Uncertainty aware kernel estimation and edge preserving attention for blind image deblurring
2023 IEEE 9TH INTERNATIONAL WORKSHOP ON COMPUTATIONAL ADVANCES IN MULTI-SENSOR ADAPTIVE PROCESSING, CAMSAP(2023)
摘要
Blind deconvolution is a challenging problem because of its ill-posed nature. Most existing blind deconvolution techniques are based on classical methods and utilize a maximum a posterior (MAP) framework to estimate clean images and blur kernels. Very recently, a method that utilizes the Deep Image Prior (DIP) principle has been proposed. This method uses two generative networks to model the deep priors of clean image and blur kernel. But this method fails for complex kernels, and estimates erroneous kernels, hence leading to ringing artifacts in the reconstructed image. To address this issue and estimate better kernels, we introduce a Bayesian uncertainty guided kernel estimation technique. Also, to improve the quality of the reconstructed images, we present a new type of edge-preserving attention. We perform evaluations on several benchmark datasets to show the performance improvement obtained by our network.
更多查看译文
关键词
Kernel Estimation,Edge Preservation,Image Deblurring,Benchmark Datasets,Clear Image,Prior Imaging,Kernel Images,Blind Deconvolution,Blur Kernel,Feature Space,Qualitative Results,Additive Noise,Network Layer,Convolution Operation,Trainable Parameters,Network Output,Attention Module,Feature Channels,Epistemic Uncertainty,Fully-connected Network,Latent Image,Hand-held Camera,Refinement Network,Good Extent
AI 理解论文
溯源树
样例
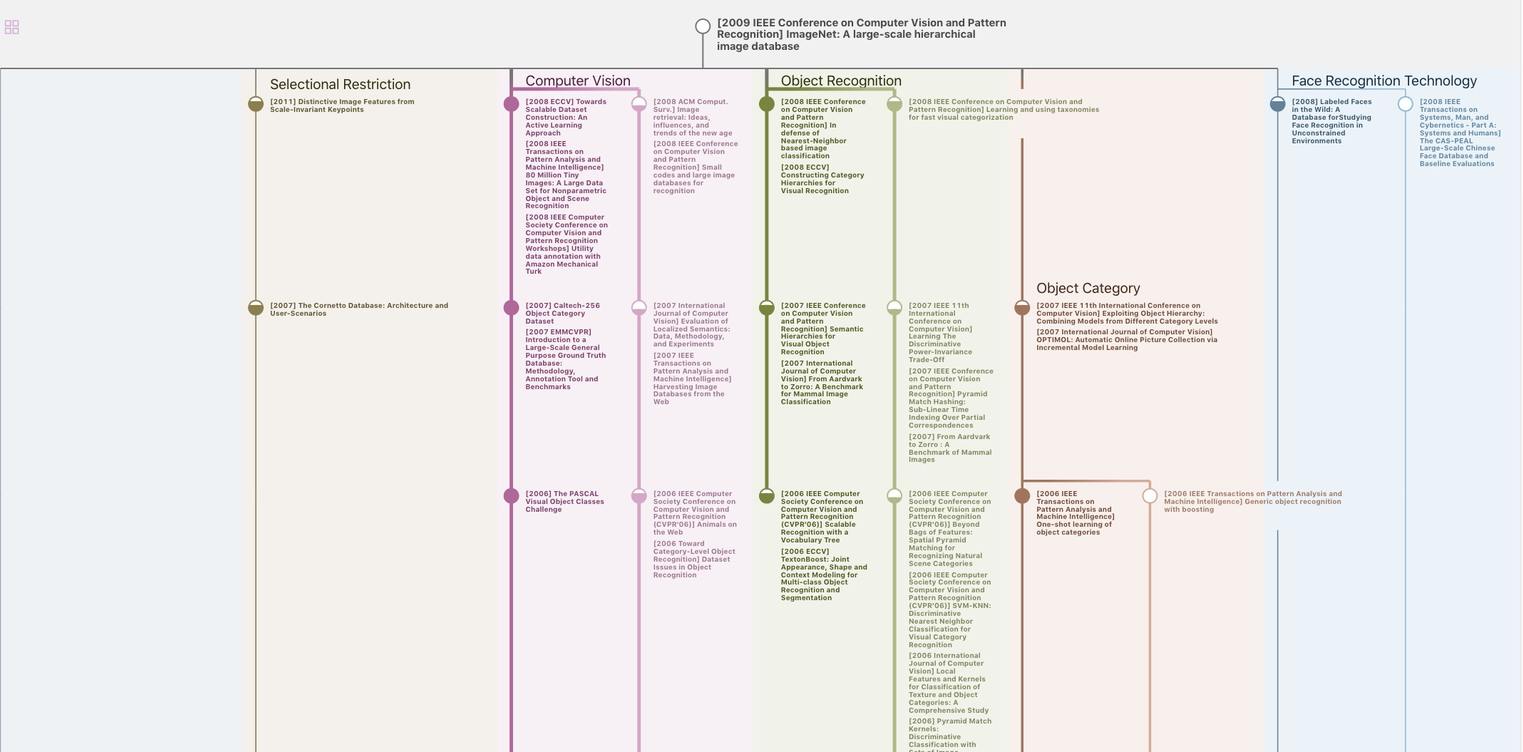
生成溯源树,研究论文发展脉络
Chat Paper
正在生成论文摘要