Variational Auto-encoders application in wireless Vehicle-to-Everything communications.
VTC Spring(2020)
摘要
In this paper, a new technique called Embedded Variational Auto Encoder (EVAE) is proposed for Vehicle-to-Everything (V2X) up-link scenario. An innovative method has been proposed for the accurate prediction of the interference at the receiving end of each user which leads to the enhancement of the end-to-end performance of the V2X. The new algorithm infers noise and fading probabilistic models effect using decentralized Probabilistic Neural Networks (PNNs), while a second centralized PNN has been embedded inside the first group of the PNNs. This single PNN will be used to infer the interference effect on each V2X receiver. The performance of EVAE is compared with the recently proposed neural networks (NN) algorithms based on conventional auto-encoders (AE). Numerical and simulation results for the achievable symbol error rates (SER) have shown a significant improvement particularly in the high SINR regime, compared with the classical systems based on maximum likeli-hood detection.
更多查看译文
关键词
Deep Learning,Variational Autoencoder,V2X,Interference prediction,Artificial Intelligence,Machine Learning,Wireless Communications
AI 理解论文
溯源树
样例
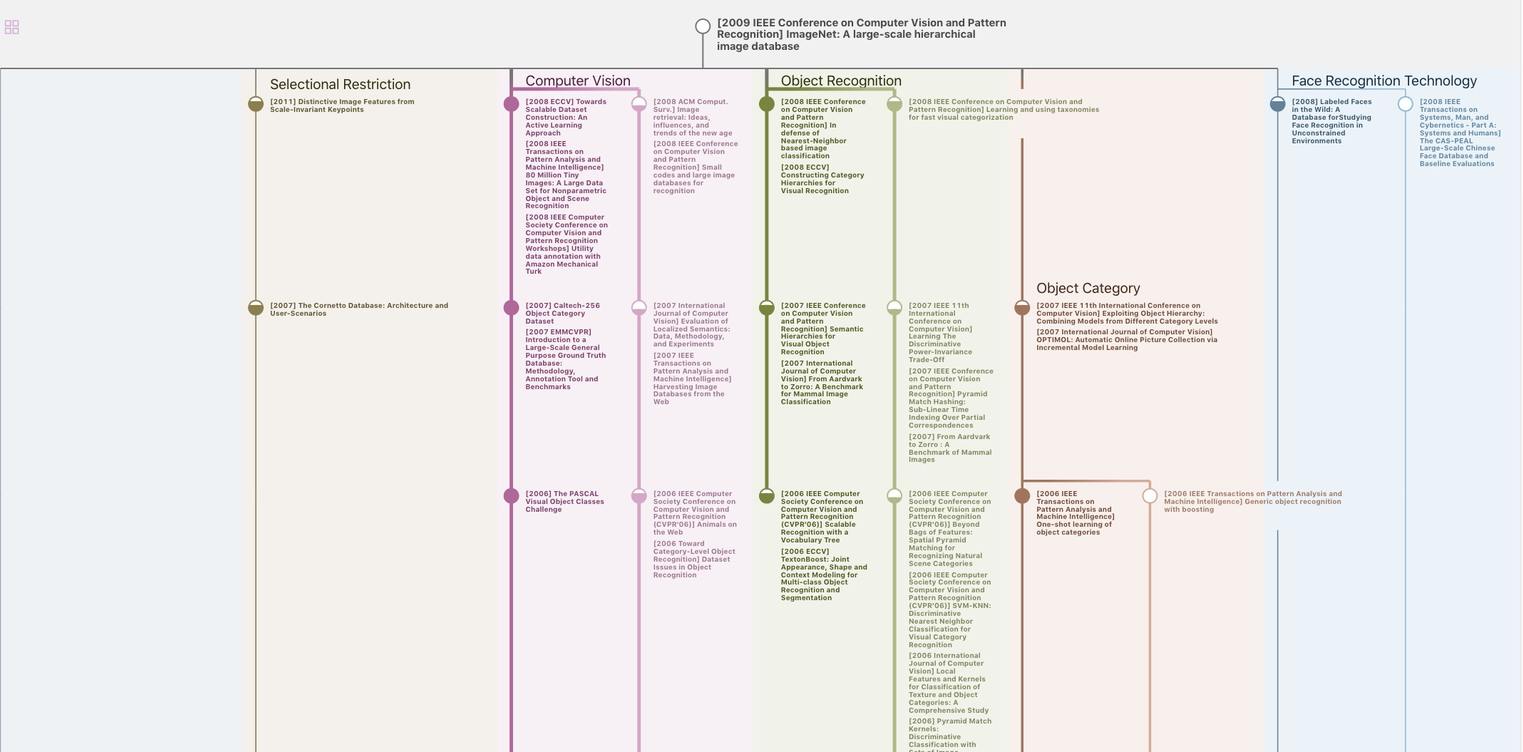
生成溯源树,研究论文发展脉络
Chat Paper
正在生成论文摘要