Machine-learned Regularization and Polygonization of Building Segmentation Masks
2020 25TH INTERNATIONAL CONFERENCE ON PATTERN RECOGNITION (ICPR)(2021)
摘要
We propose a machine learning based approach for automatic regularization and polygonization of building segmentation masks. Taking an image as input, we first predict building segmentation maps exploiting generic
fully
convolutional
network
(FCN)
. A
generative
adversarial
network
(GAN)
is then involved to perform a regularization of building boundaries to make them more realistic, i.e., having more rectilinear outlines which construct right angles if required. This is achieved through the interplay between the discriminator which gives a probability of input image being true and generator that learns from discriminator's response to create more realistic images. Finally, we train the backbone
convolutional
neural
network
(CNN)
which is adapted to predict sparse outcomes corresponding to building corners out of regularized building segmentation results. Experiments on three building segmentation datasets demonstrate that the proposed method is not only capable of obtaining accurate results, but also of producing visually pleasing building outlines parameterized as polygons.
更多查看译文
关键词
regularization,building
AI 理解论文
溯源树
样例
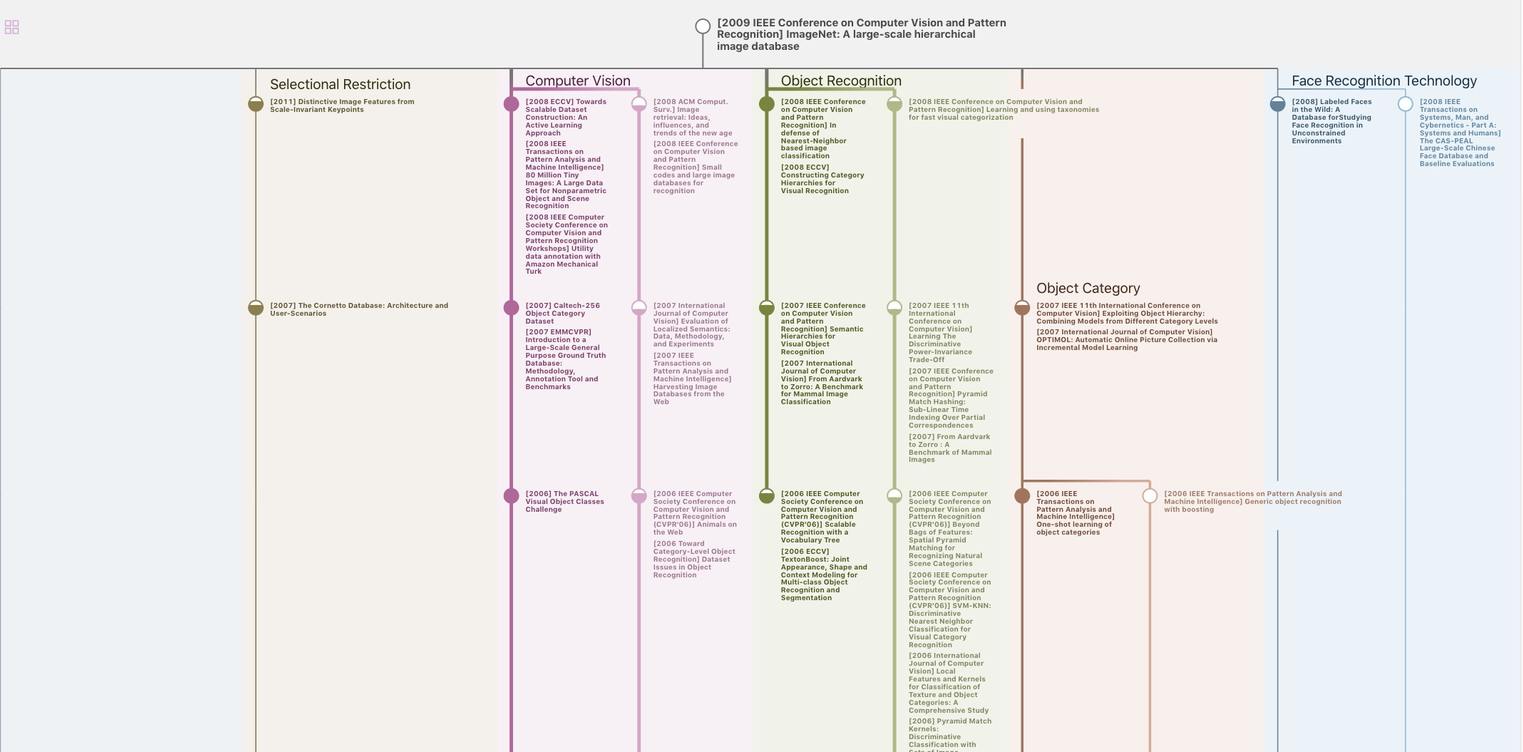
生成溯源树,研究论文发展脉络
Chat Paper
正在生成论文摘要