Scalable Inference of Symbolic Adversarial Examples
arxiv(2020)
摘要
We present a novel method for generating symbolic adversarial examples: input regions guaranteed to only contain adversarial examples for the given neural network. These regions can generate real-world adversarial examples as they summarize trillions of adversarial examples. We theoretically show that computing optimal symbolic adversarial examples is computationally expensive. We present a method for approximating optimal examples in a scalable manner. Our method first selectively uses adversarial attacks to generate a candidate region and then prunes this region with hyperplanes that fit points obtained via specialized sampling. It iterates until arriving at a symbolic adversarial example for which it can prove, via state-of-the-art convex relaxation techniques, that the region only contains adversarial examples. Our experimental results demonstrate that our method is practically effective: it only needs a few thousand attacks to infer symbolic summaries guaranteed to contain $\approx 10^{258}$ adversarial examples.
更多查看译文
关键词
symbolic adversarial examples,scalable inference
AI 理解论文
溯源树
样例
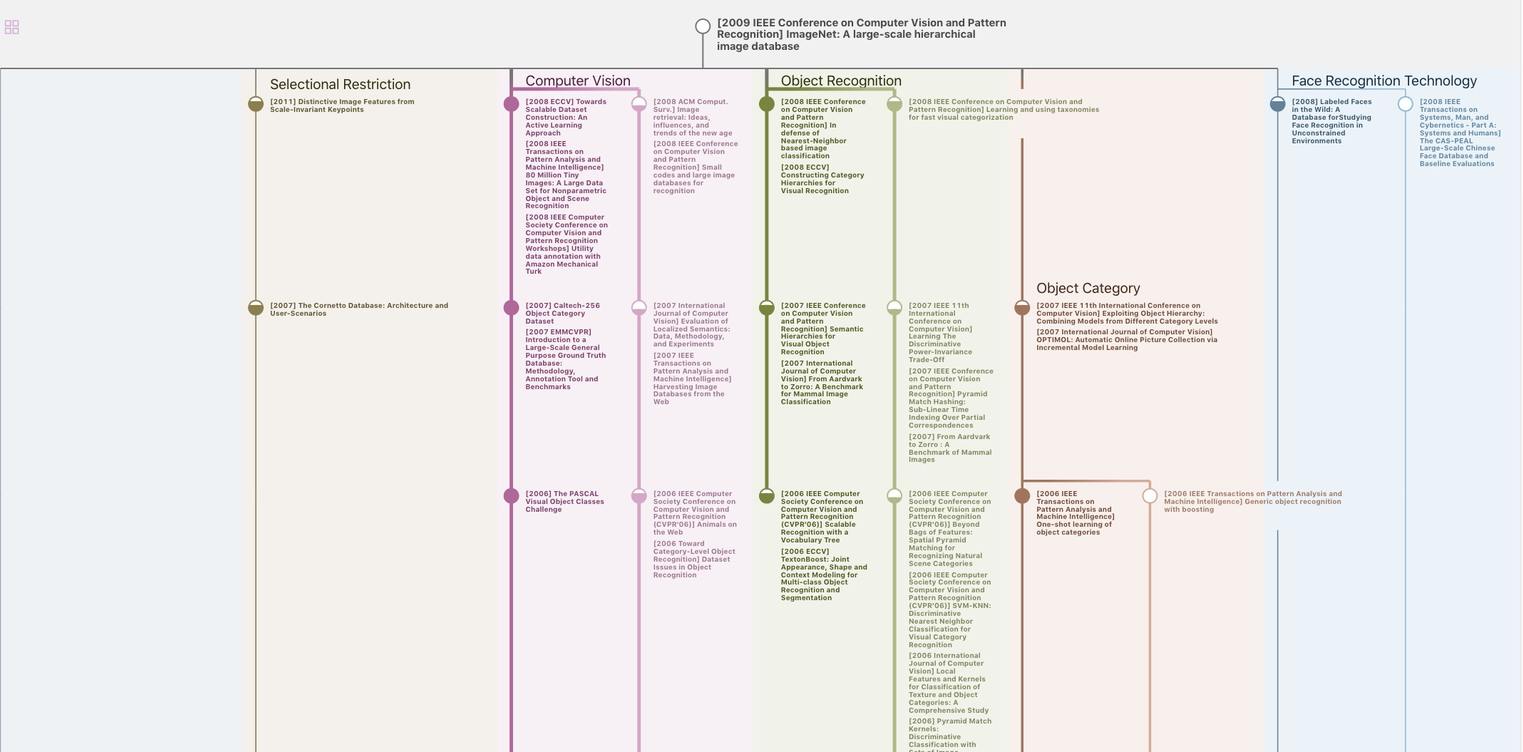
生成溯源树,研究论文发展脉络
Chat Paper
正在生成论文摘要