Pyxtal_ff: A Python Library For Automated Force Field Generation
MACHINE LEARNING-SCIENCE AND TECHNOLOGY(2021)
摘要
We present PyXtal_FF-a package based on Python programming language-for developing machine learning potentials (MLPs). The aim of PyXtal_FF is to promote the application of atomistic simulations through providing several choices of atom-centered descriptors and machine learning regressions in one platform. Based on the given choice of descriptors (including the atom-centered symmetry functions, embedded atom density, SO4 bispectrum, and smooth SO3 power spectrum), PyXtal_FF can train MLPs with either generalized linear regression or neural network models, by simultaneously minimizing the errors of energy/forces/stress tensors in comparison with the data from ab-initio simulations. The trained MLP model from PyXtal_FF is interfaced with the Atomic Simulation Environment (ASE) package, which allows different types of light-weight simulations such as geometry optimization, molecular dynamics simulation, and physical properties prediction. Finally, we will illustrate the performance of PyXtal_FF by applying it to investigate several material systems, including the bulk SiO2, high entropy alloy NbMoTaW, and elemental Pt for general purposes. Full documentation of PyXtal_FF is available at https://pyxtal-ff.readthedocs.io.
更多查看译文
关键词
machine learning potential, neural networks regression, atom-centered descriptors, atomistic simulation
AI 理解论文
溯源树
样例
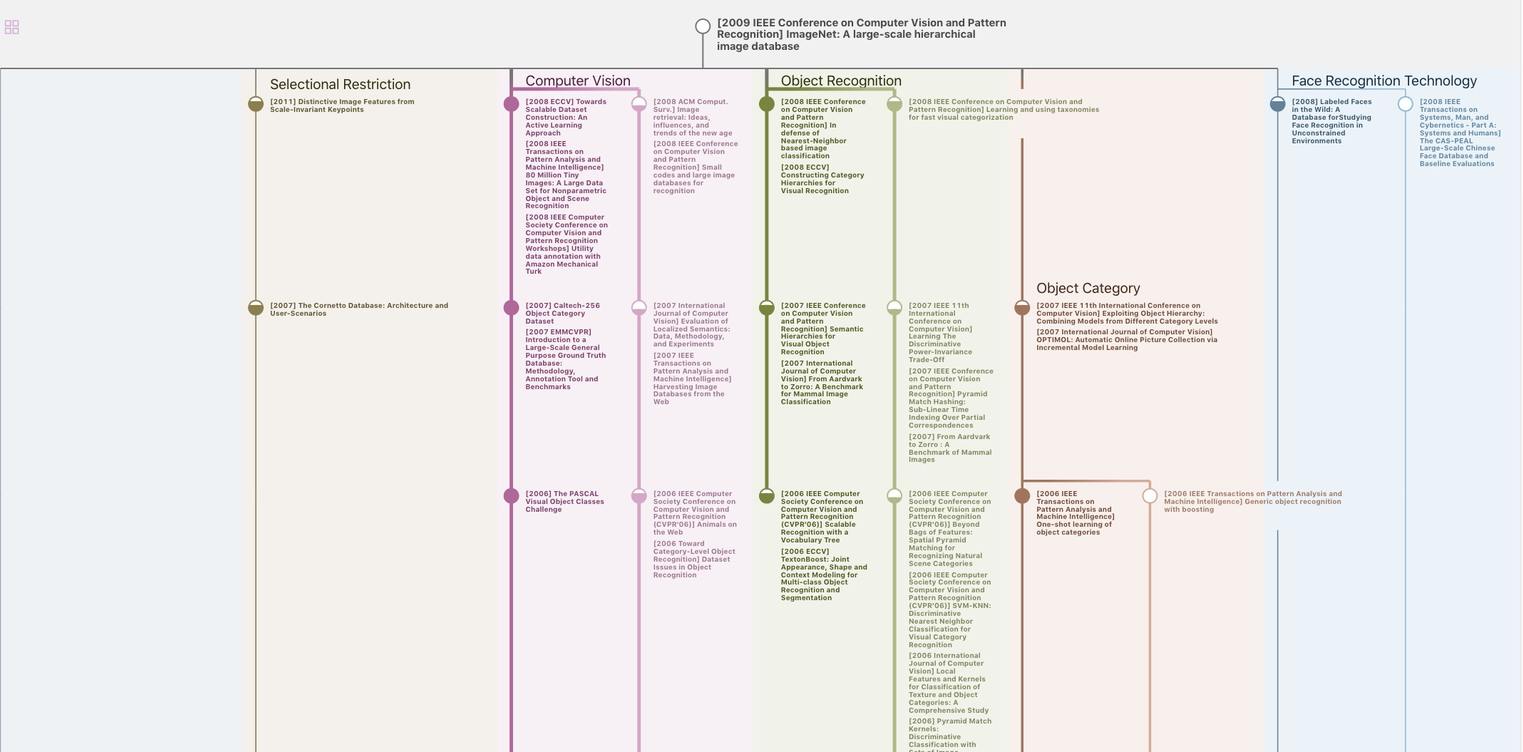
生成溯源树,研究论文发展脉络
Chat Paper
正在生成论文摘要