Supervised learning on heterogeneous, attributed entities interacting over time
arxiv(2020)
摘要
Most physical or social phenomena can be represented by ontologies where the constituent entities are interacting in various ways with each other and with their environment. Furthermore, those entities are likely heterogeneous and attributed with features that evolve dynamically in time as a response to their successive interactions. In order to apply machine learning on such entities, e.g., for classification purposes, one therefore needs to integrate the interactions into the feature engineering in a systematic way. This proposal shows how, to this end, the current state of graph machine learning remains inadequate and needs to be be augmented with a comprehensive feature engineering paradigm in space and time.
更多查看译文
关键词
entities,learning
AI 理解论文
溯源树
样例
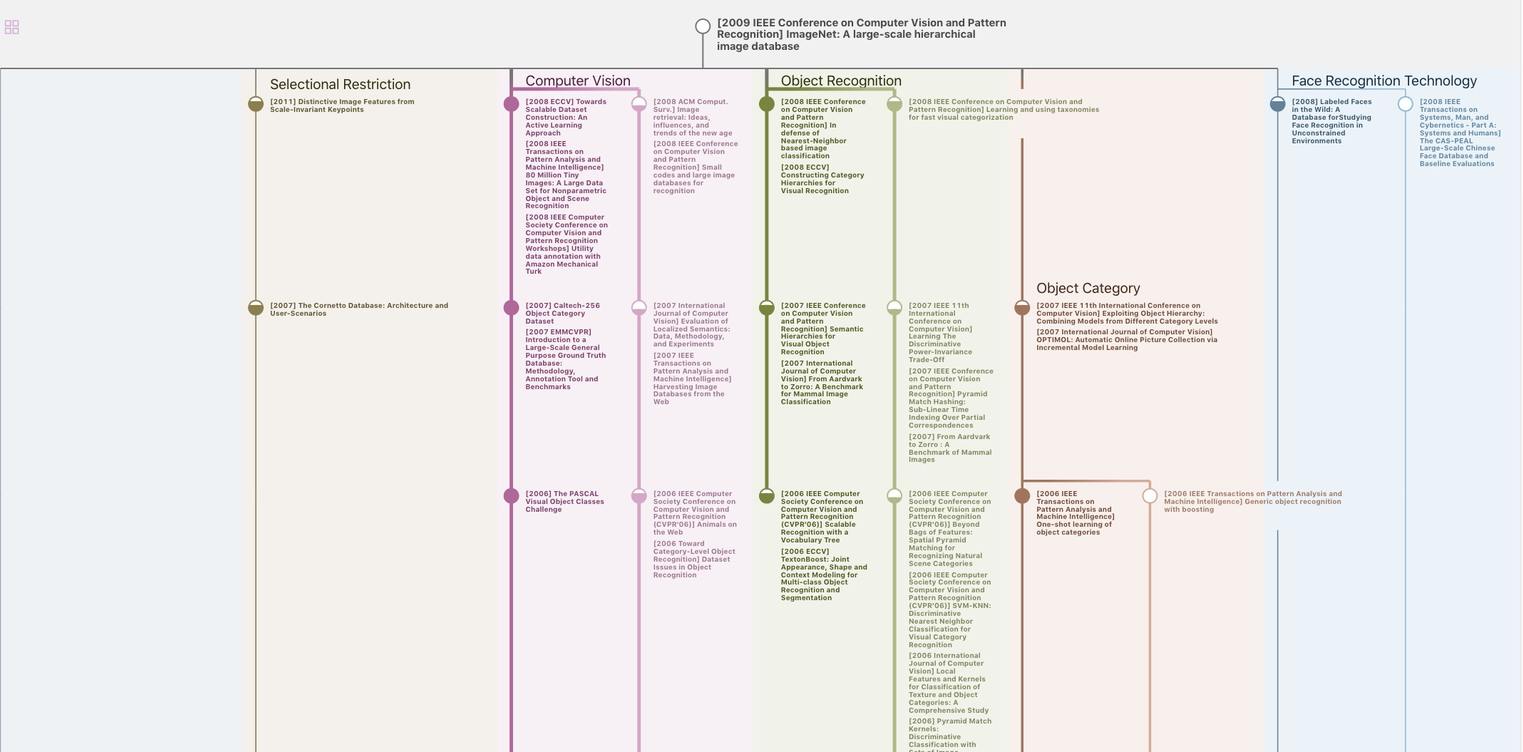
生成溯源树,研究论文发展脉络
Chat Paper
正在生成论文摘要