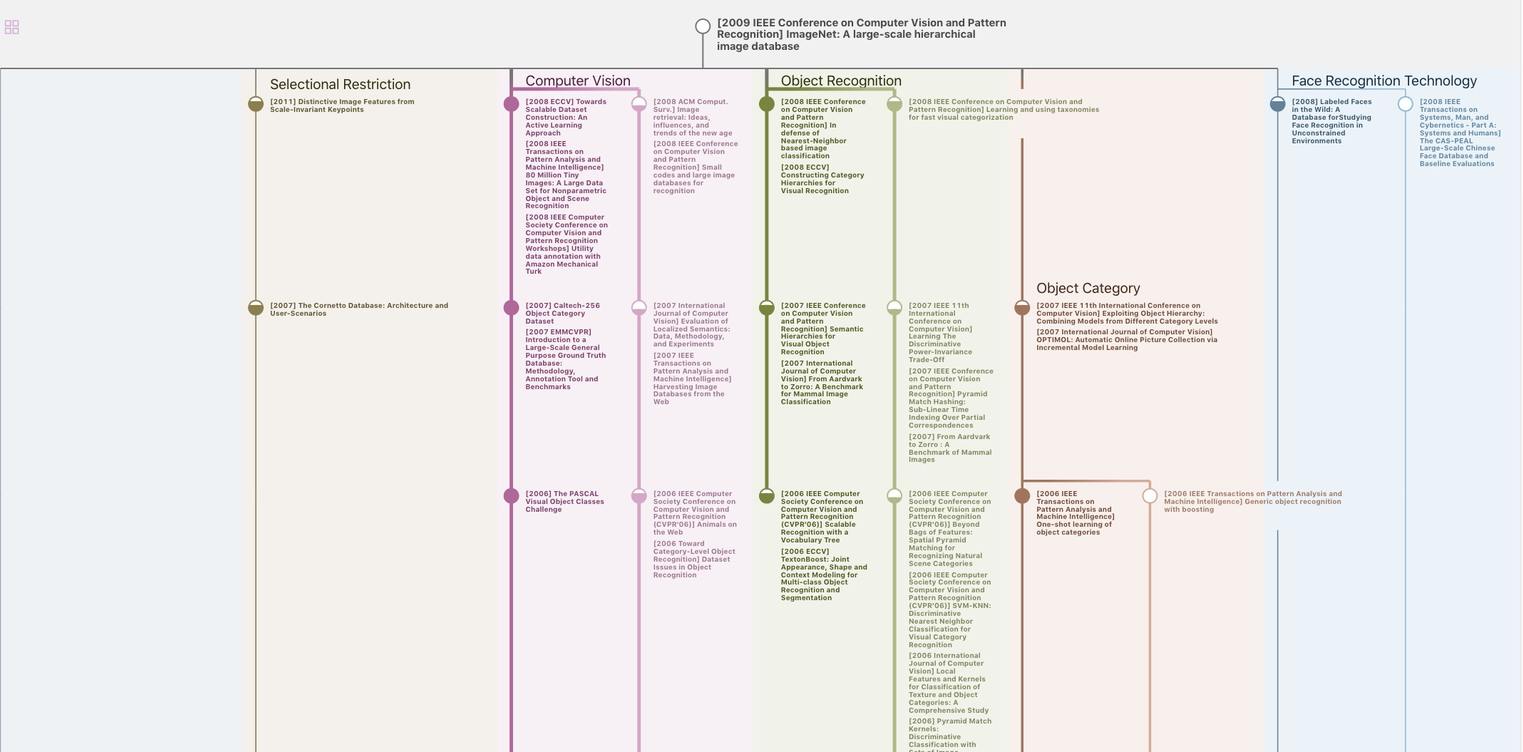
生成溯源树,研究论文发展脉络
Chat Paper
正在生成论文摘要