Deep Learning Approach For Anterior Cruciate Ligament Lesion Detection: Evaluation Of Diagnostic Performance Using Arthroscopy As The Reference Standard
JOURNAL OF MAGNETIC RESONANCE IMAGING(2020)
摘要
Background MRI is the most commonly used imaging method for diagnosing anterior cruciate ligament (ACL) injuries. However, the interpretation of knee MRI is time-intensive and depends on the clinical experience of the reader. An automated detection system based on a deep-learning algorithm may improve interpretation time and reliability. Purpose To determine the feasibility of using a deep learning approach to detect ACL injuries within the knee joint on MRI. Study Type Retrospective. Population In all, 163 subjects with an ACL tear and 245 subjects with an intact ACL. There were 285, 81, and 42 volumes for training, validation, and test sets, respectively. Field Strength/Sequence 2D sagittal proton density-weighted spectral attenuated inversion recovery sequences at 1.5T and 3.0T. Assessment Based on the architecture of 3D DenseNet, we constructed a classification convolutional neural network. We tested this deep learning approach with different inputs and two other algorithms, including VGG16 and ResNet. Then we had both inexperienced radiologists and senior radiologists read the MR images. Statistical Tests Using arthroscopic results as the reference standard, the performance of three different inputs and three different algorithms, the residents and senior radiologists assessed the classification accuracy, sensitivity, specificity, positive predictive value (PPV), negative predictive value (NPV), and area under the receiver operating characteristic curve (AUC). Results The accuracy, sensitivity, specificity, PPV, and NPV of our customized 3D deep learning architecture was 0.957, 0.976, 0.944, 0.940, and 0.976, respectively. The average AUCs were 0.946, 0.859, 0.960 for ResNet, VGG16, and our proposed network, respectively. The diagnostic accuracy of our model, residents, and senior radiologists was 0.957, 0.814, and 0.899, respectively. Data Conclusion Our study demonstrated the feasibility of using an automated deep-learning-based detection system to evaluate ACL injury. Level of Evidence 3 Technical Efficacy Stage 1
更多查看译文
关键词
deep learning, magnetic resonance imaging, anterior cruciate ligament injury, convolutional neural network
AI 理解论文
溯源树
样例
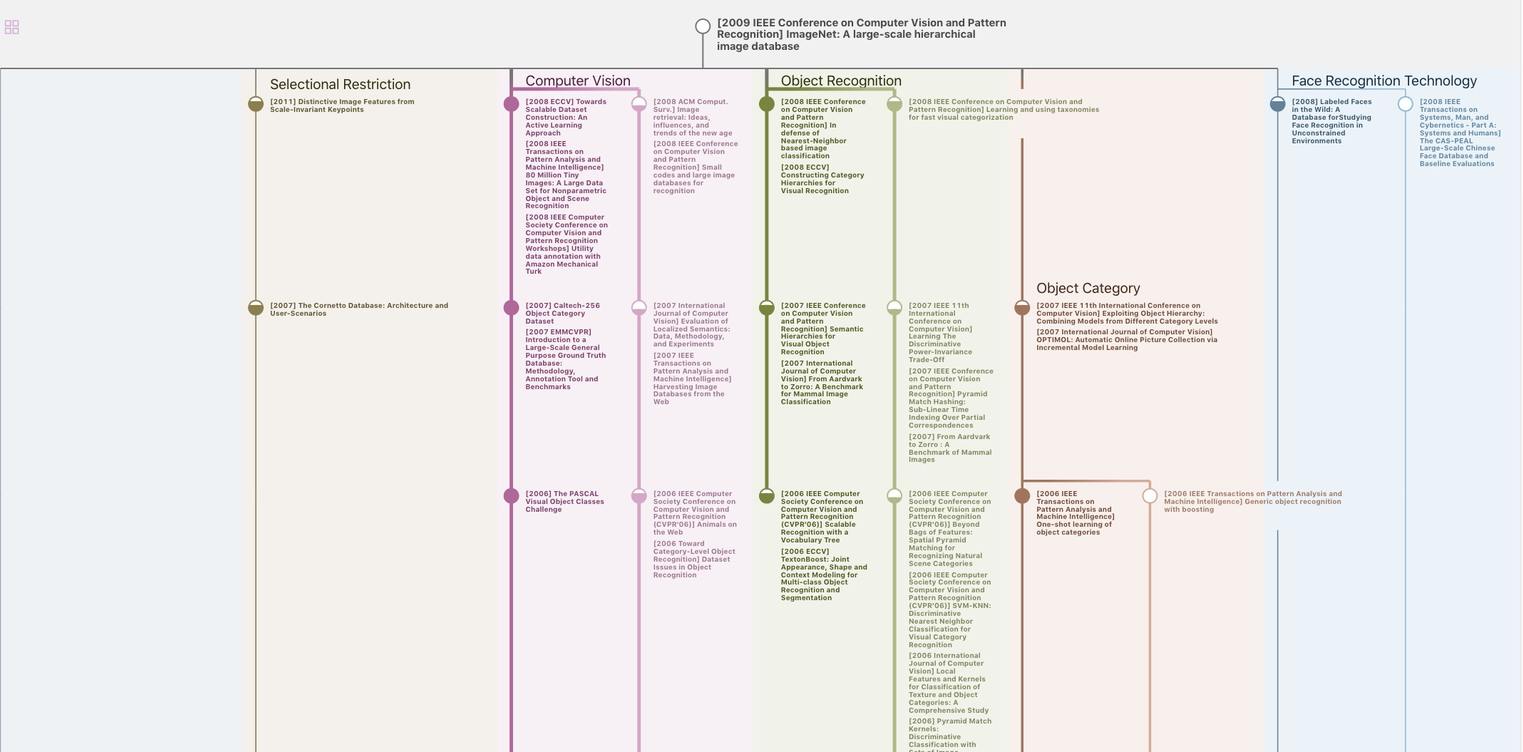
生成溯源树,研究论文发展脉络
Chat Paper
正在生成论文摘要