Online Spatio-Temporal Learning in Deep Neural Networks
arxiv(2023)
摘要
Biological neural networks are equipped with an inherent capability to continuously adapt through online learning. This aspect remains in stark contrast to learning with error backpropagation through time (BPTT) that involves offline computation of the gradients due to the need to unroll the network through time. Here, we present an alternative online learning algorithm ic framework for deep recurrent neural networks (RNNs) and spiking neural networks (SNNs), called online spatio-temporal learning (OSTL). It is based on insights from biology and proposes the clear separation of spatial and temporal gradient components. For shallow SNNs, OSTL is gradient equivalent to BPTT enabling for the first time online training of SNNs with BPTT-equivalent gradients. In addition, the proposed formulation unveils a class of SNN architectures trainable online at low time complexity. Moreover, we extend OSTL to a generic form, applicable to a wide range of network architectures, including networks comprising long short-term memory (LSTM) and gated recurrent units (GRUs). We demonstrate the operation of our algorithm ic framework on various tasks from language modeling to speech recognition and obtain results on par with the BPTT baselines.
更多查看译文
关键词
Neurons,Biology,Training,Heuristic algorithms,Approximation algorithms,Biological neural networks,Backpropagation,Backpropagation,backpropagation through time (BPTT),online learning,real-time recurrent learning (RTRL),spiking neurons
AI 理解论文
溯源树
样例
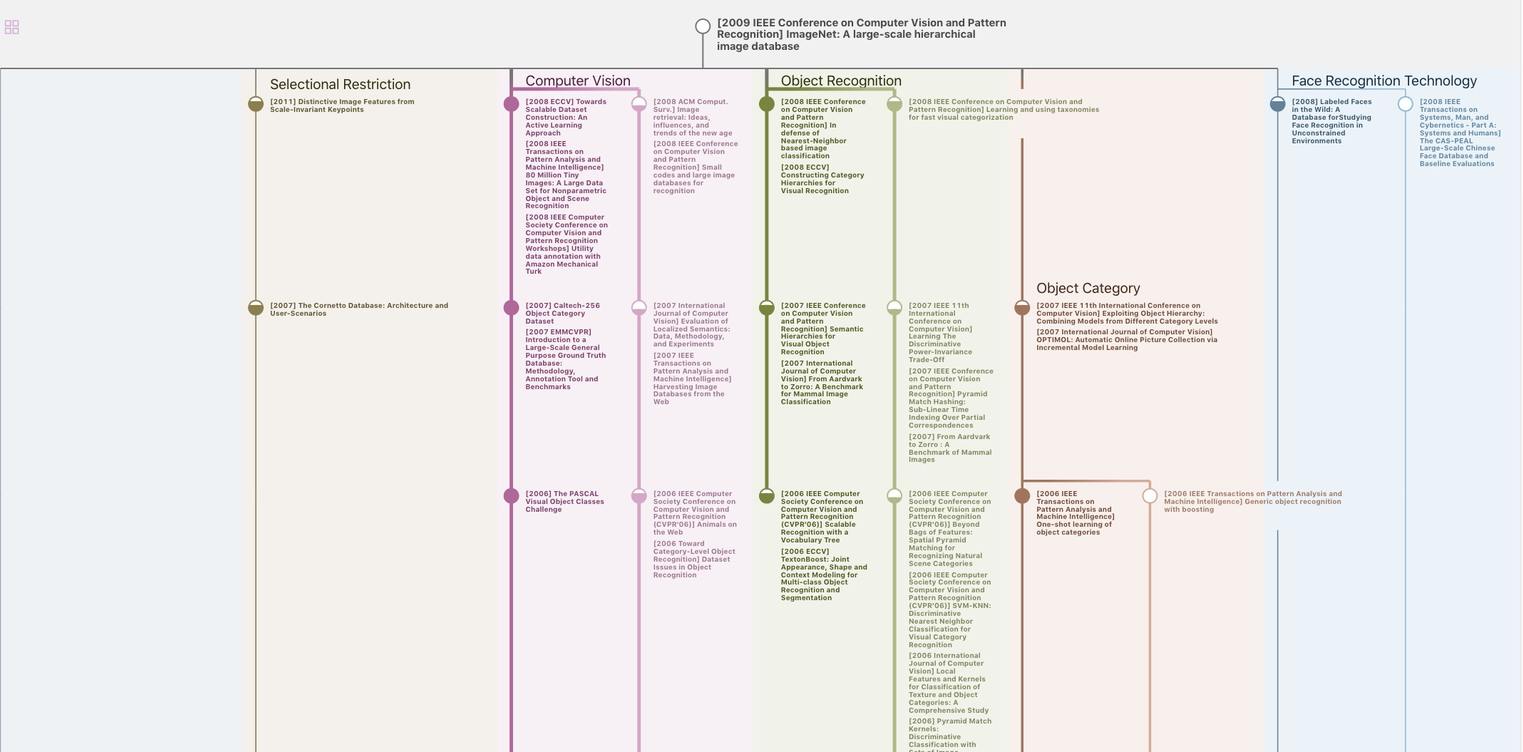
生成溯源树,研究论文发展脉络
Chat Paper
正在生成论文摘要