Neural-Enhanced Live Streaming: Improving Live Video Ingest via Online Learning
SIGCOMM '20: Annual conference of the ACM Special Interest Group on Data Communication on the applications, technologies, architectures, and protocols for computer communication Virtual Event USA August, 2020(2020)
摘要
Live video accounts for a significant volume of today's Internet video. Despite a large number of efforts to enhance user quality of experience (QoE) both at the ingest and distribution side of live video, the fundamental limitations are that streamer's upstream bandwidth and computational capacity limit the quality of experience of thousands of viewers.
To overcome this limitation, we design LiveNAS, a new live video ingest framework that enhances the origin stream's quality by leveraging computation at ingest servers. Our ingest server applies neural super-resolution on the original stream, while imposing minimal overhead on ingest clients. LiveNAS employs online learning to maximize the quality gain and dynamically adjusts the resource use to the real-time quality improvement. LiveNAS delivers high-quality live streams up to 4K resolution, outperforming WebRTC by 1.96 dB on average in Peak-Signal-to-Noise-Ratio on real video streams and network traces, which leads to 12%-69% QoE improvement for live stream viewers.
更多查看译文
关键词
video delivery, live streaming, super-resolution, deep neural networks, online learning
AI 理解论文
溯源树
样例
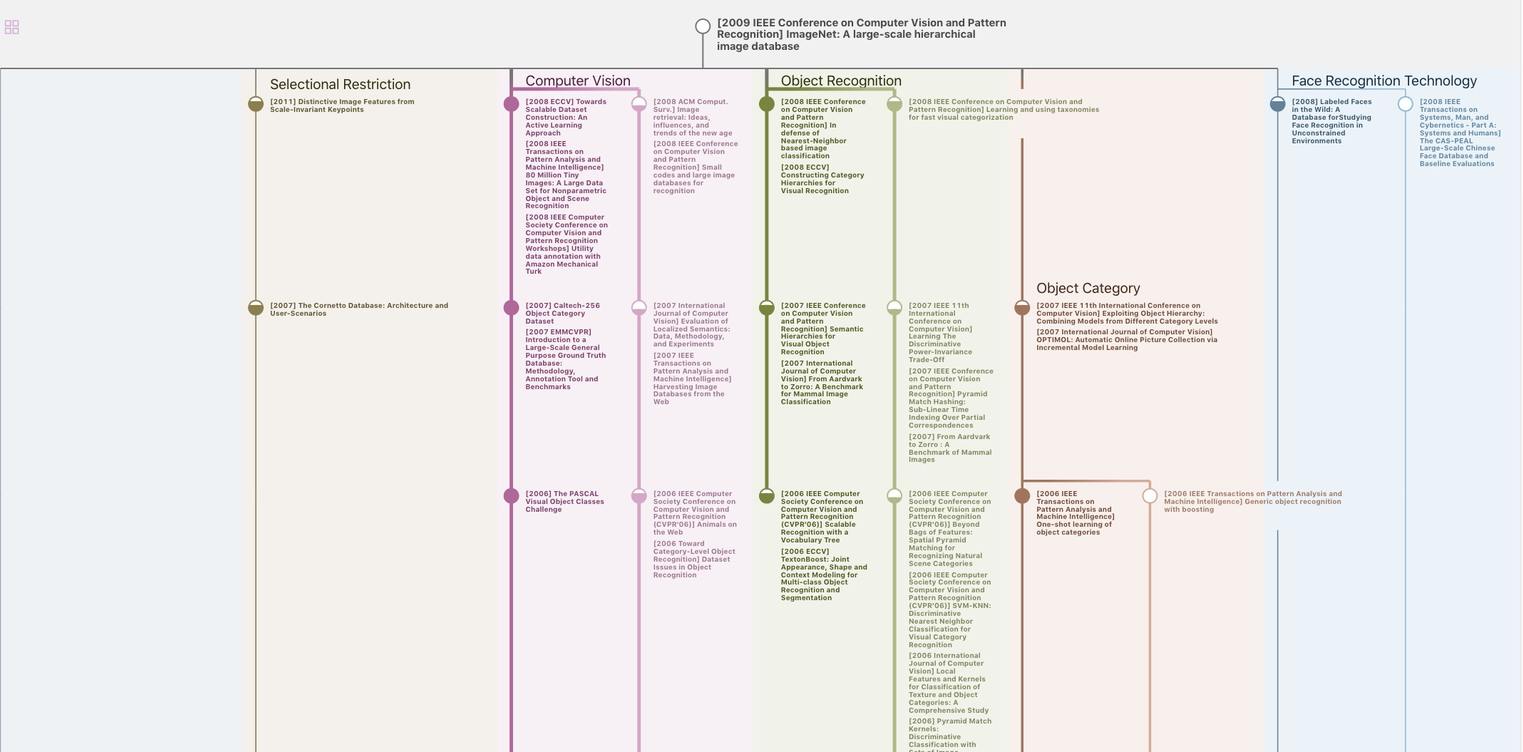
生成溯源树,研究论文发展脉络
Chat Paper
正在生成论文摘要