Using String-Comparison Measures to Improve and Evaluate Collaborative Filtering Recommender Systems.
BIAS(2020)
摘要
Recommender Systems are expert systems that utilize the user’s interests in order to recommend different products. One of the main techniques employed in this type of system is the Collaborative Filtering which recommends products to users based on their interactions and on what items similar users have liked in the past. However, many traditional methods for determining similarity do not consider temporal information neither the rich information that is contained in sequentially-ordered user interactions. Therefore, the present work proposes the usage of traditional string comparison approaches for defining new similarity measures between pairs of users that take into consideration time-ordered data. The general idea is to model the similarity computation between users as an approximate string matching problem and to employ classical algorithms that solve it. Experiments conducted in different application domains demonstrate that the measures based on a string-comparison approach can improve accuracy. Furthermore, they represent a new way of evaluating the performance of sequence-based recommendation.
更多查看译文
关键词
string-comparison
AI 理解论文
溯源树
样例
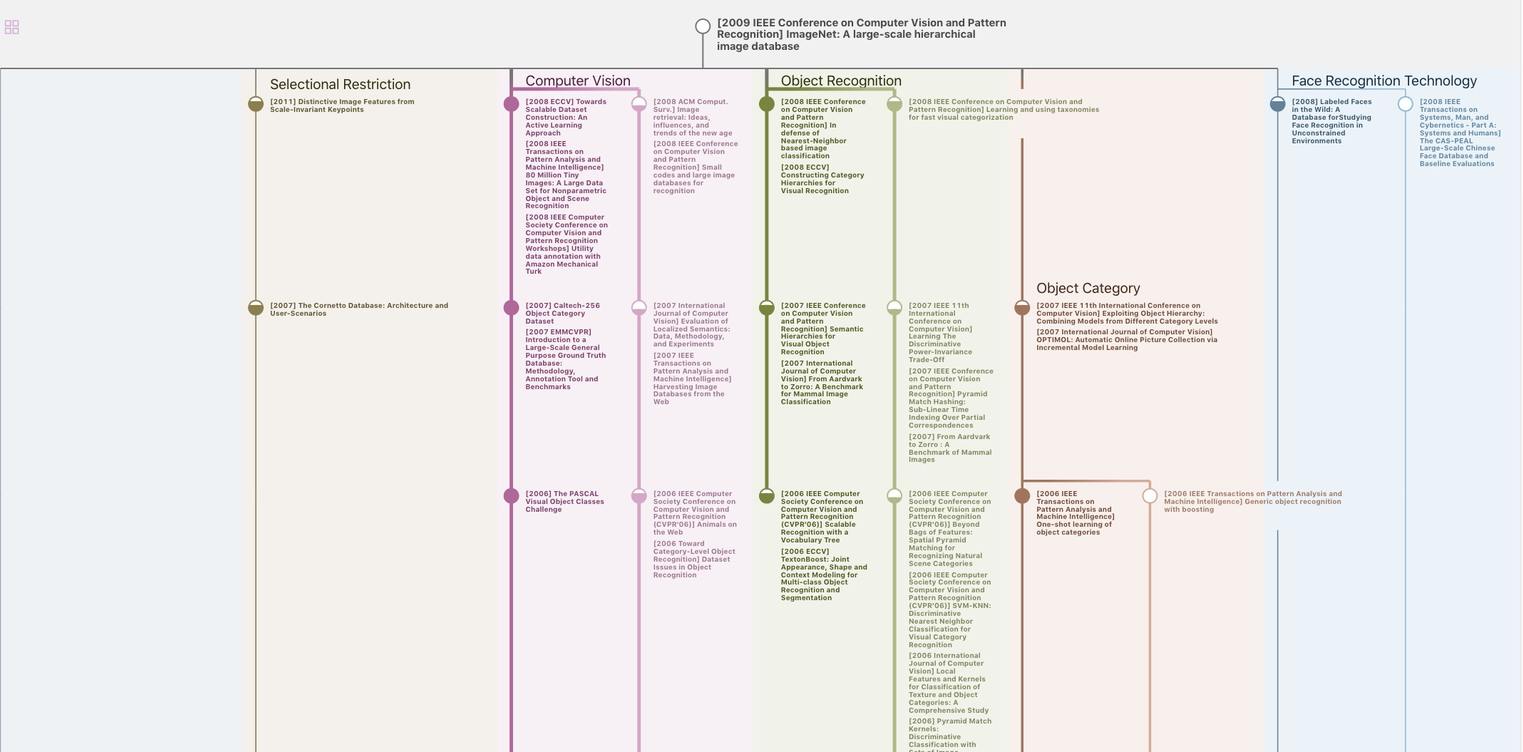
生成溯源树,研究论文发展脉络
Chat Paper
正在生成论文摘要