Extrapolated Alternating Algorithms For Approximate Canonical Polyadic Decomposition
2020 IEEE INTERNATIONAL CONFERENCE ON ACOUSTICS, SPEECH, AND SIGNAL PROCESSING(2020)
摘要
Tensor decompositions have become a central tool in machine learning to extract interpretable patterns from multiway arrays of data. However, computing the approximate Canonical Polyadic Decomposition (aCPD), one of the most important tensor decomposition model, remains a challenge. In this work, we propose several algorithms based on extrapolation that improve over existing alternating methods for aCPD. We show on several simulated and real data sets that carefully designed extrapolation can significantly improve the convergence speed hence reduce the computational time, especially in difficult scenarios.
更多查看译文
关键词
Canonical Polyadic Decomposition, Tensor, Non-convex Optimization, Block-coordinate Descent, Acceleration
AI 理解论文
溯源树
样例
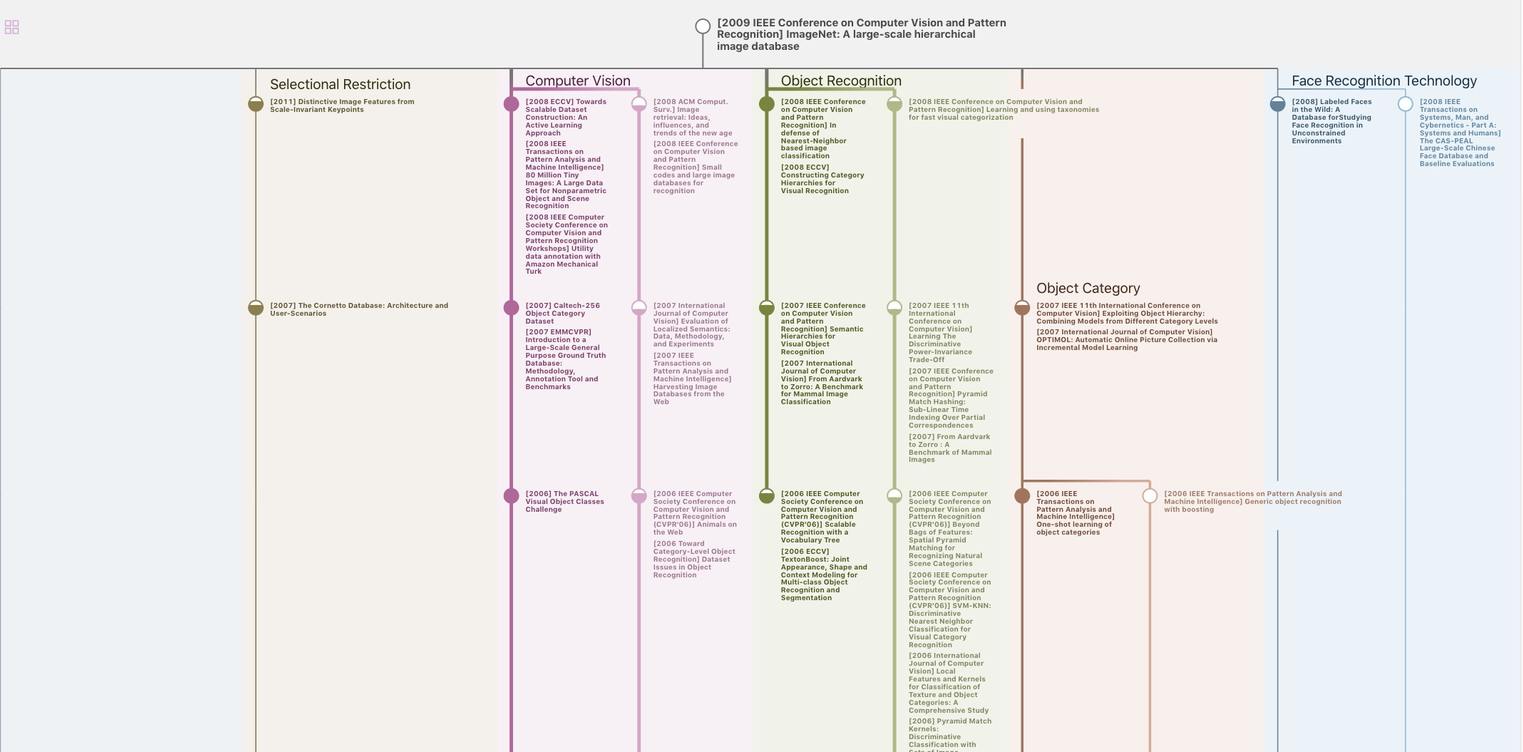
生成溯源树,研究论文发展脉络
Chat Paper
正在生成论文摘要