Fault Classification Driven By Maintenance Management For Smart Maintenance Applications
2020 IEEE INTERNATIONAL WORKSHOP ON METROLOGY FOR INDUSTRY 4.0 & IOT (METROIND4.0&IOT)(2020)
摘要
The study focuses on health monitoring applications that enables companies to offer smart maintenance services in the general context of industry 4.0. To this aim, a common practice consists in acquiring technical data, mining them using machine learning algorithms, and then deciding by taking into account commercial policies too. This work aims to demonstrate that business information can be advantageously embedded in a modified machine learning workflow. The main idea is supported by a frequent fact: practical maintenance procedures are less than the possible fault types. This assumption allows a task redefinition, thus providing an output ready for taking a final decision with better overall performances. A case study is analyzed in order to show the performance of the proposed strategy in an industrial application of condition monitoring. A combination of hypotheses and classification algorithms commonly used for predictive maintenance has been tested in order to check the performance improvement. In particular, Decision Trees, Nearest Neighbors, Linear SVM, Random Forest, AdaBoost, Naive Bayes and XGBoost have been considered. In all cases having a statistical significance, an improvement has been observed. Moreover, for the case of decision trees the advantages are gained regardless the tree heights, that is really interesting when low-performance computing platforms are actually available, as it happens for the case of IoT nodes.
更多查看译文
关键词
Smart Maintenance, Industry 4.0, Machine Learning, Feature Selection, Predictive Maintenance, Condition Monitoring, Maintenance Management, IoT
AI 理解论文
溯源树
样例
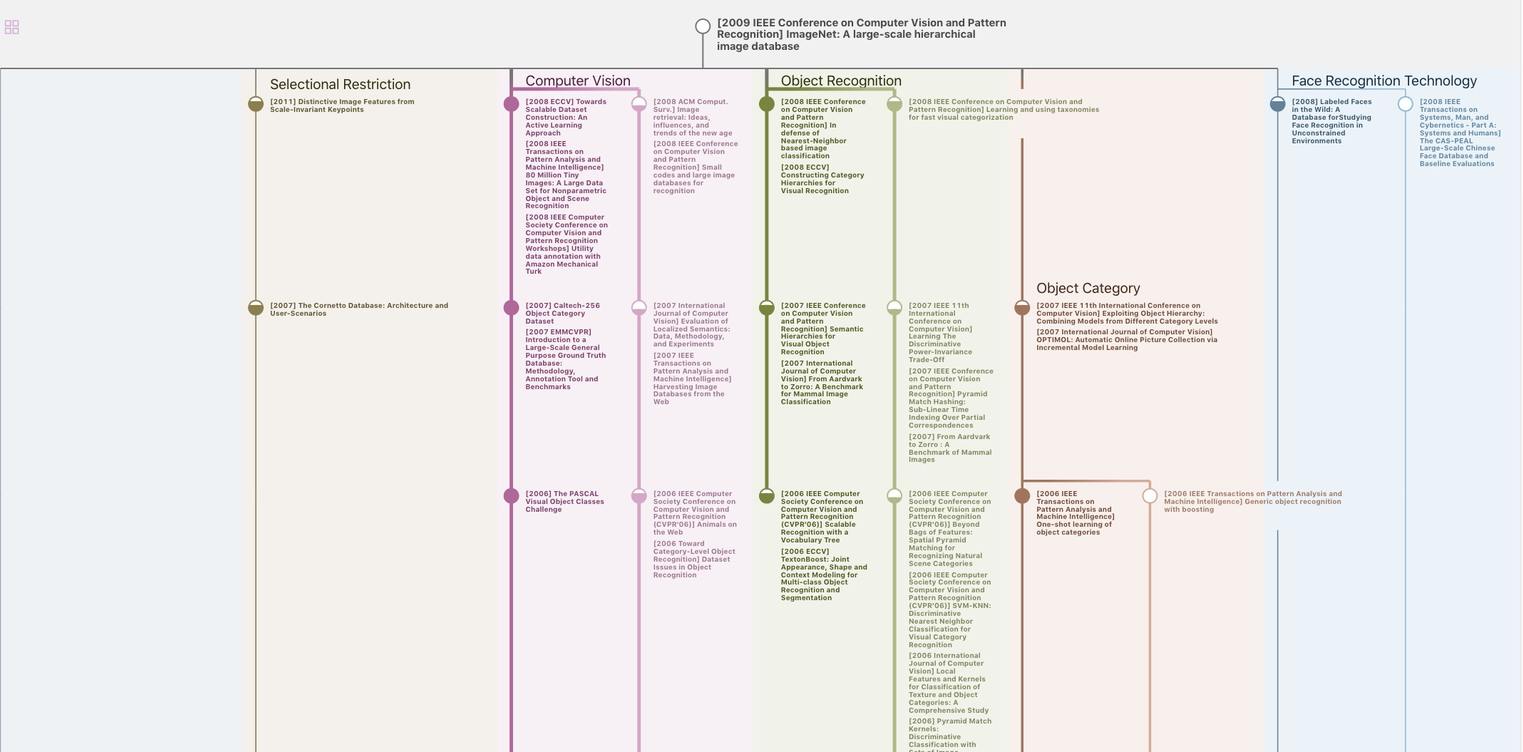
生成溯源树,研究论文发展脉络
Chat Paper
正在生成论文摘要