Reduced Sine Hyperbolic Polynomial Model For Brain Neuro- Developmental Analysis
MEDICAL IMAGING 2020: BIOMEDICAL APPLICATIONS IN MOLECULAR, STRUCTURAL, AND FUNCTIONAL IMAGING(2021)
摘要
Several studies on brain development have only considered functional connectivity (FC) of different brain regions. In the following study, we propose to add effective connectivity (EC) through Granger causality (GC) for the task of brain maturation. We do this for two different groups of subjects, i.e., children and young adults. We aim to show that the inclusion of causal interaction may further discriminate brain connections between two age groups . We extract EC feature by a new kernel-based GC (KGC) method based on a reduced Sine hyperbolic polynomial (RSP) neural network which helps to learn nonlinearity of complex brain network. Our new EC-based feature outperformed FC-based feature evaluated on Philadelphia neurocohort (PNC) study with better separation between the two different age groups. We also showed that the fusion of two sets of features (FC +EC) improved brain age prediction accuracy by more than 4%.
更多查看译文
关键词
Brain age prediction, Brain maturation, Causality, Polynomial neural network
AI 理解论文
溯源树
样例
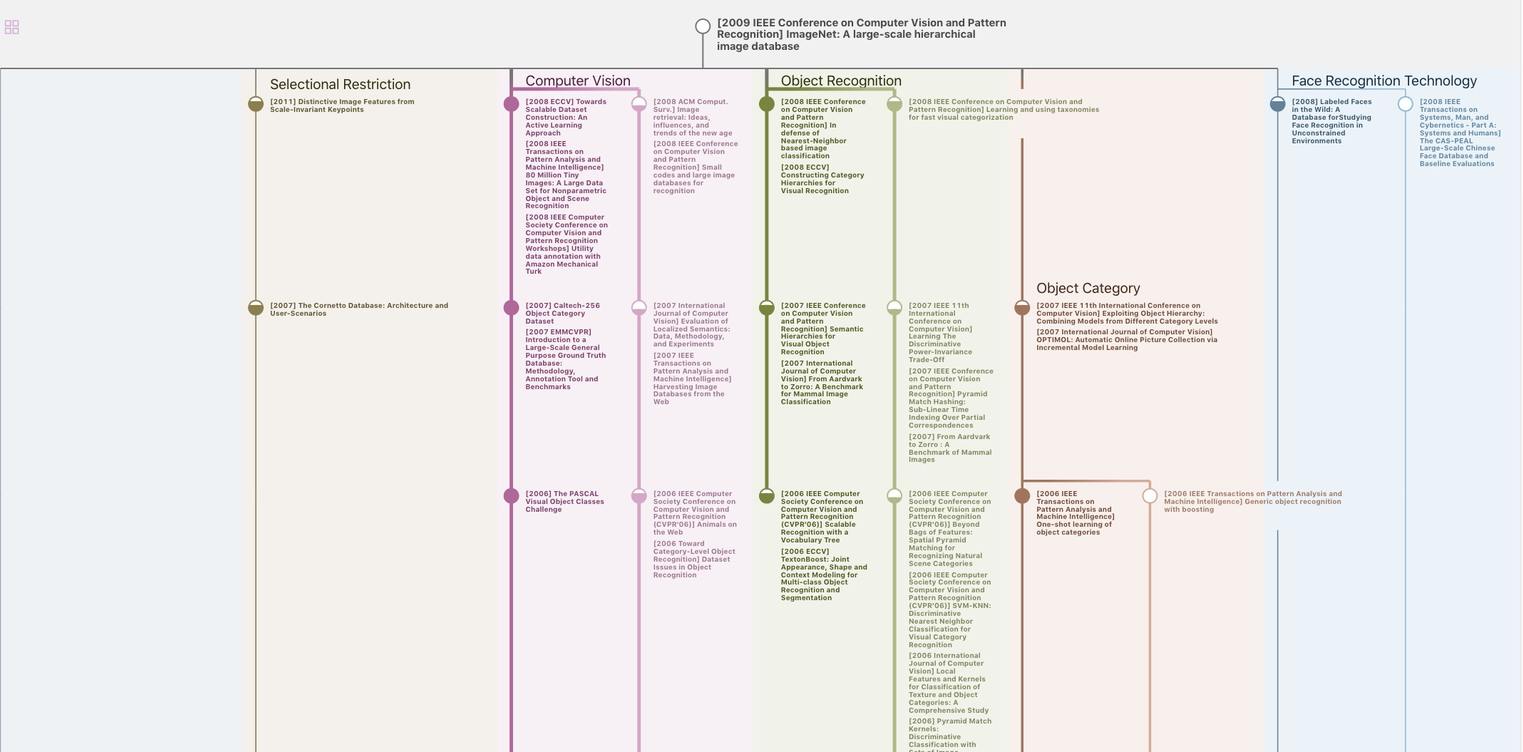
生成溯源树,研究论文发展脉络
Chat Paper
正在生成论文摘要